Facial Recognition of Sketch Images in Forensic Laboratories Employing Diverse Techniques
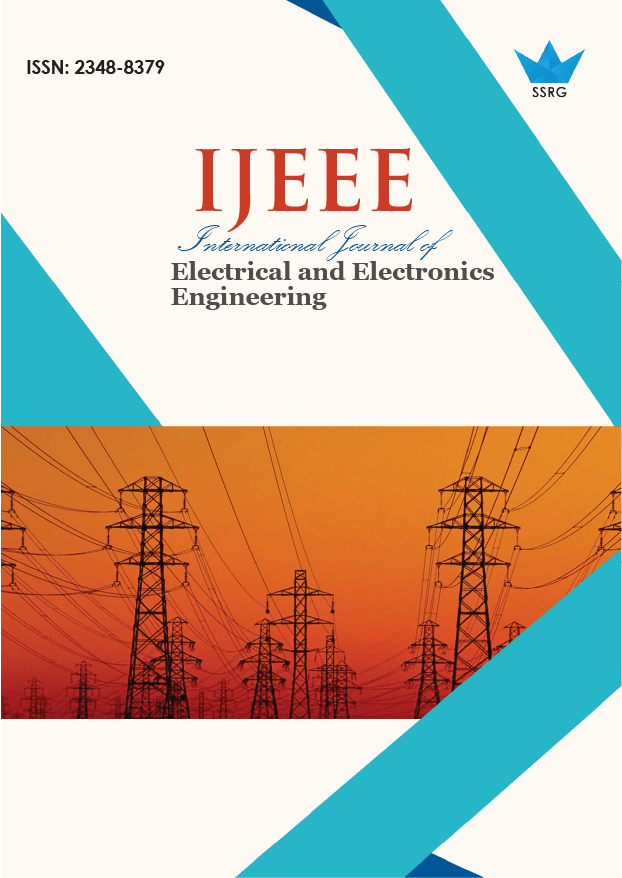
International Journal of Electrical and Electronics Engineering |
© 2024 by SSRG - IJEEE Journal |
Volume 11 Issue 5 |
Year of Publication : 2024 |
Authors : Devendra A. Itole, M.P. Sardey, Milind P. Gajare |
How to Cite?
Devendra A. Itole, M.P. Sardey, Milind P. Gajare, "Facial Recognition of Sketch Images in Forensic Laboratories Employing Diverse Techniques," SSRG International Journal of Electrical and Electronics Engineering, vol. 11, no. 5, pp. 1-11, 2024. Crossref, https://doi.org/10.14445/23488379/IJEEE-V11I5P101
Abstract:
The use of Differential Facial Recognition (DFR) over the past few years emerged as a challenging endeavor within the realms of biometrics and computer vision, grappling persistently with the complexities of illumination and pose variations. This scholarly investigation aims to propose innovative deep-learning architectures tailored to juxtapose non-visible facial depictions against an array of visible facial galleries. The taxonomy of thermal-to-visible recognition delineates into two distinct categories: feature-based methodologies and image synthesis paradigms. Notably, the latter enhances compatibility with existing recognition frameworks in both commercial and governmental sectors, bolstering efficacy in forensic examination. Additionally, the incorporation of soft biometrics, encompassing diverse traits such as age and gender, provides supplementary layers of information, thereby reinforcing the foundation of recognition algorithms. Novel strategies are introduced to navigate the intricate landscape of auxiliary training data in the LUPI scenario, pushing the boundaries of recognition performance. Additionally, a pioneering aggregation framework is conceived to enhance the robustness of landmark detection, while adversarial techniques amplify the efficacy of landmark detection mechanisms. Finally, this study scrutinises the opaque veil enveloping Generative Adversarial Networks (GANs), aiming to address concerns regarding mode collapse and diversity within the GAN framework.
Keywords:
Heterogeneous Face Recognition (HFR), Deep Learning Architectures, Thermal-to-Visible Recognition, Soft Biometrics, Generative Adversarial Networks (GANs).
References:
[1] Seyed Mehdi Iranmanesh, Ali Dabouei, and Nasser M. Nasrabadi, “Attribute Adaptive Margin Softmax Loss Using Privileged Information,” arXiv, 2009.
[CrossRef] [Google Scholar] [Publisher Link]
[2] Seyed Mehdi Iranmanesh et al., “Deep Cross Polarimetric Thermal-to-Visible Face Recognition,” 2018 International Conference on Biometrics (ICB), Australia, pp. 166-173, 2018.
[CrossRef] [Google Scholar] [Publisher Link]
[3] Yasin Yazici, Kim-Hui Yap, and Stefan Winkler, “Autoregressive Generative Adversarial Networks,” International Conference on Learning Representations, 2018.
[Google Scholar] [Publisher Link]
[4] He Zhang, and Vishal M. Patel, “Density-Aware Single Image De-Raining Using a Multi-Stream Dense Network,” IEEE Conference on Computer Vision and Pattern Recognition, pp. 695-704, 2018.
[Google Scholar] [Publisher Link]
[5] Ali Dabouei et al., “Fingerprint Distortion Rectification Using Deep Convolutional Neural Networks,” 2018 International Conference on Biometrics, Australia, pp. 1-8, 2018.
[CrossRef] [Google Scholar] [Publisher Link]
[6] Sobhan Soleymani et al., “Generalised Bilinear Deep Convolutional Neural Networks for Multimodal Biometric Identification,” 2018 25th IEEE International Conference on Image Processing (ICIP), Greece, pp. 763-767, 2018.
[CrossRef] [Google Scholar] [Publisher Link]
[7] Sebastian Nowozin, Botond Cseke, and Ryota Tomioka, “f-GAN: Training Generative Neural Samplers Using Variational Divergence Minimisation,” Advances in Neural Information Processing Systems, 2016.
[Google Scholar] [Publisher Link]
[8] He Zhang, and Vishal M. Patel, “Densely Connected Pyramid Dehazing Network,” IEEE Conference on Computer Vision and Pattern Recognition (CVPR), pp. 3194-3203, 2018.
[Google Scholar] [Publisher Link]
[9] Jia Deng et al., “Imagenet: A Large-Scale Hierarchical Image Database,” 2009 IEEE Conference on Computer Vision and Pattern Recognition, USA, pp. 248-255, 2009.
[CrossRef] [Google Scholar] [Publisher Link]
[10] Hengshuang Zhao et al., “Pyramid Scene Parsing Network,” IEEE Conference on Computer Vision and Pattern Recognition (CVPR), pp. 2881-2890, 2017.
[Google Scholar] [Publisher Link]
[11] Simon Jegou et al., “The One Hundred Layers Tiramisu: Fully Convolutional Densenets for Semantic Segmentation,” IEEE Conference on Computer Vision and Pattern Recognition (CVPR) Workshops, pp. 11-19, 2017.
[Google Scholar] [Publisher Link]
[12] Saeid Motiian et al., “Information Bottleneck Learning Using Privileged Information for Visual Recognition,” IEEE Conference on Computer Vision and Pattern Recognition (CVPR), pp. 1496-1505, 2016.
[Google Scholar] [Publisher Link]
[13] Amirsina Torfi et al., “3D Convolutional Neural Networks for Cross Audio-Visual Matching Recognition,” IEEE Access, vol. 5, pp. 22081-22091, 2017.
[CrossRef] [Google Scholar] [Publisher Link]
[14] S. Seyed Mehdi Iranmanesh et al., “Coupled Generative Adversarial Network for Heterogeneous Face Recognition,” Image and Vision Computing, vol. 94, 2020. [CrossRef] [Google Scholar] [Publisher Link]
[15] Justin Johnson, Alexandre Alahi, and Li Fei-Fei, “Perceptual Losses for Real-Time Style Transfer and Superresolution,” European Conference on Computer Vision, pp. 694–711, 2016.
[CrossRef] [Google Scholar] [Publisher Link]
[16] N. Dalal, and B. Triggs, “Histograms of Oriented Gradients for Human Detection,” 2005 IEEE Computer Society Conference on Computer Vision and Pattern Recognition (CVPR'05), USA, vol. 1, pp. 886-893, 2005.
[CrossRef] [Google Scholar] [Publisher Link]
[17] Sobhan Soleymani et al., “Multi-Level Feature Abstraction from Convolutional Neural Networks for Multimodal Biometric Identification,” 2018 24th International Conference on Pattern Recognition (ICPR), China, pp. 3469-3476, 2018.
[CrossRef] [Google Scholar] [Publisher Link]
[18] Gao Huang et al., “Densely Connected Convolutional Networks,” IEEE Conference on Computer Vision and Pattern Recognition (CVPR), pp. 4700-4708, 2017.
[Google Scholar] [Publisher Link]
[19] David Warde-Farley, and Yoshua Bengio, “Improving Generative Adversarial Networks with Denoising Feature Matching,” 2017 International Conference on Learning Representations, 2022.
[Google Scholar] [Publisher Link]
[20] Scott E. Reed et al., “Deep Visual Analogy-Making,” Advances in Neural Information Processing Systems, 2015.
[Google Scholar] [Publisher Link]
[21] Kaiming He et al., “Mask R-CNN,” IEEE International Conference on Computer Vision (ICCV), pp. 2961-2969, 2017.
[Google Scholar] [Publisher Link]
[22] Lucas Theis, Aäron Van Den Oord, and Matthias Bethge, “A Note on the Evaluation of Generative Models,” arXiv, 2015.
[CrossRef] [Google Scholar] [Publisher Link]
[23] Stan Z. Li et al., “The Casia NIR-VIS 2.0 Face Database,” IEEE Conference on Computer Vision and Pattern Recognition (CVPR) Workshops, pp. 348-353, 2013.
[Google Scholar] [Publisher Link]
[24] Brendan Klare, Zhifeng Li, and Anil K. Jain, “Matching Forensic Sketches to Mug Shot Photos,” IEEE Transactions on Pattern Analysis and Machine Intelligence, vol. 33, no. 3, pp. 639-646, 2011.
[CrossRef] [Google Scholar] [Publisher Link]