Dynamic Seizure Recognition: Invelling Epileptic Patterns with CNN-LSTM Networks
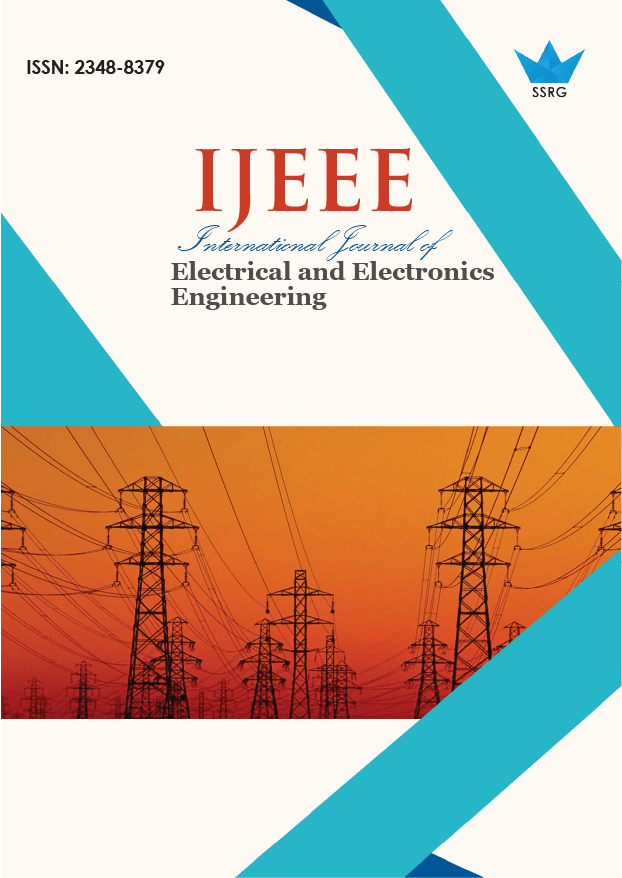
International Journal of Electrical and Electronics Engineering |
© 2024 by SSRG - IJEEE Journal |
Volume 11 Issue 5 |
Year of Publication : 2024 |
Authors : Kishori Shekokar, Shweta Dour |
How to Cite?
Kishori Shekokar, Shweta Dour, "Dynamic Seizure Recognition: Invelling Epileptic Patterns with CNN-LSTM Networks," SSRG International Journal of Electrical and Electronics Engineering, vol. 11, no. 5, pp. 199-211, 2024. Crossref, https://doi.org/10.14445/23488379/IJEEE-V11I5P118
Abstract:
Epilepsy is a condition affecting the nervous system. which is diagnosed with the aid of Electroencephalography (EEG)through a neurologist or experts. Frequent and unpredictable seizures characterise it may cause loss of consciousness, altered awareness, or unusual sensations. Reducing the use of conventional diagnostic methods is crucial, as is making the diagnosis of this condition early on, before behavioural. Signs appear. This project aims to introduce an intelligent framework for diagnosing neurological disorders (epilepsy) based on EEG recordings utilising the techniques of deep learning. In EEG signals the epileptic seizures are recognised with the help of sharp spikes of the signals. The focus lies on developing a system to transform the subjective qualitative diagnostic criteria into a more objective quantitative prognosis criterion and to analyse hidden dynamics of the Neurological data for extracting more information about the pathological versus normal status of the signals. 1D-Convolutional Neural Network and a long short-term memory hybrid model of deep learning have been employed to identify epileptic seizures. In this study, the authors used a CNN-LSTM network with 20 epochs on two separate datasets to get an optimal detection rate of EEG data exhibiting seizures compared to those without. By adding noise to the EEG signals the suggested model’s adaptability has been examined. Neurologists will find the suggested methods useful for detecting seizures in real-time.
Keywords:
Classification, Convolutional Neural Network, Epileptic seizures, E-health, Electroencephalography, Long ShortTerm Memory, Monitoring, Neurological disorder, Sensors.
References:
[1] Rajesh P.N. Rao, Brain-Computer Interfacing: An Introduction, Cambridge University Press, 2013.
[CrossRef] [Google Scholar] [Publisher Link]
[2] Siuly Siuly, and Yanchun Zhang, “Medical Big Data: Neurological Diseases Diagnosis through Medical Data Analysis,” Data Science and Engineering, vol. 1, pp. 54-64, 2016.
[CrossRef] [Google Scholar] [Publisher Link]
[3] David Borsook, “Neurological Diseases and Pain,” Brain, vol. 135, no. 2, pp. 320-344, 2012.
[CrossRef] [Google Scholar] [Publisher Link]
[4] Hans Berger, “About the Human Electroencephalogram,” Archives of Psychiatry and Nervous Diseases, vol. 87, pp. 527-570, 1929.
[CrossRef] [Google Scholar] [Publisher Link]
[5] Anubha Gupta, Pushpendra Singh, and Mandar Karlekar, “A Novel Signal Modeling Approach for Classification of Seizure and SeizureFree EEG Signals,” IEEE Transactions on Neural Systems and Rehabilitation Engineering, vol. 26, no. 5, pp. 925-935, 2018.
[CrossRef] [Google Scholar] [Publisher Link]
[6] Gaurav Dhiman et al., “BEPO: A Novel Binary Emperor Penguin Optimiser for Automatic Feature Selection,” Knowledge-Based Systems, vol. 211, 2021.
[CrossRef] [Google Scholar] [Publisher Link]
[7] Kuniaki Noda et al., “Audio-Visual Speech Recognition Using Deep Learning,” Applied Intelligence, vol. 42, pp. 722-737, 2015.
[CrossRef] [Google Scholar] [Publisher Link]
[8] Arpana Mahajan, and Sanjay Chaudhary, “Categorical Image Classification Based on Representational Deep Network (RESNET),” 2019 3rd International conference on Electronics, Communication and Aerospace Technology (ICECA), Coimbatore, India, pp. 327-330, 2019.
[CrossRef] [Google Scholar] [Publisher Link]
[9] Daniele Ravì et al., “Deep Learning for Health Informatics,” IEEE Journal of Biomedical and Health Informatics, vol. 21, no. 1, pp. 421, 2017.
[CrossRef] [Google Scholar] [Publisher Link]
[10] Surbhi Gupta, Mustafa Sameer, and Neeraj Mohan, “Detection of Epileptic Seizures Using Convolutional Neural Network,” 2021 International Conference on Emerging Smart Computing and Informatics (ESCI), Pune, India, pp. 786-790, 2021.
[CrossRef] [Google Scholar] [Publisher Link]
[11] Arpana Mahajan, Kavitha Somaraj, and Mustafa Sameer, “Adopting Artificial Intelligence Powered ConvNet to Detect Epileptic Seizures,” 2020 IEEE-EMBS Conference on Biomedical Engineering and Sciences (IECBES), Langkawi Island, Malaysia, pp. 427-432, 2021.
[CrossRef] [Google Scholar] [Publisher Link]
[12] U. Rajendra Acharya et al., “Deep Convolutional Neural Network for the Automated Detection and Diagnosis of Seizure Using EEG Signals,” Computers in Biology and Medicine, vol. 100, pp. 270-278, 2018.
[CrossRef] [Google Scholar] [Publisher Link]
[13] Ihsan Ullah et al., “An Automated System for Epilepsy Detection Using EEG Brain Signals Based on Deep Learning Approach,” Expert Systems with Applications, vol. 107, pp. 61-71, 2018.
[CrossRef] [Google Scholar] [Publisher Link]
[14] S. Raghu et al., “EEG Based Multi-Class Seizure Type Classification Using Convolutional Neural Network and Transfer Learning,” Neural Networks, vol. 124, pp. 202-212, 2020.
[CrossRef] [Google Scholar] [Publisher Link]
[15] Subhrajit Roy, Isabell Kiral-Kornek, and Stefan Harrer, “Chrononet: A Deep Recurrent Neural Network for Abnormal EEG Identification,” Artificial Intelligence in Medicine, pp. 47-56, 2019.
[CrossRef] [Google Scholar] [Publisher Link]
[16] Sirwan Tofiq Jaafar, and Mokhtar Mohammadi, “Epileptic Seizure Detection Using Deep Learning Approach,” UHD Journal of Science and Technology, vol. 3, no. 2, pp. 41-50, 2019.
[CrossRef] [Google Scholar] [Publisher Link]
[17] Xuhui Chen et al., “Cost-Sensitive Deep Active Learning for Epileptic Seizure Detection,” Proceedings of the 2018 ACM International Conference on Bioinformatics, Computational Biology, and Health Informatics, pp. 226-235, 2018.
[CrossRef] [Google Scholar] [Publisher Link]
[18] Mustafa Sameer, and Bharat Gupta, “Detection of Epileptical Seizures Based on Alpha Band Statistical Features,” Wireless Personal Communications: An International Journal, vol. 115, no. 2, pp. 909-925, 2020.
[CrossRef] [Google Scholar] [Publisher Link]
[19] S. Raghu et al., “A Novel Approach for Classification of Epileptic Seizures Using Matrix Determinant,” Expert Systems with Applications, vol. 127, pp. 323-341, 2019.
[CrossRef] [Google Scholar] [Publisher Link]
[20] Mustafa Sameer, and Bharat Gupta, “ROC Analysis of EEG Subbands for Epileptic Seizure Detection Using Naive Bayes Classifier,” Journal of Mobile Multimedia, vol. 17, no. 1-3, pp. 299-310, 2021.
[CrossRef] [Google Scholar] [Publisher Link]
[21] Abeg Kumar Jaiswal, and Haider Banka, “Epileptic Seizure Detection in EEG Signal with GModPCA and Support Vector Machine,” BioMedical Materials and Engineering, vol. 28, no. 2, pp. 141-157, 2017.
[CrossRef] [Google Scholar] [Publisher Link]
[22] Sai Manohar Beeraka et al., “Accuracy Enhancement of Epileptic Seizure Detection: A Deep Learning Approach with Hardware Realization of STFT,” Circuits, Systems, and Signal Processing, vol. 41, pp. 461-484, 2022.
[CrossRef] [Google Scholar] [Publisher Link]
[23] Mustafa Sameer, and Bharat Gupta, “Time-Frequency Statistical Features of Delta Band for Detection of Epileptic Seizures,” Wireless Personal Communications, vol. 122, pp. 489-499, 2022.
[CrossRef] [Google Scholar] [Publisher Link]
[24] Arpana Mahajan et al., “A Novel Approach on Epileptic Seizures Detection Using Convolutional Neural Network,” 2020 4th International Conference on Electronics, Communication and Aerospace Technology (ICECA), Coimbatore, India, pp. 1541-1545, 2020.
[CrossRef] [Google Scholar] [Publisher Link]
[25] Manish Sharma, Sohamkumar Patel, and U. Rajendra Acharya, “Automated Detection of Abnormal EEG Signals Using Localised Wavelet Filter Banks,” Pattern Recognition Letters, vol. 133, pp. 188-194, 2020.
[CrossRef] [Google Scholar] [Publisher Link]