Integrating ANFIS for Improved MPPT in A 24V PEMFC System with Switched Inductor Boost Converter
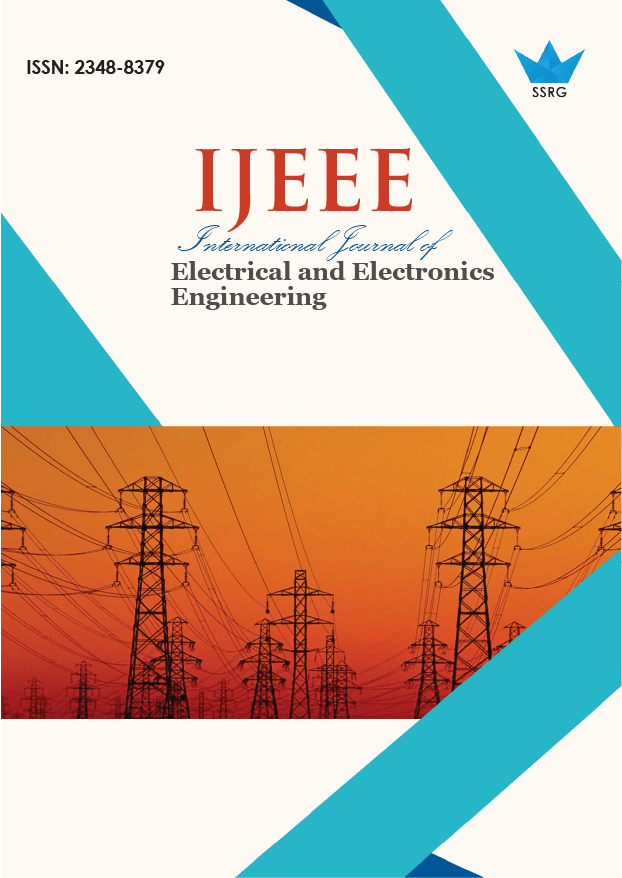
International Journal of Electrical and Electronics Engineering |
© 2024 by SSRG - IJEEE Journal |
Volume 11 Issue 5 |
Year of Publication : 2024 |
Authors : E. Kalaiyarasan, S. Singaravelu |
How to Cite?
E. Kalaiyarasan, S. Singaravelu, "Integrating ANFIS for Improved MPPT in A 24V PEMFC System with Switched Inductor Boost Converter," SSRG International Journal of Electrical and Electronics Engineering, vol. 11, no. 5, pp. 223-234, 2024. Crossref, https://doi.org/10.14445/23488379/IJEEE-V11I5P120
Abstract:
This study presents a comprehensive analysis of a 1.26 kW, 24V Proton Exchange Membrane Fuel Cell (PEMFC) system integrated with an Adaptive Neuro-Fuzzy Inference System (ANFIS) based Maximum Power Point Tracking (MPPT) control and a switched inductor DC-to-DC boost converter. The converter is designed to achieve an output voltage of 220V. The primary objectives of this research are to model the PEMFC system, design the switched inductor DC-to-DC boost converter and develop an ANFIS model for MPPT control. Simulations are conducted to observe the fuel cell voltage and current under varying air and water pressure conditions and the dynamics in the fuel cell parameters are analyzed. Additionally, the study investigates the impact of temperature variations on the fuel cell's performance. The converter output voltage and power are observed under Standard Test Conditions (STC) to evaluate the overall system efficiency. A key focus of the research is the comparison between the ANFIS-based MPPT control and Artificial Neural Network (ANN) based MPPT control for the Switched Inductor DC-to-DC Boost Converter (SIDC) output. The comparative analysis considers variations in air and water pressure as well as temperature changes. The results reveal insights into the dynamic behavior of the PEMFC system under different operating conditions. Furthermore, the comparative study between ANFIS and ANN highlights the efficacy of ANFIS in optimizing the converter output. The proposed model and control strategies contribute to the advancement of PEMFC technology, which offers a valuable foundation for the design and optimization of fuel cell systems in various environmental conditions. This research aligns with the growing interest in renewable energy sources and underscores the importance of advanced control strategies for enhancing the performance of fuel cell systems.
Keywords:
PEMFC, ANFIS, MPPT, Temperature, Smart control.
References:
[1] Miriam M. Tellez-Cruz et al., “Proton Exchange Membrane Fuel Cells (PEMFCs): Advances and Challenges,” Polymers, vol. 13, no. 18, pp. 1-54, 2021.
[CrossRef] [Google Scholar] [Publisher Link]
[2] Alexander Kraytsberg, and Yair Ein-Eli, “Review of Advanced Materials for Proton Exchange Membrane Fuel Cells,” Energy & Fuels, vol. 28, no. 12, pp. 7303-7330, 2014.
[CrossRef] [Google Scholar] [Publisher Link]
[3] Norazlianie Sazali et al., “New Perspectives on Fuel Cell Technology: A Brief Review,” Membranes, vol. 10, no. 5, pp. 1-18, 2020.
[CrossRef] [Google Scholar] [Publisher Link]
[4] Yun Wang et al., “Materials, Technological Status, and Fundamentals of PEM Fuel Cells - A Review,” Materials Today, vol. 32, pp. 178203, 2020.
[CrossRef] [Google Scholar] [Publisher Link]
[5] Christopher Herwerth et al., “Development of a Small Long Endurance Hybrid PEM Fuel Cell Powered UAV,” SAE Technical Paper, pp. 1-10, 2007.
[CrossRef] [Google Scholar] [Publisher Link]
[6] Ephraim Bonah Agyekum et al., “Research Progress, Trends, and Current State of Development on PEMFC-New Insights from a Bibliometric Analysis and Characteristics of Two Decades of Research Output,” Membranes, vol. 12, no. 11, pp. 1-33, 2022.
[CrossRef] [Google Scholar] [Publisher Link]
[7] Kui Jiao et al., “Designing the Next Generation of Proton-Exchange Membrane Fuel Cells,” Perspectives, vol. 595, pp. 361-369, 2021.
[CrossRef] [Google Scholar] [Publisher Link]
[8] Linda Ager-Wick Ellingsen et al., “Nanotechnology for Environmentally Sustainable Electromobility,” Nature Nanotechlogy, vol. 11, pp. 1039-1051, 2016.
[CrossRef] [Google Scholar] [Publisher Link]
[9] Nisa Nur Atak, Battal Dogan, and Murat Kadir Yesilyurt, “Investigation of the Performance Parameters for a PEMFC by Thermodynamic Analyses: Effects of Operating Temperature and Pressure,” Energy, vol. 282, 2023.
[CrossRef] [Google Scholar] [Publisher Link]
[10] Saad Motahhir, Aboubakr El Hammoumi, and Abdelaziz El Ghzizal, “The Most Used MPPT Algorithms: Review and the Suitable LowCost Embedded Board for Each Algorithm,” Journal of Cleaner Production, vol. 246, 2020.
[CrossRef] [Google Scholar] [Publisher Link]
[11] Liping Fan, and Xianyang Ma, “Maximum Power Point Tracking of PEMFC Based on Hybrid Artificial Bee Colony Algorithm with Fuzzy Control,” Scientific Reports, vol. 12, pp. 1-12, 2022.
[CrossRef] [Google Scholar] [Publisher Link]
[12] Abdelfatah Kolli et al., “A Review on DC/DC Converter Architectures for Power Fuel Cell Applications,” Energy Conversion and Management, vol. 105, pp. 716-730, 2015.
[CrossRef] [Google Scholar] [Publisher Link]
[13] W.R.W. Daud et al., “PEM Fuel Cell System Control: A Review,” Renewable Energy, vol. 113, pp. 620-638, 2017.
[CrossRef] [Google Scholar] [Publisher Link]
[14] Pascal Kuate Nkounhawa, Dieunedort Ndapeu, and Bienvenu Kenmeugne, “Artificial Neural Network (ANN) and Adaptive Neuro-Fuzzy Inference System (ANFIS): Application for a Photovoltaic System under Unstable Environmental Conditions,” International Journal of Energy and Environmental Engineering, vol. 13, pp. 821-829, 2022.
[CrossRef] [Google Scholar] [Publisher Link]
[15] Yun Wang et al., “A Review of Polymer Electrolyte Membrane Fuel Cells: Technology, Applications, and Needs on Fundamental Research,” Applied Energy, vol. 88, no. 4, pp. 981-1007, 2011.
[CrossRef] [Google Scholar] [Publisher Link]
[16] Adam Z. Weber, Sivagaminathan Balasubramanian, and Prodip K. Das, “Chapter 2 Proton Exchange Membrane Fuel Cells,” Advances in Chemical Engineering, vol. 41, pp. 66-144, 2012.
[CrossRef] [Google Scholar] [Publisher Link]
[17] Ahmed Al-Hmouz et al., “Modeling and Simulation of an Adaptive Neuro-Fuzzy Inference System (ANFIS) for Mobile Learning,” IEEE Transactions on Learning Technologies, vol. 5, no. 3, pp. 226-237, 2012.
[CrossRef] [Google Scholar] [Publisher Link]