Synchrophasor-Driven Machine Learning Application for Enhanced Power System Stability Monitoring: A Comprehensive Review
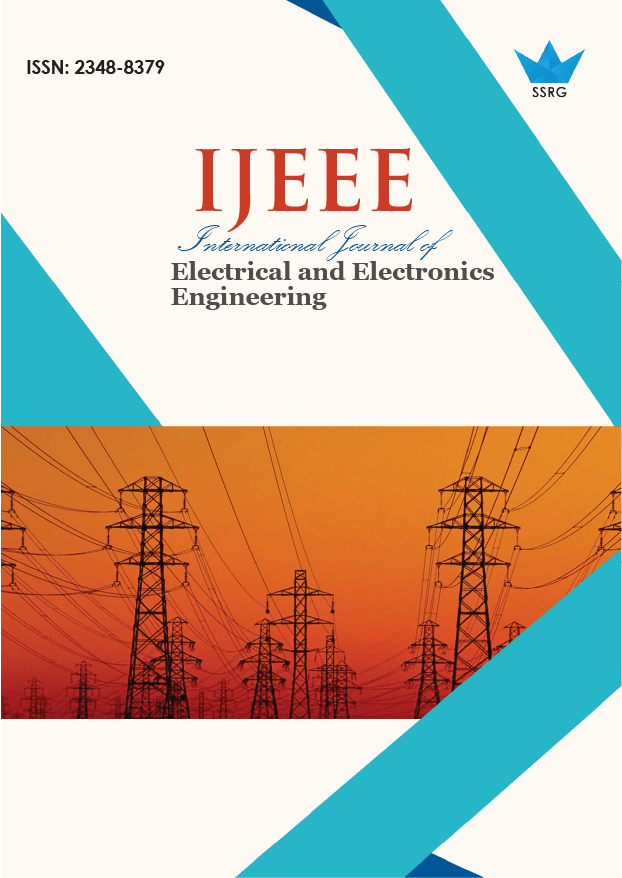
International Journal of Electrical and Electronics Engineering |
© 2024 by SSRG - IJEEE Journal |
Volume 11 Issue 5 |
Year of Publication : 2024 |
Authors : Kunal Samad, Arunkumar Patil, Katkar Siddhant Satyapal, Santosh Diggikar |
How to Cite?
Kunal Samad, Arunkumar Patil, Katkar Siddhant Satyapal, Santosh Diggikar, "Synchrophasor-Driven Machine Learning Application for Enhanced Power System Stability Monitoring: A Comprehensive Review," SSRG International Journal of Electrical and Electronics Engineering, vol. 11, no. 5, pp. 242-260, 2024. Crossref, https://doi.org/10.14445/23488379/IJEEE-V11I5P122
Abstract:
The continuous increase in complexity and nature of loads in a power system has given rise to unknown inevitable risks hidden from the Power System Operator (PSOs). All the electric equipment in a power grid are assets to a utility whose ultimate goal is to prevent severe damage to these significant electrical components caused by disturbances within a power system. Several utilities are transitioning towards an intelligent grid. Advanced communication technology and the revolution in Artificial Intelligence (AI) have helped PSOs to accurately determine the state of any dynamic power system, providing an observable and comprehensible power grid. First, the paper explores the concept and significance of big data by highlighting some unique characteristics of synchrophasor measurement generated at every interval in conjunction with traditional data. Then, there are challenges in data acquisition and processing techniques while handling these raw data. Also, the methodology and standards are considered for verifying the reliability of synchrophasor data before any online and offline power system study. Secondly, the paper emphasizes the importance of stability analysis for identifying vulnerable points within the network prone to voltage collapse. It will also discuss advancements in simulation studies and control strategies followed by operators to identify critical contingencies and implement corrective measures, enhancing the overall security of a power system using wide-area monitoring systems. Subsequently, the paper focuses on WAMS Enabled Machine Learning Approaches for Power System Stability. This segment comprehensively covers transient and voltage stability assessments, showcasing the innovative intersection of machine learning techniques with WAMS technology. The discussion sheds light on various applications of machine learning in the realm of power system stability.
Keywords:
Synchrophasor technology, Wide Area Monitoring System (WAMS), Phasor Measurement Unit (PMUs), Synchrophasor technology, Big data, Artificial Intelligence, Machine Learning, Power System Analysis, Transient Stability Analysis and Voltage Stability Analysis.
References:
[1] Vladimir Terzija et al., “Wide-Area Monitoring, Protection, and Control of Future Electric Power Networks,” Proceedings of the IEEE, vol. 99, no. 1, pp. 80-93, 2011.
[CrossRef] [Google Scholar] [Publisher Link]
[2] Alok Jain et al., “Implementation Techniques for Frequency & Phasor Estimation in Phasor Measurement Units (PMUs),” 2018 3rd International Conference on Internet of Things: Smart Innovation and Usages (IoT-SIU), Bhimtal, India, pp. 1-6, 2018.
[CrossRef] [Google Scholar] [Publisher Link]
[3] Prachi Mafidar Joshi, and H.K. Verma, “Synchrophasor Measurement Applications and Optimal PMU Placement: A Review,” Electric Power Systems Research, vol. 199, 2021.
[CrossRef] [Google Scholar] [Publisher Link]
[4] M. Dhinu Lal, and Ramesh Varadarajan, “A Review of Machine Learning Approaches in Synchrophasor Technology,” IEEE Access, vol. 11, pp. 33520-33541, 2023.
[CrossRef] [Google Scholar] [Publisher Link]
[5] M. Kezunovic, and A. Abur, “Emerging the Temporal and Spatial Aspects of Data and Information for Improved Power System Monitoring Applications,” Proceedings of the IEEE, vol. 93, no. 11, pp. 1909-1919, 2005.
[CrossRef] [Google Scholar] [Publisher Link]
[6] N.S. Sodha et al., “Phasor Measurement Applications in Smart Grid,” Power Engineer Journal, vol. 15, no. 1, pp. 4-13, 2013.
[Google Scholar] [Publisher Link]
[7] Ikponmwosa Idehen, Wonhyeok Jang, and Thomas Overbye, “PMU Data Feature Considerations for Realistic, Synthetic Data Generation,” Proceedings of the 2019 North American Power Symposium (NAPS), Wichita, USA, pp. 1-6, 2019.
[CrossRef] [Google Scholar] [Publisher Link]
[8] EPFL, EPFL Smart Grid – The Vision. [Online]. Available: https://www.epfl.ch/labs/desl-pwrs/smartgrid/
[9] North American Synchrophasor Initiative (NAPSI), PMU Data, 2020. [Online]. Available: https://www.naspi.org/
[10] Phani Harsha Gadde et al., “Efficient Compression of PMU Data in WAMS,” IEEE Transactions on Smart Grid, vol. 7, no. 5, pp. 24062413, 2016.
[CrossRef] [Google Scholar] [Publisher Link]
[11] Makarand Ballal, Amit Kulkarni, and Hiralal Suryawanshi, “Data Compression Techniques for Phasor Measurement Unit (PMU) Applications in Smart Transmission Grid,” International Journal of Emerging Electric Power Systems, vol. 21, no. 3, 2020.
[CrossRef] [Google Scholar] [Publisher Link]
[12] Tianshu Bi et al., “The Impact of Time Synchronization Deviation on the Performance of Synchrophasor Measurements and Wide Area Damping Control,” IEEE Transactions on Smart Grid, vol. 8, no. 4, pp. 1545-1552, 2017.
[CrossRef] [Google Scholar] [Publisher Link]
[13] M.S. Almas et al., “Vulnerability of Synchrophasor-Based WAMPAC Applications’ to Time Synchronization Spoofing,” IEEE Transactions on Smart Grid, vol. 9, no. 5, pp. 4601-4612, 2018.
[CrossRef] [Google Scholar] [Publisher Link]
[14] “IEEE Standard for Synchrophasor Data Transfer for Power Systems,” IEEE Std C37.118.2-2011 (Revision of IEEE Std C37.118-2005), pp.1-53, 2011.
[CrossRef] [Google Scholar] [Publisher Link]
[15] Jue Li et al., “Electronic Transformer Performance Evaluation and Its Impact on PMU,” IET Generation, Transmission & Distribution, vol. 13, no. 23, pp. 5396-5403, 2019.
[CrossRef] [Google Scholar] [Publisher Link]
[16] Can Huang et al., “Data Quality Issues for Synchrophasor Applications Part II: Problem Formulation and Potential Solutions,” Journal of Modern Power Systems and Clean Energy, vol. 4, no. 3, pp. 353-361, 2016.
[CrossRef] [Google Scholar] [Publisher Link]
[17] Xinran Zhang et al., “Experimental Test of PMU Measurement Errors and the Impact on Load Model Parameter Identification,” IET Generation, Transmission & Distribution, vol. 14, no. 20, pp. 4593-4604, 2020.
[CrossRef] [Google Scholar] [Publisher Link]
[18] Hua Lin et al., “A Study of Communication and Power System Infrastructure Interdependence on PMU-Based Wide Area Monitoring and Protection,” 2012 IEEE Power and Energy Society General Meeting, San Diego, USA, pp. 1-7, 2012.
[CrossRef] [Google Scholar] [Publisher Link]
[19] Houda Daki et al., “Big Data Management in Smart Grid: Concepts, Requirements and Implementation,” Journal of Big Data, vol. 4, pp. 1-19, 2017.
[CrossRef] [Google Scholar] [Publisher Link]
[20] Mania Pavella, “Power System Transient Stability Assessment - Traditional vs Modern Methods,” Control Engineering Practice, vol. 6, no. 10, pp. 1233-1246, 1998. [CrossRef] [Google Scholar] [Publisher Link]
[21] Cheng-Jin Ye, and Min-Xiang Huang, “Multi-Objective Optimal Power Flow Considering Transient Stability Based on Parallel NSGAII,” IEEE Transactions on Power Systems, vol. 30, no. 2, pp. 857-866, 2015.
[CrossRef] [Google Scholar] [Publisher Link]
[22] Md. Kamal Hossain, and Mohd. Hasan Ali, “Transient Stability Augmentation of PV/DFIG/SG-Based Hybrid Power System by Nonlinear Control-Based Variable Resistive FCL,” IEEE Transactions on Sustainable Energy, vol. 6, no. 4, pp. 1638-1649, 2015.
[CrossRef] [Google Scholar] [Publisher Link]
[23] L. Wehenkel, Th. Van Cutsem, and M. Ribbens-Pavella, “Inductive Inference Applied to On-Line Transient Stability Assessment of Electric Power Systems,” Automatica, vol. 25, no. 3, pp. 445-451, 1989.
[CrossRef] [Google Scholar] [Publisher Link]
[24] Tingyan Guo, and Jovica V. Milanović, “Probabilistic Framework for Assessing the Accuracy of Data Mining Tool for Online Prediction of Transient Stability,” IEEE Transactions on Power Systems, vol. 29, no. 1, pp. 377-385, 2014.
[CrossRef] [Google Scholar] [Publisher Link]
[25] Yue Yang et al., “Measurement-Based Cell-DT Method for Power System Transient Stability Classification,” CSEE Journal of Power and Energy Systems, vol. 3, no. 3, pp. 278-285, 2017.
[CrossRef] [Google Scholar] [Publisher Link]
[26] Liangyu Ma, Lijuan Zhao, and Xiaoxia Wang, “Prediction of Thermal System Parameters Based on PSO-ELM Hybrid Algorithm,” 2017 Chinese Automation Congress (CAC), Jinan, China, pp. 3136-3141, 2017.
[CrossRef] [Google Scholar] [Publisher Link]
[27] M. Cortes-Carmona, G. Jimenez-Estevez, and J. Guevara-Cedeno, “Support Vector Machines for On-Line Security Analysis of Power Systems,” 2008 IEEE/PES Transmission and Distribution Conference and Exposition: Latin America, Bogota, Colombia, pp. 1-6, 2008.
[CrossRef] [Google Scholar] [Publisher Link]
[28] Nicholas Gregory Baltas et al., “A Comparative Analysis of Decision Trees, Support Vector Machines, and Artificial Neural Networks for On-line Transient Stability Assessment,” 2018 International Conference on Smart Energy Systems and Technologies (SEST), pp. 1-6, 2018.
[CrossRef] [Google Scholar] [Publisher Link]
[29] Yang Li, and Zhen Yang, “Application of EOS-ELM with Binary Jaya Based Feature Selection to Real-Time Transient Stability Assessment Using PMU Data,” IEEE Access, vol. 5, pp. 23092-23101, 2017.
[CrossRef] [Google Scholar] [Publisher Link]
[30] Yang Li, and Xueping Gu, “Feature Selection for Transient Stability Assessment Based on Improved Maximal Relevance and Minimal Redundancy Criterion,” arXiv, pp. 1-8, 2019.
[CrossRef] [Google Scholar] [Publisher Link]
[31] Jun Liu et al., “An Improved Power System Transient Stability Prediction Model Based on mRMR Feature Selection and WTA Ensemble Learning,” Applied Sciences, vol. 10, no. 7, pp. 1-17, 2020.
[CrossRef] [Google Scholar] [Publisher Link]
[32] Yanjun Zhang et al., “Optimized Extreme Learning Machine for Power System Transient Stability Prediction Using Synchrophasors,” Mathematical Problems in Engineering, vol. 2015, pp. 1-8, 2015.
[CrossRef] [Google Scholar] [Publisher Link]
[33] Jiashu Fang et al., “A Data-Driven Method for Online Transient Stability Monitoring with Vision-Transformer Networks,” International Journal of Electrical Power & Energy Systems, vol. 149, 2023.
[CrossRef] [Google Scholar] [Publisher Link]
[34] Bendong Tan et al., “A Deep Imbalanced Learning Framework for Transient Stability Assessment of Power System,” IEEE Access, vol. 7, pp. 81759-81769, 2019. [CrossRef] [Google Scholar] [Publisher Link]
[35] Tohid Behdadnia, Yusuf Yaslan, and Istemihan Genc, “A New Method of Decision Tree Based Transient Stability Assessment Using Hybrid Simulation for Real-Time PMU Measurements,” IET Generation, Transmission & Distribution, vol. 15, no. 4, pp. 678-693, 2021.
[CrossRef] [Google Scholar] [Publisher Link]
[36] Xin Li et al., “An Intelligent Transient Stability Assessment Framework with Continual Learning Ability,” IEEE Transactions on Industrial Informatics, vol. 17, no. 12, pp. 8131-8141, 2021.
[CrossRef] [Google Scholar] [Publisher Link]
[37] Anqi He et al., “Machine Learning Based Method for Transient Stability Assessment of Power Systems,” 2023 IEEE 3rd International Conference on Power, Electronics and Computer Applications (ICPECA), Shenyang, China, pp. 1165-1168, 2023.
[CrossRef] [Google Scholar] [Publisher Link]
[38] Izzuddin Fathin Azhar, Lesnanto Multa Putranto, and Roni Irnawan, “Development of PMU-Based Transient Stability Detection Methods Using CNN-LSTM Considering Time Series Data Measurement,” Energies, vol. 15, no. 21, pp. 1-20, 2022.
[CrossRef] [Google Scholar] [Publisher Link]
[39] Xiaoyan Han et al., “LSTM-CNN-Based Transient Stability Assessment,” 2021 International Conference on Power System Technology (POWERCON), Haikou, China, pp. 1930-1935, 2021.
[CrossRef] [Google Scholar] [Publisher Link]
[40] Y. Liu, P. Zhang, and J. Zhao, “Convolutional Neural Network and Long Short-Term Memory Based Deep Learning Model for Transient Stability Assessment,” IEEE Transactions on Industrial Informatics, vol. 15, no. 6, pp. 3593-3602, 2019.
[41] M. Jiang, X. Zhang, and F. Li, “Transformer-Based Model for Transient Stability Assessment in Large-Scale Power Systems,” IEEE Transactions on Smart Grid, vol. 12, no. 3, pp. 2016-2025, 2021.
[42] X. Zhou, B. Zhang, and Y. Lin, “Transient Stability Prediction Using Deep Belief Networks,” IET Generation, Transmission & Distribution, vol. 14, no. 7, pp. 1348-1355, 2020.
[43] Salah Mokred, Yifei Wang, and Tiancong Chen, “Modern Voltage Stability Index for Prediction of Voltage Collapse and Estimation of Maximum Load-Ability for Weak Buses and Critical Line Identification,” International Journal of Electrical Power & Energy Systems, vol. 145, 2023.
[CrossRef] [Google Scholar] [Publisher Link]
[44] Bazilah Ismail et al., “New Line Voltage Stability Index (BVSI) for Voltage Stability Assessment in Power System: The Comparative Studies,” IEEE Access, vol. 10, pp. 103906-103931, 2022.
[CrossRef] [Google Scholar] [Publisher Link]
[45] Tong Su et al., “Deep Belief Network Enabled Surrogate Modeling for Fast Preventive Control of Power System Transient Stability,” IEEE Transactions on Industrial Informatics, vol. 18, no. 1, pp. 315-326, 2022.
[CrossRef] [Google Scholar] [Publisher Link]
[46] A.R. Bahmanyar, and A. Karami, “Power System Voltage Stability Monitoring Using Artificial Neural Networks with A Reduced Set of Inputs,” International Journal of Electrical Power & Energy Systems, vol. 58, pp. 246-256, 2014.
[CrossRef] [Google Scholar] [Publisher Link]
[47] Debbie Q. Zhou, Udaya D. Annakkage, and Athula D. Rajapakse, “Online Monitoring of Voltage Stability Margin Using an Artificial Neural Network,” IEEE PES General Meeting, Minneapolis, USA, pp. 1-1, 2010.
[CrossRef] [Google Scholar] [Publisher Link]
[48] O.P. Rahi et al., “Power System Voltage Stability Assessment through Artificial Neural Network,” Procedia Engineering, vol. 30, pp. 5360, 2012.
[CrossRef] [Google Scholar] [Publisher Link]
[49] A. Senthil Kumar, S. Rajasekar, and P. Ajay-D-Vimal Raj, “Power Quality Profile Enhancement of Utility Connected Microgrid System Using ANFIS-UPQC,” Procedia Technology, vol. 21, pp. 112-119, 2015.
[CrossRef] [Google Scholar] [Publisher Link]
[50] Duotong Yang et al., “Adaptive Decision-Trees-Based Regional Voltage Control,” 2017 North American Power Symposium (NAPS), Morgantown, USA, pp. 1-6, 2017. [CrossRef] [Google Scholar] [Publisher Link]
[51] Santiago P. Torres, Washington H. Peralta, and Carlos A. Castro, “Power System Loading Margin Estimation Using a Neuro-Fuzzy Approach,” IEEE Transactions on Power Systems, vol. 22, pp. 1955-1964, 2007.
[CrossRef] [Google Scholar] [Publisher Link]
[52] Xiangfei Meng et al., “Construction of Decision Tree Based on C4.5 Algorithm for Online Voltage Stability Assessment,” International Journal of Electrical Power & Energy Systems, vol. 118, 2020.
[CrossRef] [Google Scholar] [Publisher Link]
[53] Weijie Li et al., “Comparison of Decision Tree Attribute Selection Methods for Static Voltage Stability Margin Assessment,” 2018 IEEE 2nd International Electrical and Energy Conference (CIEEC), Beijing, China, pp. 201-206, 2018.
[CrossRef] [Google Scholar] [Publisher Link]
[54] Mohammed Amroune, Tarek Bouktir, and Ismail Musirin, “Power System Voltage Stability Assessment Using a Hybrid Approach Combining Dragonfly Optimization Algorithm and Support Vector Regression,” Arabian Journal for Science and Engineering, vol. 43, pp. 3023-3036, 2018.
[CrossRef] [Google Scholar] [Publisher Link]
[55] Hanieh Mohammadi et al., “Voltage Stability Assessment Using Multi-Objective Biogeography-Based Subset Selection,” International Journal of Electrical Power & Energy Systems, vol. 103, 2018.
[CrossRef] [Google Scholar] [Publisher Link]
[56] Khanh Pham et al., “Ensemble Learning-Based Classification Models for Slope Stability Analysis,” Catena, vol. 196, 2021.
[CrossRef] [Google Scholar] [Publisher Link]
[57] Yuchen Zhang et al., “A Missing-Data Tolerant Method for Data-Driven Short-Term Voltage Stability Assessment of Power Systems,” IEEE Transactions on Smart Grid, vol. 10, no. 5, pp. 5663-5674, 2019.
[CrossRef] [Google Scholar] [Publisher Link]
[58] Salim S. Maaji et al., “On-Line Voltage Stability Monitoring Using an Ensemble AdaBoost Classifier,” 2018 4th International Conference on Information Management (ICIM), Oxford, UK, pp. 253-259, 2018.
[CrossRef] [Google Scholar] [Publisher Link]
[59] Tonglin Luo, and Xuchu Jiang, “A Novel Multi-Task Learning Method for Evaluating Short-Term Voltage Stability with Incomplete PMU Measurements,” Complex & Intelligent Systems, vol. 10, pp. 1971-1983, 2024.
[CrossRef] [Google Scholar] [Publisher Link]
[60] Zhujun Zhu et al., “Static Voltage Stability Assessment Using a Random Under Sampling Bagging BP Method,” Processes, vol. 10, no. 10, pp. 1-14, 2022.
[CrossRef] [Google Scholar] [Publisher Link]
[61] Y. Zhang et al., “LSTM for Short-Term Voltage Stability,” 2021.
[62] Zijian Feng et al., “Transferable Deep Learning Power System Short-Term Voltage Stability Assessment with Physics-Informed Topological Feature Engineering,” arXiv, pp. 1-7, 2023.
[CrossRef] [Google Scholar] [Publisher Link]
[63] Yang Li et al., “PMU Measurements-Based Short-Term Voltage Stability Assessment of Power Systems via Deep Transfer Learning,” IEEE Transactions on Instrumentation and Measurement, vol. 72, 2023.
[CrossRef] [Google Scholar] [Publisher Link]
[64] Syed Muhammad Hur Rizvi, Sajan K. Sadanandan, and Anurag K. Srivastava, “Data-Driven Short-Term Voltage Stability Assessment Using Convolutional Neural Networks Considering Data Anomalies and Localization,” IEEE Access, vol. 9, pp. 128345-128358, 2021.
[CrossRef] [Google Scholar] [Publisher Link]