Grading of Retinal Hard Exudates by Using VistaView Devise Based on Hybrid Algorithm
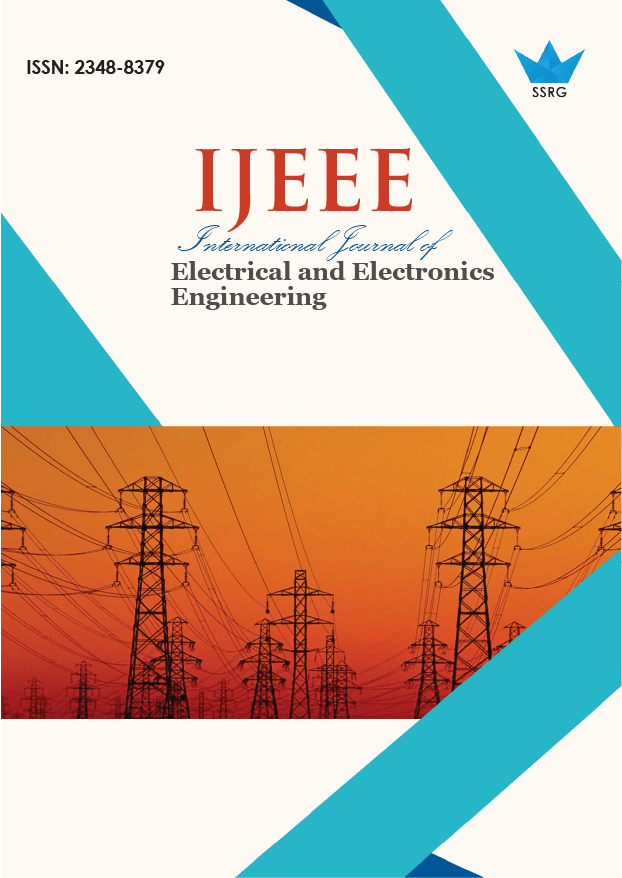
International Journal of Electrical and Electronics Engineering |
© 2024 by SSRG - IJEEE Journal |
Volume 11 Issue 6 |
Year of Publication : 2024 |
Authors : Suhair Hussein Talib, Osama Qasim |
How to Cite?
Suhair Hussein Talib, Osama Qasim, "Grading of Retinal Hard Exudates by Using VistaView Devise Based on Hybrid Algorithm," SSRG International Journal of Electrical and Electronics Engineering, vol. 11, no. 6, pp. 41-49, 2024. Crossref, https://doi.org/10.14445/23488379/IJEEE-V11I6P105
Abstract:
Untreated Diabetic Retinopathy (DR) can lead to different levels of visual impairment and potentially complete loss of eyesight. Undergoing an annual eye examination can be immensely advantageous in identifying prospective visual impairments, particularly for individuals with diabetes, aiding in preventing early-stage vision deterioration. Due to technological improvements, retinal imaging technologies for cell phones are now commercially available. The remarkable feature of retinal imaging systems is their capacity to conduct cost-effective and efficient screening for diabetic retinopathy in diverse settings. Nevertheless, the accuracy of DR detection can be influenced by the area of view and image quality. Due to their small size, smartphone-based retinal imaging plans typically produce images of inferior quality and have a narrower field of vision than traditional fundus cameras. The goal of this study is to thoroughly examine several approaches to handling retinal images in the field of ophthalmology in order to identify and assess the degree of abnormalities. The research endeavors to obtain retinal images with cutting-edge imaging technologies such as the Vista view. On the other hand, fundus cameras are too expensive and heavy for most health clinics to buy and transport. Thus, compact, portable, and reasonable retinal imaging technologies for quick DR screening are needed. Every health clinic cannot afford fundus cameras, which are bulky and cumbersome. Thus, quick DR screening retinal imaging systems that are tiny, portable, and affordable are in demand. Then examined the view field of commercial smartphone-based portable ophthalmoscope systems to assess whether they are acceptable for DR screening during a general health exam. The Vistaview retinal imaging system has better image quality and an extensive field of view than other devices. It then makes use of a variety of image-enhancing algorithms to raise the photographs' quality and clarity. The researchers investigated the effectiveness of MRLS, a multi-level rhombus segmentation method, for identifying lesions in retinal images caused by conditions like diabetic retinopathy and macular degeneration. This method uses median filters and morphological processes to identify anomalies automatically. The assessment of lesions created on their severity and progression is another area of investigation in this study. In order to do this, an Artificial Neural Network (ANN) system based on the ANN's Delta Learning Rule (DLR) is proposed. The resolve of this system is to identify images and control whether a dangerous situation is present. This work is a significant step towards the development of reliable and effective methods for the prompt diagnosis and treatment of retinal disorders.
Keywords:
VistaView, Diabetic Retinopathy (DR), MRLS, Median filter, DLR.
References:
[1] Ramachandran Rajalakshmi et al., “Review of Retinal Cameras for Global Coverage of Diabetic Retinopathy Screening,” Eye, vol. 35, pp. 162-172, 2021.
[CrossRef] [Google Scholar] [Publisher Link]
[2] Susmit Das et al., “Feasibility and Clinical Utility of Handheld Fundus Cameras for Retinal Imaging,” Eye, vol. 37, pp. 274-279, 2023.
[3] Yung-Hui Li et al., “Computer-Assisted Diagnosis for Diabetic Retinopathy Based on Fundus Images Using Deep Convolutional Neural Network,” Mobile Information Systems, 2019.
[CrossRef] [Google Scholar] [Publisher Link]
[4] Petteri Teikari et al., “Embedded Deep Learning in Ophthalmology: Making Ophthalmic Imaging Smarter,” Therapeutic Advances in Ophthalmology, vol. 11, pp. 1-21, 2019.
[CrossRef] [Google Scholar] [Publisher Link]
[5] Hossein Nazari Khanamiri, Austin Nakatsuka, and Jaafar El-Annan, “Smartphone Fundus Photography,” Medicine, pp. 1-5, 2017.
[CrossRef] [Google Scholar] [Publisher Link]
[6] Silke Aumann et al., “Optical Coherence Tomography (OCT): Principle and Technical Realization,” High Resolution Imaging in Microscopy and Ophthalmology, pp. 59-85, 2019.
[CrossRef] [Google Scholar] [Publisher Link]
[7] Laura K. Young, and Hannah E. Smithson, “ERICA: Emulated Retinal Image Capture - A Tool for Testing, Training and Validating Retinal Image Processing Methods,” bioRxiv, pp. 1-15, 2021.
[CrossRef] [Google Scholar] [Publisher Link]
[8] Yehui Yang et al., “Lesion Detection and Grading of Diabetic Retinopathy via Two-Stages Deep Convolutional Neural Networks,” Medical Image Computing and Computer Assisted Intervention (MICCAI), pp. 533-540, 2017.
[CrossRef] [Google Scholar] [Publisher Link]
[9] Songhua Xie, and Hui Nie, “Retinal Vascular Image Segmentation Using Genetic Algorithm Plus FCM Clustering,” 2013 Third International Conference on Intelligent System Design and Engineering Applications, Hong Kong, China, pp. 1225-1228, 2013.
[CrossRef] [Google Scholar] [Publisher Link]
[10] Avleen Malhi, Reaya Grewal, and Husanbir Singh Pannu, “Detection and Diabetic Retinopathy Grading Using Digital Retinal Images,” International Journal of Intelligent Robotics and Applications, vol. 7, pp. 426-458, 2023.
[CrossRef] [Google Scholar] [Publisher Link]
[11] Yuqiang Zhang et al., “An O-Shape Neural Network with Attention Modules to Detect Junctions in Biomedical Images without Segmentation,” IEEE Journal of Biomedical and Health Informatics, vol. 26, no. 2, pp. 774-785, 2022.
[CrossRef] [Google Scholar] [Publisher Link]
[12] Kranthi Kumar Palavalasa, and Bhavani Sambaturu, “Automatic Diabetic Retinopathy Detetion Using Digital Image Processing,” 2018 International Conference on Communication and Signal Processing (ICCSP), Chennai, India, pp. 0072-0076, 2018.
[CrossRef] [Google Scholar] [Publisher Link]
[13] Harini R., and Sheela N., “Feature Extraction and Classifcation of Retinal Images for Automated Detection of Diabetic Retinopathy,” 2016 Second International Conference on Cognitive Computing and Information Processing (CCIP), Mysuru, India, pp. 1-4, 2016.
[CrossRef] [Google Scholar] [Publisher Link]
[14] Zhixi Li et al., “Using Deep Leaning Models to Detect Ophthalmic Diseases: A Comparative Study,” Frontiers in Medicine, vol. 10, pp. 1-11, 2023.
[CrossRef] [Google Scholar] [Publisher Link]
[15] Wei Zhou et al., “Automatic Detection of Exudates in Digital Color Fundus Images Using Superpixel Multi-Feature Classification,” IEEE Access, vol. 5, pp. 17077-17088, 2017.
[CrossRef] [Google Scholar] [Publisher Link]
[16] Dimple Nagpal et al., “A Review of Diabetic Retinopathy: Datasets, Approaches, Evaluation Metrics and Future Trends,” Journal of King Saud University - Computer and Information Sciences, vol. 34, no. 9, pp. 7138-7152, 2022.
[CrossRef] [Google Scholar] [Publisher Link]
[17] Dimple Nagpal et al., “Recent Advancement for Diagnosing Diabetic Retinopathy,” Journal of Computational and Theoretical Nanoscience, vol. 17, no. 11. pp. 5096-5104, 2020.
[CrossRef] [Google Scholar] [Publisher Link]
[18] Review of Optometry, Handheld Retinal Camera Allows On-the-Go Imaging, Volk’s Newest Combines Photography and Data Management in One Device, 2020. [Online]. Available: https://www.reviewofoptometry.com/article/handheld-retinal-camera-allowsonthego-imaging
[19] Volk, VistaView™ – Volk Optical. [Online]. Available: https://www.volk.com/pages/vistaview%E2%84%A2