AquaPredict: Deploying Data-Driven Aquatic Models for Optimizing Sustainable Agriculture Practices
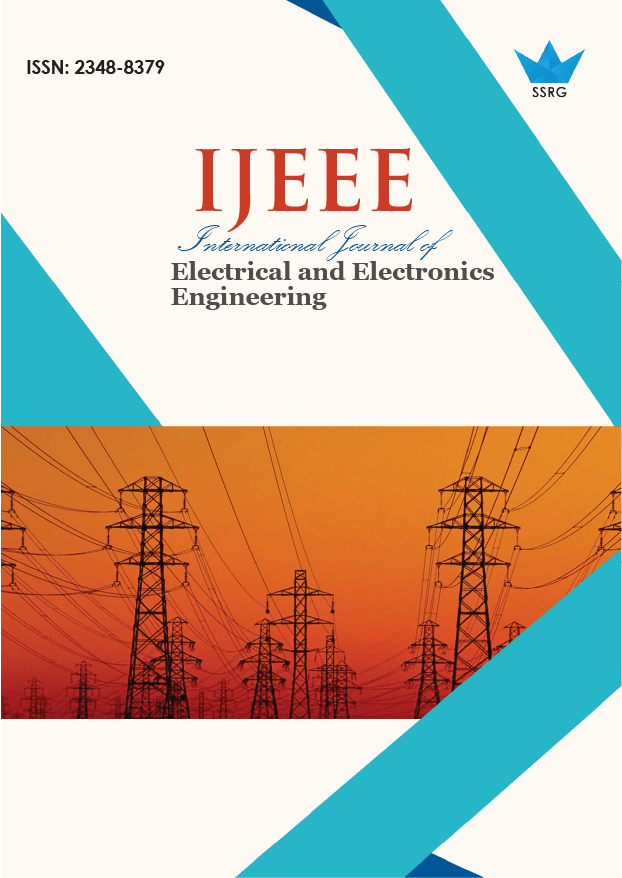
International Journal of Electrical and Electronics Engineering |
© 2024 by SSRG - IJEEE Journal |
Volume 11 Issue 6 |
Year of Publication : 2024 |
Authors : P. Laxmikanth, V. Vijayasherly, M. Mounika, Pendem Swetha, J Adilakshmi, M. Bhavsingh |
How to Cite?
P. Laxmikanth, V. Vijayasherly, M. Mounika, Pendem Swetha, J Adilakshmi, M. Bhavsingh, "AquaPredict: Deploying Data-Driven Aquatic Models for Optimizing Sustainable Agriculture Practices," SSRG International Journal of Electrical and Electronics Engineering, vol. 11, no. 6, pp. 76-90, 2024. Crossref, https://doi.org/10.14445/23488379/IJEEE-V11I6P109
Abstract:
This paper introduces AquaPredict, an innovative predictive framework designed to assess the impacts of agricultural practices on aquatic systems through advanced machine learning algorithms and the integration of diverse data sources. Utilizing Random Forests, Gradient Boosting Machines (GBMs), and Deep Neural Networks (DNNs), AquaPredict surpasses existing models like SWAT in predictive accuracy, precision, recall, F1 score, AUC-ROC, and other key metrics, demonstrating a marked improvement in the ability to forecast environmental outcomes. Through rigorous validation across various scenarios, including drought, heavy rainfall, and nutrient runoff, AquaPredict achieved notable accuracy (0.93), precision (0.95), and recall (0.91) alongside a low Log Loss (0.29) and high Explained Variance (0.93), underscoring its superior performance and reliability. The framework’s integration of satellite imagery, water quality sensors, and agricultural records into a coherent predictive model represents a significant contribution to sustainable agriculture, offering a nuanced understanding of the complex interplay between agricultural practices and aquatic ecosystem health. This paper not only details the development and evaluation of AquaPredict but also highlights its potential implications for informed decision-making in environmental management and policy. Recommendations for future research focus on expanding the model’s capabilities, including the incorporation of socio-economic factors and the exploration of emerging technologies, to further enhance its applicability and effectiveness in promoting sustainable agricultural practices and preserving aquatic environments.
Keywords:
Sustainable agriculture, Aquatic ecosystems, Machine Learning, Data integration, Environmental modeling, Predictive analytics.
References:
[1] Qazi Umar Farooq et al., “AgriAqua Intelligence: Advancing Smart Farming with Ecosystem-Based Water Management Solutions,” International Journal of Computer Engineering in Research Trends, vol. 11, no. 1, pp. 51–60, 2024.
[2] K. Saikanth et al., “Advancing Sustainable Agriculture: A Comprehensive Review for Optimizing Food Production and Environmental Conservation,” International Journal of Plant & Soil Science, vol. 35, no. 16, pp. 417-425, 2023.
[Google Scholar]
[3] K.K. Priya et al., “Towards a Greener Tomorrow: The Role of Data Science in Shaping Sustainable Farming Practices,” International Journal of Computer Engineering in Research Trends, vol. 11, no. 4, pp. 12-19, 2024.
[4] Maria Teresa Linaza et al., “Data-Driven Artificial Intelligence Applications for Sustainable Precision Agriculture,” Agronomy, vol. 11, no. 6, pp. 12-27, 2021.
[CrossRef] [Google Scholar] [Publisher Link]
[5] Kenny Paul et al., “Viable Smart Sensors and their Application in Data-Driven Agriculture,” Computers and Electronics in Agriculture, vol. 198, pp. 107096, 2022.
[CrossRef] [Google Scholar] [Publisher Link]
[6] Sachin S. Kamble, Angappa Gunasekaran, and Shradha A. Gawankar, “Achieving Sustainable Performance in a Data-Driven Agriculture Supply Chain: A Review for Research and Applications,” International Journal of Production Economics, vol. 219, pp. 179-194, 2020.
[CrossRef] [Google Scholar] [Publisher Link]
[7] Mohamed Hamada, and Al-Fayadh A, “Wavelet-Aided Selective Encoding for Enhanced Lossless Image Compression,” Frontiers in Collaborative Research, vol. 1, no. 2, pp. 1-9, 2023.
[Publisher Link]
[8] Kusmec, Aaron et al., “Interdisciplinary Strategies to Enable Data-Driven Plant Breeding in a Changing Climate,” One Earth, vol. 4, no. 3, pp. 372-383, 2021.
[CrossRef] [Google Scholar] [Publisher Link]
[9] Rayikanti Anasurya, “Next-Gen Agriculture: Revolutionizing Farming with IoT and Sustainability,” International Journal of Computer Engineering in Research Trends, vol. 9, no. 1, pp. 21-27, 2022.
[Google Scholar] [Publisher Link]
[10] Nyakuri Jean Pierre et al., “IoT and AI-based Smart Soil Quality Assessment for Data-Driven Irrigation and Fertilization,” American Journal of Computing and Engineering, vol. 5, no. 2, pp. 1-14, 2022.
[CrossRef] [Google Scholar] [Publisher Link]
[11] Ashok Kumar Koshariya et al., “Data-Driven Insights for Agricultural Management: Leveraging Industry 4.0 Technologies for Improved Crop Yields and Resource Optimization,” Robotics and Automation in Industry 4.0, CRC Press, pp. 260-274, 2024.
[Google Scholar] [Publisher Link]
[12] B. Vikranth, Rajeev Wankar, and C. Raghavendra Rao, “Topology Aware Task Stealing for On-Chip NUMA Multi-Core Processors,” Procedia Computer Science, vol. 18, pp. 379-388, 2013.
[CrossRef] [Google Scholar] [Publisher Link]
[13] B. Phaneendra Babu et al., “Fault Diagnosis in Multi-Level Inverter System Using Adaptive Back Propagation Neural Network,” Proceeding of 2008 Annual IEEE India Conference, vol. 2, pp. 494-498, 2008.
[CrossRef] [Google Scholar] [Publisher Link]
[14] Ch. Sarada, V. Dattatreya, and K.Vijaya Lakshmi, “Deep Learning Based Breast Image Classification Study for Cancer Detection,” Proceedings of the 2023 IEEE International Conference on Integrated Circuits and Communication Systems (ICICACS), pp. 01-08, 2023.
[CrossRef] [Google Scholar] [Publisher Link]
[15] Rockstroma J, Barron J, and Addepalli Lavanya, “Aquatic-Based Optimization Techniques for Sustainable Agricultural Development,” Frontiers in Collaborative Research, vol. 1, no. 1, pp. 12-21, 2023.
[Google Scholar] [Publisher Link]
[16] V. Dattatreya, K.V. Chalapati Rao, and M. Raghava, “Smart Sprint Methodology for Financial Management System Development,” Proceedings of the International Conference Advanced Computer Intelligence & Informatics, Springer, pp. 397-411, 2019.
[Google Scholar] [Publisher Link]
[17] Namita M. Butale, and Dattatraya V. Kodavade., “Survey Paper on Detection of Unhealthy Region of Plant Leaves Using Image Processing and Soft Computing Techniques,” International Journal of Computer Engineering in Research Trends, vol. 5, no. 12, pp. 232-235, 2018.
[Publisher Link]
[18] M Sri Lakshmi et al., “Evaluating the Isolation Forest Method for Anomaly Detection in Software-Defined Networking Security,” Journal of Electrical Systems, vol. 19, no. 4, pp. 279-297, 2023.
[CrossRef] [Google Scholar] [Publisher Link]
[19] A. Mallareddy, R. Sridevi, and Ch. G.V.N. Prasad, “Enhanced P-gene Based Data Hiding for Data Security in the Cloud,” International Journal of Recent Technology and Engineering, vol. 8, no. 1, pp. 2086-2093, 2019.
[Google Scholar] [Publisher Link]
[20] Ch. G. V. N. Prasad et al., “Edge Computing and Blockchain in Smart Agriculture Systems,” International Journal on Recent and Innovation Trends in Computing and Communication, vol. 10, no. 1, pp. 265-274, 2022.
[Google Scholar] [Publisher Link]
[21] S. Mekala et al., “EASND: Energy Adaptive Secure Neighbour Discovery Scheme for Wireless Sensor Networks,” International Journal on Recent and Innovation Trends in Computing and Communication, vol. 11, no. 5s, pp. 446-458, 2023.
[Google Scholar] [Publisher Link]
[22] M.Jahir Pasha et al., “LRDADF: An AI-Enabled Framework for Detecting Low-Rate DDoS Attacks in Cloud Computing Environments,” Measurement: Sensors, vol. 28, pp. 100828, 2023.
[CrossRef] [Google Scholar] [Publisher Link]
[23] Sagar Mekala et al., “Machine Learning and Fuzzy Logic Based Intelligent Algorithm for Energy Efficient Routing in Wireless Sensor Networks,” Proceedings of the International Conference on Multidisciplinary Trends and Artificial Intelligence, Springer, 2023, pp. 523-533.
[Google Scholar] [Publisher Link]
[24] Sha Huang et al., “A Commentary Review on the use of Normalized Difference Vegetation Index (NDVI) in the Era of Popular Remote Sensing,” Journal of Forestry Research, vol. 32, no. 1, pp. 1-6, 2021.
[CrossRef] [Google Scholar] [Publisher Link]
[25] Jaibir Singh et al., “Enhancing Cloud Data Privacy with a Scalable Hybrid Approach: HE-DP-SMC,” Journal of Electrical Systems, vol. 19, no. 4, pp. 350-375, 2023.
[CrossRef] [Google Scholar] [Publisher Link]
[26] Birger Larsen et al., “NebulaSafeguard Prototypes: Advancing Information Integrity through Unified Decentralized Verification Schemes,” International Journal of Computer Engineering in Research Trends, vol. 11, no. 1, pp. 43-50, 2024.
[Google Scholar] [Publisher Link]