A Unified Framework for Detecting Gradual and Abrupt Concept Drifts in Data Stream Mining: The Concept Drift Detection Framework with Hybrid Meta-Learning (CDDF-HML)
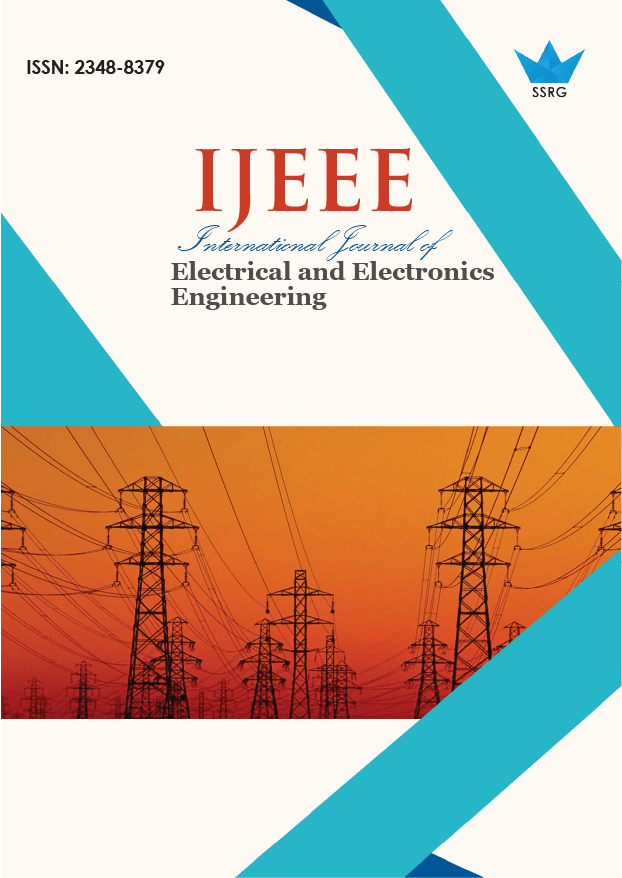
International Journal of Electrical and Electronics Engineering |
© 2024 by SSRG - IJEEE Journal |
Volume 11 Issue 7 |
Year of Publication : 2024 |
Authors : Gollanapalli V. Prasad, Kapil Sharma |
How to Cite?
Gollanapalli V. Prasad, Kapil Sharma, "A Unified Framework for Detecting Gradual and Abrupt Concept Drifts in Data Stream Mining: The Concept Drift Detection Framework with Hybrid Meta-Learning (CDDF-HML)," SSRG International Journal of Electrical and Electronics Engineering, vol. 11, no. 7, pp. 39-50, 2024. Crossref, https://doi.org/10.14445/23488379/IJEEE-V11I7P103
Abstract:
The dynamic structure of data streams provides major challenges for sustaining prediction model accuracy over time. Concept drift, defined as changes in underlying data distributions, has been proven to have a considerable impact on the performance of machine learning models in real-time applications. While earlier methods often focus on either slow or abrupt concept drifts, a unified framework capable of identifying both types quickly is absent. As a result, to overcome the issue mentioned above, we propose the Concept Drift Detection Framework with Hybrid Meta-Learning, abbreviated as CDDF-HML. This incandescent method applies meta-learning, adaptive feature selection and ensemble-based process to address both slow as well as sudden concept drifts. Due to this, the framework is most appropriate in dynamic data stream mining, where the underlying structure is continually changing. It showcases how it can identify deviations of ideas with further capability in accommodating various data conditions. The study also performs the comparative analysis with other techniques to demonstrate that CDDF-HML is really an effective tool for discovering concept drift. The future possibilities of CDDF-HML include the implementation of the method within specific domains, further development of granular adjustment approaches, structural and extensional amendments to scalability, and partnerships with professionals from various industries. It is beneficial in the improvement of the concept drift detection in data stream mining so that the reliability of the model can be assured in dynamic data situations.
Keywords:
Concept drift, Data stream mining, Machine learning, Meta-learning, Adaptive feature selection, Ensemble learning, Real-time monitoring, Gradual drift and Rapid drift, as well as the term framework.
References:
[1] T. Ryan Hoens, Robi Polikar, and Nitesh V. Chawla, “Learning from Streaming Data with Concept Drift and Imbalance: An Overview,” Progress in Artificial Intelligence, vol. 1, pp. 89-101, 2012.
[CrossRef] [Google Scholar] [Publisher Link]
[2] Zeki Murat Çınar et al., “Machine Learning in Predictive Maintenance Towards Sustainable Smart Manufacturing in Industry 4.0,” Sustainability, vol. 12, no. 19, pp. 1-42, 2020.
[CrossRef] [Google Scholar] [Publisher Link]
[3] Gregory Ditzler, and Robi Polikar, “Incremental Learning of Concept Drifts from Streaming Imbalanced Data,” IEEE Transactions on Knowledge and Data Engineering, vol. 25, no. 10, pp. 2283-2301, 2013.
[CrossRef] [Google Scholar] [Publisher Link]
[4] Tabssum Khan, Arkan Ahmed Hussein, and Ahmad M. Hussein Shabani, “StreamDrift: A Unified Model for Detecting Gradual and Sudden Changes in Data Streams,” International Journal of Computer Engineering in Research Trends, vol. 11, no. 5, pp. 58-64, 2024.
[CrossRef] [Publisher Link]
[5] Paolo Dini, Mykola Makhortykh, and Maryna Sydorova, “DataStreamAdapt: Unified Detection Framework for Gradual and Abrupt Concept Drifts,” Synthesis: A Multidisciplinary Research Journal, vol. 1, no. 4, pp. 1-9, 2023.
[Google Scholar] [Publisher Link]
[6] Oleksii Tsepa, and Mir Mohsen Pedram, “ShiftSense: A Unified Framework for Comprehensive Detection of Gradual and Abrupt Concept Shifts in Streaming Data,” Frontiers in Collaborative Research, vol. 1, no. 4, pp. 1-9, 2023.
[Google Scholar] [Publisher Link]
[7] Pedro Domingos, and Geoff Hulten, “Mining High-Speed Data Streams,” Proceedings of the Sixth ACM SIGKDD International Conference on Knowledge Discovery and Data Mining, pp. 71-80, 2000.
[Google Scholar] [Publisher Link]
[8] Anh Vu Luong et al., “Heterogeneous Ensemble Selection for Evolving Data Streams,” Pattern Recognition, vol. 112, 2021.
[CrossRef] [Google Scholar] [Publisher Link]
[9] Heitor M. Gomes et al., “Adaptive Random Forests for Evolving Data Stream Classification,” Machine Learning, vol. 106, pp. 1469- 1495, 2017.
[CrossRef] [Google Scholar] [Publisher Link]
[10] Hammam Abdelwahab, “Evaluation of Drift Detection Techniques for Automated Machine Learning Pipelines,” Master Thesis, Fraunhofer-Gesellschaft, 2022.
[CrossRef] [Google Scholar] [Publisher Link]
[11] Yange Sun et al., “An Online Ensemble Using Adaptive Windowing for Data Streams with Concept Drift,” International Journal of Distributed Sensor Networks, vol. 12, no. 5, 2016.
[CrossRef] [Google Scholar] [Publisher Link]
[12] Elhadj Benkhelifa, Lokhande Gaurav, and Vidya Sagar S.D., “BioShieldNet: Advanced Biologically Inspired Mechanisms for Strengthening Cybersecurity in Distributed Computing Environments,” International Journal of Computer Engineering in Research Trends, vol. 11, no. 3, pp. 1-9, 2024.
[CrossRef] [Publisher Link]
[13] Jianjun Chen et al., “A Meta-Learning Method for Electric Machine Bearing Fault Diagnosis under Varying Working Conditions with Limited Data,” IEEE Transactions on Industrial Informatics, vol. 19, no. 3, pp. 2552-2564, 2023.
[CrossRef] [Google Scholar] [Publisher Link]
[14] Kritika, “A Deep Dive into Code Smell and Vulnerability Using Machine Learning and Deep Learning Techniques,” International Journal of Computer Engineering in Research Trends, vol. 11, no. 4, pp. 32-45, 2024.
[CrossRef] [Google Scholar] [Publisher Link]
[15] Thomas N. Rincy, and Roopam Gupta, “Ensemble Learning Techniques and its Efficiency in Machine Learning: A Survey,” 2 nd International Conference on Data, Engineering and Applications (IDEA), pp. 1-6, 2020.
[CrossRef] [Google Scholar] [Publisher Link]
[16] Lokhande Gaurav, Maloth Bhavsingh, and Jaime Lloret, “QuantumShield Framework: Pioneering Resilient Security in IoT Networks Through Quantum-Resistant Cryptography and Federated Learning Techniques,” International Journal of Computer Engineering in Research Trends, vol. 11, no. 1, pp. 61-69, 2024.
[Publisher Link]
[17] Ning Lu, Guangquan Zhang, and Jie Lu, “Concept Drift Detection via Competence Models,” Artificial Intelligence, vol. 209, pp. 11-28, 2014.
[CrossRef] [Google Scholar] [Publisher Link]
[18] Jie Lu et al., “Learning under Concept Drift: A Review,” IEEE Transactions on Knowledge and Data Engineering, vol. 31, no. 12, pp. 2346-2363, 2018.
[CrossRef] [Google Scholar] [Publisher Link]
[19] Azlinah Mohamed et al., “The State of the Art and Taxonomy of Big Data Analytics: View from New Big Data Framework,” Artificial Intelligence Review, vol. 53, pp. 989-1037, 2020.
[CrossRef] [Google Scholar] [Publisher Link]
[20] Birger Larsen et al., “NebulaSafeguard Prototypes: Advancing Information Integrity through Unified Decentralized Verification Schemes,” International Journal of Computer Engineering in Research Trends, vol. 11, no. 1, pp. 43-54, 2024.
[CrossRef] [Publisher Link]
[21] M. Sunitha et al., “Ascertaining along with the Taxonomy of Vegetation Folio Ailment Employing CNN Besides LVQ Algorithm,” International Journal on Recent and Innovation Trends in Computing and Communication, vol. 11, no. 6, pp. 113-117, 2023.
[CrossRef] [Publisher Link]
[22] Kavitha Soppari, and N. Subhash Chandra, “Automated Digital Image Watermarking Based on Multi-Objective Hybrid Meta-Heuristic-Based Clustering Approach,” International Journal of Intelligent Robotics and Applications, vol. 7, no. 1, 2022.
[CrossRef] [Google Scholar] [Publisher Link]
[23] Vijaykrishnan Narayanan, and Kevin W. Eliceiri, “Deep Wavelet Packet Decomposition with Adaptive Entropy Modeling for Selective Lossless Image Compression,” Synthesis: A Multidisciplinary Research Journal, vol. 1, no. 1, pp. 1-10, 2023.
[Google Scholar] [Publisher Link]
[24] K. Samunnisa, and Sunil Vijaya Kumar Gaddam, “Blockchain-Based Decentralized Identity Management for Secure Digital Transactions,” Synthesis: A Multidisciplinary Research Journal, vol. 1, no. 2, pp. 22-29, 2023.
[Publisher Link]