Automated Monitoring of Gym Exercises through Human Pose Analysis
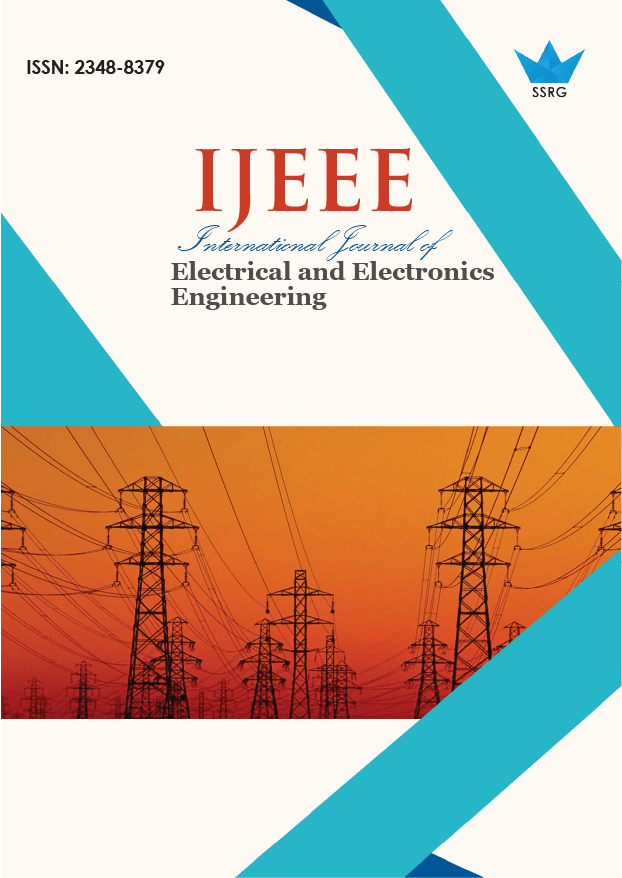
International Journal of Electrical and Electronics Engineering |
© 2024 by SSRG - IJEEE Journal |
Volume 11 Issue 7 |
Year of Publication : 2024 |
Authors : Ajitkumar Shitole, Mahesh Gaikwad, Prathamesh Bhise, Yash Dusane, Pranav Gaikwad |
How to Cite?
Ajitkumar Shitole, Mahesh Gaikwad, Prathamesh Bhise, Yash Dusane, Pranav Gaikwad, "Automated Monitoring of Gym Exercises through Human Pose Analysis," SSRG International Journal of Electrical and Electronics Engineering, vol. 11, no. 7, pp. 57-65, 2024. Crossref, https://doi.org/10.14445/23488379/IJEEE-V11I7P105
Abstract:
This study offers a game-changing approach that combines techniques and exercises to ensure accuracy and injuryfree performance in all activities, including squats and push-ups. The main goal is to teach proper structure and body posture to reduce injuries while keeping the body strong and having fun. The preparation process has an easy-to-use interface that makes repetitions and exercise preparation tangible. This is much newer than fitness tracking. Consider a system that tracks reps and provides quick feedback as you perform the exercise. Teach users to maintain good posture and reduce the risk of injury by receiving real-time alerts. This work explores the complexities of self-tracking, push alerts and reports, turning every activity into an efficient and mindful one. With 20 different exercises targeting different muscle areas and representative groups, the system will get even better with future mode notifications. To improve performance and safety, the module will give an alert on the screen if the user’s body is faulty. The device uses powerful machine learning algorithms and powerful camera-based prediction technology to provide users with instant feedback on poses and techniques. The proposed platform has the potential to transform the energy industry by changing behavior and improving the safety and effectiveness of exercise. It is a useful tool for anyone who wants to improve their body and health.
Keywords:
Fitness technology, Personalized workouts, Real-time feedback, Social motivation, Fitness companion.
References:
[1] Jinbao Wang et al., “Deep 3D Human Pose Estimation: A Review,” Computer Vision and Image Understanding, vol. 210, pp. 1-21, 2021.
[CrossRef] [Google Scholar] [Publisher Link]
[2] Alessia Bertugli et al., “AC-VRNN: Attentive Conditional-VRNN for Multi-Future Trajectory Prediction,” Computer Vision and Image Understanding, vol. 210, pp. 1-10, 2021.
[CrossRef] [Google Scholar] [Publisher Link]
[3] Torsten Schlett, Christian Rathgeb, and Christoph Busch, “Deep Learning-Based Single Image Face Depth Data Enhancement,” Computer Vision and Image Understanding, vol. 210, pp. 1-15, 2021.
[CrossRef] [Google Scholar] [Publisher Link]
[4] Muhammed Kocabas, Salih Karagoz, and Emre Akbas, “Self-Supervised Learning of 3D Human Pose Using Multi-View Geometry,” 2019 IEEE/CVF Conference on Computer Vision and Pattern Recognition (CVPR), Long Beach, USA, pp. 1077-1086, 2019.
[CrossRef] [Google Scholar] [Publisher Link]
[5] Tianlang Chen et al., “Anatomy-Aware 3D Human Pose Estimation with Bone-Based Pose Decomposition,” IEEE Transactions on Circuits and Systems for Video Technology, vol. 32, no. 1, pp. 198-209, 2022.
[CrossRef] [Google Scholar] [Publisher Link]
[6] Amir Nadeem, Ahmad Jalal, and Kibum Kim, “Human Actions Tracking and Recognition Based on Body Parts Detection via Artificial Neural Network,” 2020 3rd International Conference on Advancements in Computational Sciences (ICACS), Lahore, Pakistan, pp. 1-6, 2020.
[CrossRef] [Google Scholar] [Publisher Link]
[7] Ankit Verma et al., “Web Application Implementation with Machine Learning,” 2021 2nd International Conference on Intelligent Engineering and Management (ICIEM), London, United Kingdom, pp. 423-428, 2021.
[CrossRef] [Google Scholar] [Publisher Link]
[8] Dario Pavllo et al., “3D Human Pose Estimation in Video with Temporal Convolutions and Semi-Supervised Training,” 2019 IEEE/CVF Conference on Computer Vision and Pattern Recognition (CVPR), Long Beach, USA, pp. 7745-7754, 2019.
[CrossRef] [Google Scholar] [Publisher Link]
[9] Liqian Ma et al., “Direct Dense Pose Estimation,” 2021 International Conference on 3D Vision (3DV), London, United Kingdom, pp. 721-730, 2021.
[CrossRef] [Google Scholar] [Publisher Link]
[10] Shuangjun Liu, Naveen Sehgal, and Sarah Ostadabbas, “Adapted Human Pose: Monocular 3D Human Pose Estimation with Zero Real 3D Pose Data,” Applied Intelligence, vol. 52, pp. 14491-14506, 2022.
[CrossRef] [Google Scholar] [Publisher Link]
[11] Bastian Wandt, and Bodo Rosenhahn, “Repnet: Weakly Supervised Training of an Adversarial Reprojection Network for 3D Human Pose Estimation,” 2019 IEEE/CVF Conference on Computer Vision and Pattern Recognition (CVPR), Long Beach, USA, pp. 7774-7783, 2019.
[CrossRef] [Google Scholar] [Publisher Link]
[12] Zhe Cao et al., “Openpose: Realtime Multi-Person 2D Pose Estimation Using Part Affinity Fields,” IEEE Transactions on Pattern Analysis and Machine Intelligence, vol. 43, no. 1, pp. 172-186, 2021.
[CrossRef] [Google Scholar] [Publisher Link]
[13] Mia Kokic, Danica Kragic, and Jeannette Bohg, “Learning to Estimate Pose and Shape of Hand-Held Objects from RGB Images,” 2019 IEEE/RSJ International Conference on Intelligent Robots and Systems (IROS), Macau, China, pp. 3980-3987, 2019.
[CrossRef] [Google Scholar] [Publisher Link]
[14] Yana Hasson et al., “Learning Joint Reconstruction of Hands and Manipulated Objects,” 2019 IEEE/CVF Conference on Computer Vision and Pattern Recognition (CVPR), Long Beach, USA, pp. 11799-11808, 2019.
[CrossRef] [Google Scholar] [Publisher Link]
[15] Arshad Javeed, “Performance Optimization Techniques for ReactJS,” 2019 IEEE International Conference on Electrical, Computer and Communication Technologies (ICECCT), Coimbatore, India, pp. 1-5, 2019.
[CrossRef] [Google Scholar] [Publisher Link]
[16] Domènech López Antonio, “Sign Language Recognition ASL Recognition with MediaPipe and Recurrent Neural Networks,” Bachelor Thesis, FH Aachen University of Applied Sciences, 2020.
[Google Scholar] [Publisher Link]
[17] Gerges H. Samaan et al., “MediaPipe’s Landmarks with RNN for Dynamic Sign Language Recognition,” Electronics, vol. 11, no. 19, 2022.
[CrossRef] [Google Scholar] [Publisher Link]
[18] Rupesh Kumar, Ashutosh Bajpai, and Ayush Sinha, “Mediapipe and CNNs for Real-Time ASL Gesture Recognition,” arXiv, pp. 1-5, 2023.
[CrossRef] [Google Scholar] [Publisher Link]
[19] Long Zhao et al., “Semantic Graph Convolutional Networks for 3D Human Pose Regression,” 2019 IEEE/CVF Conference on Computer Vision and Pattern Recognition (CVPR), Long Beach, CA, USA, pp. 3420-3430, 2019.
[CrossRef] [Google Scholar] [Publisher Link]
[20] Yuanlu Xu, Song-Chun Zhu, and Tony Tung, “DenseRaC: Joint 3D Pose and Shape Estimation by Dense Render-and-Compare,” 2019 IEEE/CVF International Conference on Computer Vision (ICCV), Seoul, Korea (South), pp. 7759-7769, 2019.
[CrossRef] [Google Scholar] [Publisher Link]
[21] Yujun Cai et al., “Exploiting Spatial-Temporal Relationships for 3D Pose Estimation via Graph Convolutional Networks,” 2019 IEEE/CVF International Conference on Computer Vision (ICCV), Seoul, Korea (South), pp. 2272-2281, 2019.
[CrossRef] [Google Scholar] [Publisher Link]