A Novel Hybridized Optimization Mechanism for Efficient Channel Estimation Using Adaptive and Attention-Based Convolutional Autoencoder in MIMO-NOMA for mm Wave Systems
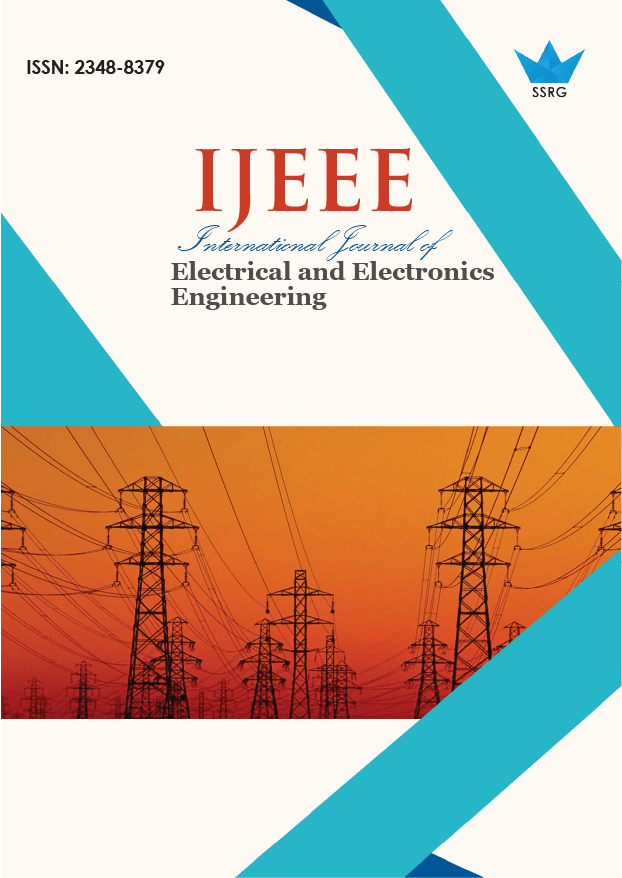
International Journal of Electrical and Electronics Engineering |
© 2024 by SSRG - IJEEE Journal |
Volume 11 Issue 7 |
Year of Publication : 2024 |
Authors : Belcy D. Mathews, Tamilarasi Muthu |
How to Cite?
Belcy D. Mathews, Tamilarasi Muthu, "A Novel Hybridized Optimization Mechanism for Efficient Channel Estimation Using Adaptive and Attention-Based Convolutional Autoencoder in MIMO-NOMA for mm Wave Systems," SSRG International Journal of Electrical and Electronics Engineering, vol. 11, no. 7, pp. 78-101, 2024. Crossref, https://doi.org/10.14445/23488379/IJEEE-V11I7P107
Abstract:
The channel estimation is crucial in the “millimeter Wave (mmWave) Massive Multiple-Input Multiple-Output (MIMO) and Non-Orthogonal Multiple Access (NOMA)” devices. Hybrid beamforming techniques are employed nowadays to minimize the complexity and equipment price. However, the absence of digital beam forming in mmWave affects the dynamic range and accuracy of the channel estimation. Previous research is concentrated mainly on predicting narrow-band mmWave channels using deep learning networks as the wideband channels of mmWave create a considerable amount of range and noise issues. Accurate channel estimation in the MIMO system is challenging because of the increased number of antennas and RadioFrequency (RF) chains. MIMO system communications using mmWave are frequently chosen because of their massive spectrum resources. Therefore, it is essential to tackle the obstacles obtained in the standard channel estimation framework by developing a MIMO-NOMA network with the help of deep learning methods. In this paper, an advanced tuning and prediction approach with a deep learning mechanism is designed to perform an accurate estimation of channels for the MIMO-NOMA system. Moreover, a hybridized optimization model called Wild Horse-Piranha Foraging Optimization Algorithm (WH-PFOA) is developed and utilized with Adaptive and Attention-based Convolutional Autoencoder (Ada-ACAE) for estimating the channels in mmWave-based MIMO-NOMA system. Furthermore, the complexity rate and the hardware cost of the MIMO-NOMA network are reduced by adapting the hybrid beam-forming mechanism. Initially, to perform channel estimation, the pilot symbols are tuned by the introduced WH-PFOA to enhance the channel estimation performance. Later, the channel estimation is carried out with the optimal pilot symbols and the channel coefficients are validated. Numerical results show that the proposed channel estimation and pilot estimation process outperforms the state-of-the-art approaches.
Keywords:
Millimeter wave network, Massive Multiple-Input Multiple-Output, Non-Orthogonal Multiple Access, Attentionbased convolutional autoencoder, Wild horse-piranha foraging optimization algorithm.
References:
[1] Bingtong Xiang, Die Hu, and Jun Wu, “Deep Learning-Based Downlink Channel Estimation for FDD Massive MIMO Systems,” IEEE Wireless Communications Letters, vol. 12, no. 4, pp. 699-702, 2023.
[CrossRef] [Google Scholar] [Publisher Link]
[2] Chang-Jae Chun, Jae-Mo Kang, and Il-Min Kim, “Deep Learning-Based Channel Estimation for Massive MIMO Systems,” IEEE Wireless Communications Letters, vol. 8, no. 4, pp. 1228-1231, 2019.
[CrossRef] [Google Scholar] [Publisher Link]
[3] Byungju Lim et al., “Joint Pilot Design and Channel Estimation Using Deep Residual Learning for Multi-Cell Massive MIMO Under Hardware Impairments,” IEEE Transactions on Vehicular Technology, vol. 71, no. 7, pp. 7599-7612, 2022.
[CrossRef] [Google Scholar] [Publisher Link]
[4] Mehran Soltani, Vahid Pourahmadi, and Hamid Sheikhzadeh, “Pilot Pattern Design for Deep Learning-Based Channel Estimation in OFDM Systems,” IEEE Wireless Communications Letters, vol. 9, no. 12, pp. 2173-2176, 2020.
[CrossRef] [Google Scholar] [Publisher Link]
[5] Chang-Jae Chun, Jae-Mo Kang, and Il-Min Kim, “Deep Learning-Based Joint Pilot Design and Channel Estimation for Multiuser MIMO Channels,” IEEE Communications Letters, vol. 23, no. 11, pp. 1999-2003, 2019.
[CrossRef] [Google Scholar] [Publisher Link]
[6] Liangtian Wan, Kaihui Liu, and Wei Zhang, “Deep Learning-Aided Off-Grid Channel Estimation for Millimeter Wave Cellular Systems,” IEEE Transactions on Wireless Communications, vol. 21, no. 5, pp. 3333-3348, 2022.
[CrossRef] [Google Scholar] [Publisher Link]
[7] Marius Arvinte, and Jonathan I. Tamir, “MIMO Channel Estimation Using Score-Based Generative Models,” IEEE Transactions on Wireless Communications, vol. 22, no. 6, pp. 3698-3713, 2023.
[CrossRef] [Google Scholar] [Publisher Link]
[8] Ahmet Emir et al., “Deep Learning Empowered Semi-Blind Joint Detection in Cooperative NOMA,” IEEE Access, vol. 9, pp. 61832- 61852, 2021.
[CrossRef] [Google Scholar] [Publisher Link]
[9] Chi Nguyen, Tiep M. Hoang, and Adnan A. Cheema, “Channel Estimation Using CNN-LSTM in RIS-NOMA Assisted 6G Network,” IEEE Transactions on Machine Learning in Communications and Networking, vol. 1, pp. 43-60, 2023.
[CrossRef] [Google Scholar] [Publisher Link]
[10] Md. Habibur Rahman et al., “HyDNN: A Hybrid Deep Learning Framework Based Multiuser Uplink Channel Estimation and Signal Detection for NOMA-OFDM System,” IEEE Access, vol. 11, pp. 66742-66755, 2023.
[CrossRef] [Google Scholar] [Publisher Link]
[11] Xiaoyi Zhu et al., “A Deep Learning and Geospatial Data-Based Channel Estimation Technique for Hybrid Massive MIMO Systems,” IEEE Access, vol. 9, pp. 145115-145132, 2021.
[CrossRef] [Google Scholar] [Publisher Link]
[12] Y.G. Li et al., “MIMO-OFDM for Wireless Communications: Signal Detection with Enhanced Channel Estimation,” IEEE Transactions on Communications, vol. 50, no. 9, pp. 1471-1477, 2002.
[CrossRef] [Google Scholar] [Publisher Link]
[13] Yiqing Zhang et al., “Deep Expectation-Maximization for Joint MIMO Channel Estimation and Signal Detection,” IEEE Transactions on Signal Processing, vol. 70, pp. 4483-4497, 2022.
[CrossRef] [Google Scholar] [Publisher Link]
[14] Marcello Cicerone, Osvaldo Simeone, and Umberto Spagnolini, “Channel Estimation for MIMO-OFDM Systems by Modal Analysis/Filtering,” IEEE Transactions on Communications, vol. 54, no. 11, pp. 2062-2074, 2006.
[CrossRef] [Google Scholar] [Publisher Link]
[15] Muhammad Umer Zia et al., “Deep Learning for Parametric Channel Estimation in Massive MIMO Systems,” IEEE Transactions on Vehicular Technology, vol. 72, no. 4, pp. 4157-4167, 2023.
[CrossRef] [Google Scholar] [Publisher Link]
[16] Hui-Ping Yin et al., “Deep-Learning-Based Channel Estimation for Chaotic Wireless Communication,” IEEE Wireless Communications Letters, vol. 13, no. 1, pp. 143-147, 2024.
[CrossRef] [Google Scholar] [Publisher Link]
[17] Jae-Mo Kang, Chang-Jae Chun, and Il-Min Kim, “Deep Learning Based Channel Estimation for MIMO Systems with Received SNR Feedback,” IEEE Access, vol. 8, pp. 121162-121181, 2020.
[CrossRef] [Google Scholar] [Publisher Link]
[18] Aihua Zhang et al., “Channel Estimation for MmWave Massive MIMO with Hybrid Precoding Based on Log-Sum Sparse Constraints,” IEEE Transactions on Circuits and Systems II: Express Briefs, vol. 68, no. 6, pp. 1882-1886, 2021.
[CrossRef] [Google Scholar] [Publisher Link]
[19] In-Soo Kim, and Junil Choi, “FCFGS-CV-Based Channel Estimation for Wideband mmWave Massive MIMO Systems With Low-Resolution ADCs,” IEEE Wireless Communications Letters, vol. 8, no. 6, pp. 1648-1652, 2019.
[CrossRef] [Google Scholar] [Publisher Link]
[20] Rui Zhang et al., “Channel Estimation for mmWave Massive MIMO Systems with Mixed-ADC Architecture,” IEEE Open Journal of the Communications Society, vol. 4, pp. 606-613, 2023.
[CrossRef] [Google Scholar] [Publisher Link]
[21] Muneeb Ahmad, and Soo Young Shin, “Wavelet-Based Massive MIMO-NOMA with Advanced Channel Estimation and Detection Powered by Deep Learning,” Physical Communication, vol. 61, 2023.
[CrossRef] [Google Scholar] [Publisher Link]
[22] Zhen Chen et al., “Hybrid Evolutionary-Based Sparse Channel Estimation for IRS-Assisted mmWave MIMO Systems,” IEEE Transactions on Wireless Communications, vol. 21, no. 3, pp. 1586-1601, 2022.
[CrossRef] [Google Scholar] [Publisher Link]
[23] Hengtao He et al., “Deep Learning-Based Channel Estimation for Beamspace mmWave Massive MIMO Systems,” IEEE Wireless Communications Letters, vol. 7, no. 5, pp. 852-855, 2018.
[CrossRef] [Google Scholar] [Publisher Link]
[24] Asmaa Abdallah et al., “Deep Learning-Based Frequency-Selective Channel Estimation for Hybrid mmWave MIMO Systems,” IEEE Transactions on Wireless Communications, vol. 21, no. 6, pp. 3804-3821, 2022.
[CrossRef] [Google Scholar] [Publisher Link]
[25] Xisuo Ma et al., “Model-Driven Deep Learning Based Channel Estimation and Feedback for Millimeter-Wave Massive Hybrid MIMO Systems,” IEEE Journal on Selected Areas in Communications, vol. 39, no. 8, pp. 2388-2406, 2021.
[CrossRef] [Google Scholar] [Publisher Link]
[26] Iraj Naruei, and Farshid Keynia, “Wild Horse Optimizer: A New Meta-Heuristic Algorithm for Solving Engineering Optimization Problems,” Engineering with Computers, vol. 38, pp. 3025-3056, 2022.
[CrossRef] [Google Scholar] [Publisher Link]
[27] Shuai Cao et al., “A Novel Meta-Heuristic Algorithm for Numerical and Engineering Optimization Problems: Piranha Foraging Optimization Algorithm (PFOA),” IEEE Access, vol. 11, pp. 92505-92522, 2023.
[CrossRef] [Google Scholar] [Publisher Link]
[28] B.M.R. Manasa et al., “A Novel Channel Estimation Framework in MIMO Using Serial Cascaded Multiscale Autoencoder and Attention LSTM with Hybrid Heuristic Algorithm,” Sensors, vol. 23, no. 22, pp. 1-27, 2023.
[CrossRef] [Google Scholar] [Publisher Link]
[29] Eva Trojovska, and Mohammad Dehghani, “Clouded Leopard Optimization: A New Nature-Inspired Optimization Algorithm,” IEEE Access, vol. 10, pp. 102876-102906, 2022.
[CrossRef] [Google Scholar] [Publisher Link]
[30] Muxuan Han et al., “Walrus Optimizer: A Novel Nature-Inspired Metaheuristic Algorithm,” Expert Systems with Applications, vol. 239, 2024.
[CrossRef] [Google Scholar] [Publisher Link]