Early Detection of Alzheimer’s Disease: Leveraging Biomarker from FDG-PET Using Weighted SVM Clustering
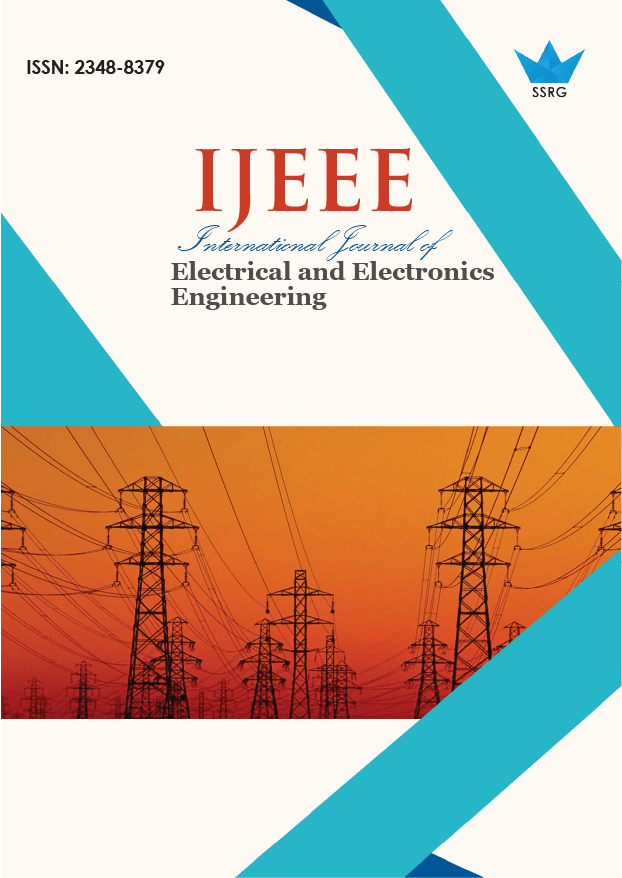
International Journal of Electrical and Electronics Engineering |
© 2024 by SSRG - IJEEE Journal |
Volume 11 Issue 7 |
Year of Publication : 2024 |
Authors : Chintan R. Varnagar, Nirali N. Madhak, Prashant D. Maheta, Sanjay D. Bhanderi, Haresh M. Rathod |
How to Cite?
Chintan R. Varnagar, Nirali N. Madhak, Prashant D. Maheta, Sanjay D. Bhanderi, Haresh M. Rathod, "Early Detection of Alzheimer’s Disease: Leveraging Biomarker from FDG-PET Using Weighted SVM Clustering," SSRG International Journal of Electrical and Electronics Engineering, vol. 11, no. 7, pp. 185-198, 2024. Crossref, https://doi.org/10.14445/23488379/IJEEE-V11I7P116
Abstract:
Alzheimer’s Disease (AD) is a progressive neurodegenerative disorder marked by cognitive decline, memory loss, and impaired reasoning, caused by the accumulation of amyloid-beta plaques and neurofibrillary tangles of tau protein. This study utilizes neuroimaging Fluorodeoxyglucose Positron Emission Tomography (FDG-PET) and structural MRI and clinical data from 1069 subjects acquired through the Alzheimer’s Disease Neuroimaging Initiative (ADNI). Early-stage AD presents challenges for classification between Mild Cognitive Impairment (MCI) and Cognitively Normal (CN) individuals, as structural abnormalities are less pronounced, making sMRI-derived features less discriminative. However, functional connectivity disruptions in brain regions are notable in the early AD stages. This study investigates whether the metabolic brain assessment biomarker, Support Uptake Value Ratio (SUVR) from FDG-PET, can enhance classification performance between MCI and CN classes effectively or not. We introduced a novel weighted random SVM cluster, an ensemble method that outperforms single SVM classifiers by addressing issues of limited data and noise. Using the AAL3 Atlas, which provides finer subdivisions of brain regions (168 regions compared to AAL2’s 90), we achieved a more detailed connectivity analysis. Employing rigorous preprocessing and a Stratified K-Fold Nested Cross Validation with k=5 and an 8:2 train-validation to test split ensured robust hyperparameter tuning and model selection to prevent overfitting and selection bias. The proposed method demonstrates good classification accuracy for MCI vs. CN and exhibits good ROC characteristics, indicating sensitivity to detect disruptive metabolic changes, highlighting its potential in early AD detection.
Keywords:
Alzheimer’s diseases, Quantified biomarkers of AD, Positron Emission Tomography, FDG-PET, Weighted SVM cluster.
References:
[1] Kejal Kantarci et al., “White-Matter Integrity on DTI and the Pathologic Staging of Alzheimer’s Disease,” Neurobiology of Aging, vol. 56, pp. 172-179, 2017.
[CrossRef] [Google Scholar] [Publisher Link]
[2] Alberto Serrano-Pozo et al., “Neuropathological Alterations in Alzheimer Disease,” Cold Spring Harbor Perspectives in Medicine, vol. 1, pp. 1-24, 2011.
[CrossRef] [Google Scholar] [Publisher Link]
[3] Mark W. Bondi, Emily C. Edmonds, and David P. Salmon, “Alzheimer’s Disease: Past, Present, and Future,” Journal of the International Neuropsychological Society, vol. 23, no. 9-10, pp. 818-831, 2017.
[CrossRef] [Google Scholar] [Publisher Link]
[4] Marcos Vinícius Ferreira Silva et al., “Alzheimer’s Disease: Risk Factors and Potentially Protective Measures,” Journal of Biomedical Science, vol. 26, pp. 1-11, 2019.
[CrossRef] [Google Scholar] [Publisher Link]
[5] Marta Crous-Bou et al., “Alzheimer’s Disease Prevention: from Risk Factors to Early Intervention,” Alzheimer’s Research & Therapy, vol. 9, pp. 1-9, 2017.
[CrossRef] [Google Scholar] [Publisher Link]
[6] Elizabeth Joe, and John M. Ringman, “Cognitive Symptoms of Alzheimer’s Disease: Clinical Management and Prevention,” British Medical Journal, vol. 367, 2019.
[CrossRef] [Google Scholar] [Publisher Link]
[7] J. Mendiola-Precoma et al., “Therapies for Prevention and Treatment of Alzheimer’s Disease,” Biomed Research International, vol. 2016, pp. 1-17, 2016.
[CrossRef] [Google Scholar] [Publisher Link]
[8] George Crisan et al., “Radiopharmaceuticals for PET and SPECT Imaging: A Literature Review over the Last Decade,” International Journal of Molecular Sciences, vol. 23, no. 9, pp. 1-52, 2022.
[CrossRef] [Google Scholar] [Publisher Link]
[9] Sandip Basu et al., “The Basic Principles of FDG-PET/CT Imaging,” PET Clinics, vol. 9, no. 4, pp. 355-370, 2014.
[CrossRef] [Google Scholar] [Publisher Link]
[10] Ashwini Prem Kumar et al., “Neuronal PET Tracers for Alzheimer’s Disease,” Biochemical and Biophysical Research Communications, vol. 587, pp. 58-62, 2022.
[CrossRef] [Google Scholar] [Publisher Link]
[11] Lucas Porcello Schilling et al., “Imaging Alzheimer’s Disease Pathophysiology with PET,” Dementia & Neuropsychologia, vol. 10, no. 2, pp. 79-90, 2016.
[CrossRef] [Google Scholar] [Publisher Link]
[12] Charles Marcus, Esther Mena, and Rathan M. Subramaniam, “Brain PET in the Diagnosis of Alzheimer’s Disease,” Clinical Nuclear Medicine, vol. 39, no. 10, pp. e413-e426, 2014.
[CrossRef] [Google Scholar] [Publisher Link]
[13] N. Sadeghi et al., “Automatic Classification of Alzheimer’s Disease vs. Frontotemporal Dementia: A Spatial Decision Tree Approach with FDG-PET,” 2008 5th IEEE International Symposium on Biomedical Imaging: From Nano to Macro, Paris, France, pp. 408-411, 2008.
[CrossRef] [Google Scholar] [Publisher Link]
[14] Silvia Paola Caminiti et al., “FDG-PET and CSF Biomarker Accuracy in Prediction of Conversion to Different Dementias in a Large Multicentre MCI Cohort,” NeuroImage Clinical, vol. 18, pp. 167-177, 2018.
[CrossRef] [Google Scholar] [Publisher Link]
[15] Qing Li et al., “Classification of Alzheimer’s Disease, Mild Cognitive Impairment, and Cognitively Unimpaired Individuals Using Multi-Feature Kernel Discriminant Dictionary Learning,” Frontiers in Computational Neuroscience, vol. 11, pp. 1-14, 2018.
[CrossRef] [Google Scholar] [Publisher Link]
[16] Liqun Kuang et al., “Metabolic Brain Network Analysis of FDG-PET in Alzheimer’s Disease Using Kernel-Based Persistent Features,” Molecules, vol. 24, no. 12, pp. 1-14, 2019.
[CrossRef] [Google Scholar] [Publisher Link]
[17] Sebastian Palmqvist et al., “Detailed Comparison of Amyloid PET and CSF Biomarkers for Identifying Early Alzheimer Disease,” Neurology, vol. 85, no. 14, pp. 1240-1249, 2015.
[CrossRef] [Google Scholar] [Publisher Link]
[18] Bradley T. Wyman et al., “Standardization of Analysis Sets for Reporting Results from ADNI MRI Data,” Alzheimer’s Dementia, vol. 9, no. 3, pp. 332-337, 2013.
[CrossRef] [Google Scholar] [Publisher Link]
[19] Krzysztof J. Gorgolewski et al., “The Brain Imaging Data Structure, A Format for Organizing and Describing Outputs of Neuroimaging Experiments,” Scientific Data, vol. 3, 2016.
[CrossRef] [Google Scholar] [Publisher Link]
[20] Karl J. Friston et al., Statistical Parametric Mapping: The Analysis of Functional Brain Images, 1st ed., Elsevier, 2007.
[Google Scholar] [Publisher Link]
[21] Benjamin A Thomas et al., “PETPVC: A Toolbox for Performing Partial Volume Correction Techniques in Positron Emission Tomography,” Physics in Medicine & Biology, vol. 61, no. 22, 2016.
[CrossRef] [Google Scholar] [Publisher Link]
[22] Andrew Hoopes et al., “SynthStrip: Skull-Stripping for any Brain Image,” NeuroImage, vol. 260, 2022.
[CrossRef] [Google Scholar] [Publisher Link]
[23] Edmund T. Rolls et al., “Automated Anatomical Labelling Atlas 3,” NeuroImage, vol. 206, 2020.
[CrossRef] [Google Scholar] [Publisher Link]
[24] Fabian Pedregosa et al., “Scikit-Learn: Machine Learning in Python,” Journal of Machine Learning Research, vol. 12, pp. 2825-2830, 2011.
[Google Scholar] [Publisher Link]