Demand Side Management Using a Novel NatureInspired Pelican Optimization Algorithm in a Smart Grid Environment
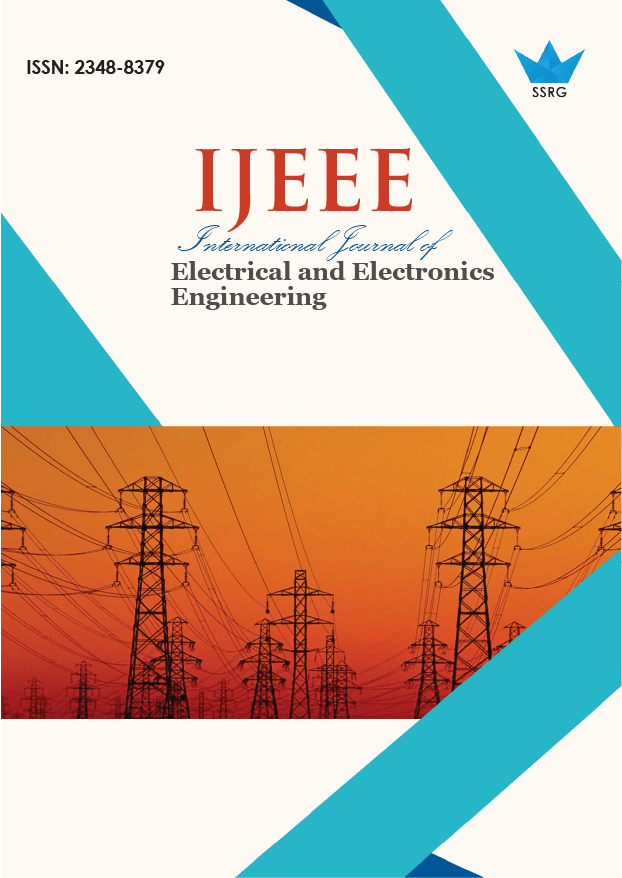
International Journal of Electrical and Electronics Engineering |
© 2024 by SSRG - IJEEE Journal |
Volume 11 Issue 7 |
Year of Publication : 2024 |
Authors : H. Lakshmi |
How to Cite?
H. Lakshmi, "Demand Side Management Using a Novel NatureInspired Pelican Optimization Algorithm in a Smart Grid Environment," SSRG International Journal of Electrical and Electronics Engineering, vol. 11, no. 7, pp. 238-246, 2024. Crossref, https://doi.org/10.14445/23488379/IJEEE-V11I7P121
Abstract:
A global trend that creates many uses for the data generated by dynamic networks is the deployment of smart grids. Converting this enormous transformation of data into information that the electrical system can use is the difficult part. Using Demand-Side Management (DSM) strategies to maximize power system management in real-time is one illustration of this. This article presents the Pelican Optimization Algorithm (POA), a unique optimization technique influenced by its chaotic nature. It provides the DSM with many controlled devices with a load-shifting solution. The loading-shifting issue has been managed hourly through the day in order to minimize the Peak to Average load Ratio (PAR), reduce the cost of power, and lower peak demand. It is suggested to use the POA mathematical model to solve optimization issues. POA’s performance is evaluated using twenty-three function objectives from different unimodal and multimodal categories. While the optimization results for multimodal functions provide a great ability using POA exploration to discover the major optimal region of the space for searching, the optimization findings at unimodal functions demonstrate the high exploitation power of POA for seeking the most practicable solution. Additionally, the efficiency of the POA in maximizing real-world applications is determined using four engineering design issues. To evaluate POA’s optimization proficiency, its results are contrasted with those of eight popular metaheuristic algorithms. The Pelican Optimization Algorithm (POA) approach is used for residential loads in Singapore to minimize DSM issues and achieve load-shifting objectives. The results of the simulation show that the demand-side management approach under consideration lowers the highest load demand of the smart grid while producing significant cost savings.
Keywords:
Peak to Average load Ratio (PAR), Pelican Optimization Algorithm (POA), Demand Side Management (DSM), Smart Grid (SG).
References:
[1] M. Usman Saleem et al., “Integrating Smart Energy Management System with the Internet of Things and Cloud Computing for Efficient Demand Side Management in Smart Grids,” Energies, vol. 16, no. 12, pp. 1-21, 2023.
[CrossRef] [Google Scholar] [Publisher Link]
[2] Vinothini Arumugham et al., “An Artificial-Intelligence-Based Renewable Energy Prediction Program for Demand-Side Management in Smart Grids,” Sustainability, vol. 15, no. 6, pp. 1-26, 2023.
[CrossRef] [Google Scholar] [Publisher Link]
[3] Nehmedo Alamir et al., “Developing Hybrid Demand Response Technique for Energy Management in Microgrid Based on a Pelican Optimization Algorithm,” Electric Power Systems Research, vol. 214, 2023.
[CrossRef] [Google Scholar] [Publisher Link]
[4] Hamid Karimi, and Shahram Jadid, “Multi-Layer Energy Management of Smart Integrated-Energy Microgrid Systems Considering Generation and Demand-Side Flexibility,” Applied Energy, vol. 339, 2023.
[CrossRef] [Google Scholar] [Publisher Link]
[5] Bishwajit Dey, Soham Dutta, and Fausto Pedro Garcia Marquez, “Intelligent Demand Side Management for Exhaustive Techno-Economic Analysis of Microgrid System,” Sustainability, vol. 15, no. 3, pp. 1-15, 2023.
[CrossRef] [Google Scholar] [Publisher Link]
[6] Hoda Abd El-Sattar et al., “Maximizing Hybrid Microgrid System Performance: A Comparative Analysis and Optimization Using a Gradient Pelican Algorithm,” Renewable Energy, vol. 227, 2024.
[CrossRef] [Google Scholar] [Publisher Link]
[7] Jiyong Li et al., “Capacity Optimization of Independent Microgrid with Electric Vehicles Based on Improved Pelican Optimization Algorithm,” Energies, vol. 16, no. 6, pp. 1-23, 2023.
[CrossRef] [Google Scholar] [Publisher Link]
[8] Khaled Abedrabboh, and Luluwah Al-Fagih, “Applications of Mechanism Design in Market-Based Demand-Side Management: A Review,” Renewable and Sustainable Energy Reviews, vol. 171, 2023.
[CrossRef] [Google Scholar] [Publisher Link]
[9] Abdul Hafeez, Rashid Alammari, and Atif Iqbal, “Utilization of EV Charging Station in Demand Side Management Using Deep Learning Method,” IEEE Access, vol. 11, pp. 8747-8760, 2023.
[CrossRef] [Google Scholar] [Publisher Link]
[10] Ahmed Tijjani Dahiru et al., “A Comprehensive Review of Demand Side Management in Distributed Grids Based on Real Estate Perspectives,” Environmental Science and Pollution Research, vol. 30, pp. 81984-82013, 2023.
[CrossRef] [Google Scholar] [Publisher Link]
[11] Gokul Sidarth Thirunavukkarasu et al., “Role of Optimization Techniques in Microgrid Energy Management Systems-A Review,” Energy Strategy Reviews, vol. 43, 2022.
[CrossRef] [Google Scholar] [Publisher Link]
[12] Hanguan Wen et al., “An Energy Demand-Side Management and Net Metering Decision Framework,” Energy, vol. 271, 2023.
[CrossRef] [Google Scholar] [Publisher Link]
[13] Subhasis Panda et al., “Residential Demand Side Management Model, Optimization and Future Perspective: A Review,” Energy Reports, vol. 8, pp. 3727-3766, 2022.
[CrossRef] [Google Scholar] [Publisher Link]
[14] Eity Sarker et al., “Progress on the Demand Side Management in Smart Grid and Optimization Approaches,” International Journal of Energy Research, vol. 45, no.1, pp. 36-64, 2021.
[CrossRef] [Google Scholar] [Publisher Link]
[15] Salman Sadiq Shuvo, and Yasin Yilmaz, “Demand-Side and Utility-Side Management Techniques for Increasing EV Charging Load,” IEEE Transactions on Smart Grid, vol. 14, no. 5, pp. 3889-3898, 2023.
[CrossRef] [Google Scholar] [Publisher Link]
[16] Viorica Rozina Chifu et al., “Deep Q-Learning-Based Smart Scheduling of EVs for Demand Response in Smart Grids,” Applied Sciences, vol. 14, no. 4, pp. 1-21, 2024.
[CrossRef] [Google Scholar] [Publisher Link]
[17] P. Balakumar, T. Vinopraba, and K. Chandrasekaran, “Deep Learning Based Real Time Demand Side Management Controller for Smart Building Integrated with Renewable Energy and Energy Storage System,” Journal of Energy Storage, vol. 58, 2023.
[CrossRef] [Google Scholar] [Publisher Link]
[18] Karim M. Hassanin et al., “A Comparative Study for Two Novel Optimization Algorithms Used to Solve Microgrid Energy Management Problem Considering Energy Storage System,” Research Square, pp. 1-37, 2024.
[CrossRef] [Google Scholar] [Publisher Link]
[19] Hassan Wasim Khan et al., “Intelligent Optimization Framework for Efficient Demand-Side Management in Renewable Energy Integrated Smart Grid,” IEEE Access, vol. 9, pp. 124235-124252, 2021.
[CrossRef] [Google Scholar] [Publisher Link]
[20] S. Thejus, and Sivraj P., “Deep Learning-Based Power Consumption and Generation Forecasting for Demand Side Management,” 2021 Second International Conference on Electronics and Sustainable Communication Systems (ICESC), Coimbatore, India, pp. 1350-1357, 2021.
[CrossRef] [Google Scholar] [Publisher Link]