Multi-Area Economic Load Dispatch Using Deep Recurrent Model Expending Renewable Energy
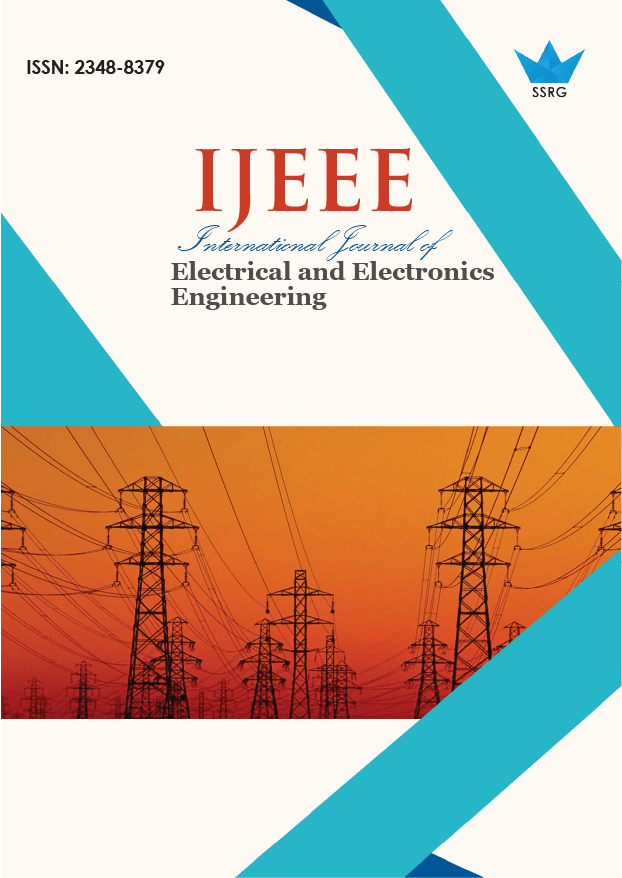
International Journal of Electrical and Electronics Engineering |
© 2024 by SSRG - IJEEE Journal |
Volume 11 Issue 7 |
Year of Publication : 2024 |
Authors : A. Antony Charles, R. Venkadesh |
How to Cite?
A. Antony Charles, R. Venkadesh, "Multi-Area Economic Load Dispatch Using Deep Recurrent Model Expending Renewable Energy," SSRG International Journal of Electrical and Electronics Engineering, vol. 11, no. 7, pp. 247-257, 2024. Crossref, https://doi.org/10.14445/23488379/IJEEE-V11I7P122
Abstract:
In minimizing the fuel cost through dispatch strategies by allocating power generation, Multi-Area Economic Load Dispatch (MAELD) poses a severe problem. The balance limitations must be met for the power distribution in economical load dispatch, and the generating limit, transmission line, and power balance limitations must be fulfilled. Traditional methods fail miserably when used to MAELD because of their complexity and non-linear issues. Many more metaheuristic algorithms have been used to solve the economic dispatch problems. In this research, an improvised version of the Deep Recurrent Neural Network model (DRNN) based on Long Short-Term Memory (LSTM) has been used to solve MAELD problems for four areas with a 3, 13 and 40-unit system. The LSTM algorithm combines the efficiency and diversity of heuristic search techniques with the subsistence of the most vital premise on or later evolutionary algorithms. This method eliminates the need on the way to comprehend the gradient of the optimization problem during the optimization search. The algorithm’s performance was examined in various unit systems, and fine-tuning the parameters reveals its unique qualities and vulnerabilities in the most appropriate applications. Compared to the other metamorphic procedures, the recommended system minimizes cost, valve point loading, and emission. Multi-Area Economic Load Dispatch solved three separate test scenarios. Using LSTM optimization methods, the optimal demand sharing of power-generating units is assessed. The simulation findings, generated using the MATLAB/Simulink platform, show that LSTM delivers high-quality cost solutions without violating constraints.
Keywords:
MAELD problem, Long Short-Term Memory, Evolutionary, Yield, Optimization.
References:
[1] Allen J. Wood, Bruce F. Wollenberg, and Gerald B. Sheble, Power Generation Operation and Control, 3rd ed., Wiley Publication, New York, USA, 2013.
[Google Scholar] [Publisher Link]
[2] K.P. Wong, and C.C. Fung, “Simulated Annealing Based Economic Dispatch Algorithm,” IEE Proceedings C (Generation, Transmission and Distribution), vol. 140, no. 6, pp. 509-515, 1993.
[CrossRef] [Google Scholar] [Publisher Link]
[3] D. Srinivasan, C.S. Chang, and A.C. Liew, “Multiobjective Generation Scheduling Using Fuzzy Optimal Search Technique”, IEE Proceedings - Generation, Transmission and Distribution, vol. 141, no. 3, pp. 233-242, 1994.
[CrossRef] [Google Scholar] [Publisher Link]
[4] A. Farag, S. Al-Baiyat, and T.C. Cheng, “Economic Load Dispatch Multiobjective Optimization Procedures Using Linear Programming Techniques”, IEEE Transactions on Power Systems, vol. 10, no. 2, pp. 731-738, 1995.
[CrossRef] [Google Scholar] [Publisher Link]
[5] D. Srinivasan, and A.G.B. Tettamanzi, “An Evolutionary Algorithm for Evaluation of Emission Compliance Options Given the Clean Air Act Amendments,” IEEE Transactions on Power Systems, vol. 12, no. 1, pp. 336-341, 1997.
[CrossRef] [Google Scholar] [Publisher Link]
[6] Carlos A. Coello Coello, “A Comprehensive Survey of Evolutionary-Based Multiobjective Optimization Techniques,” Knowledge and Information Systems, vol. 1, pp. 269-308, 1999.
[CrossRef] [Google Scholar] [Publisher Link]
[7] Kashif Hussain et al., “Metaheuristic Research: A Comprehensive Survey,” Artificial Intelligence Review, vol. 52, pp. 2191-2233, 2018.
[CrossRef] [Google Scholar] [Publisher Link]
[8] Fernando Fausto et al., “From Ants to Whales: Metaheuristics for All Tastes,” Artificial Intelligence Review, vol. 53, pp. 753-810, 2019.
[CrossRef] [Google Scholar] [Publisher Link]
[9] Yassine Meraihi et al., “Dragonfy Algorithm: A Comprehensive Review and Applications,” Neural Computing and Applications, vol. 32, pp. 16625-16646, 2020.
[CrossRef] [Google Scholar] [Publisher Link]
[10] Osama Ahmad Alomari et al., “Gene Selection for Microarray Data Classification Based on Grey Wolf Optimizer Enhanced with Triz - Inspired Operators,” Knowledge-Based Systems, vol. 223, 2021.
[CrossRef] [Google Scholar] [Publisher Link]
[11] Lamees Mohammad Dalbah et al., “A Modifed Coronavirus Herd Immunity Optimizer for Capacitated Vehicle Routing Problem,” Journal of King Saud University - Computer and Information Sciences, vol. 34, no. 8, part A, pp. 4782-4795, 2022.
[CrossRef] [Google Scholar] [Publisher Link]
[12] Raed Abu Zitar et al., “An Intensive and Comprehensive Overview of Jaya Algorithm, Its Versions and Applications,” Archives of Computational Methods in Engineering, vol. 29, pp. 763-792, 2021.
[CrossRef] [Google Scholar] [Publisher Link]
[13] Mahmud Salem Alkoffash et al., “A Nonconvex Economic Load Dispatch Using Hybrid Salp Swarm Algorithm,” Arabian Journal for Science and Engineering, vol. 46, pp. 8721-8740, 2021.
[CrossRef] [Google Scholar] [Publisher Link]
[14] Muhammed Jassem Al-Muhammed, and Raed Abu Zitar, “Probability-Directed Random Search Algorithm for an Unconstrained Optimization Problem,” Applied Soft Computing, vol. 71, pp. 165-182, 2018.
[CrossRef] [Google Scholar] [Publisher Link]
[15] Pengzhen Ren et al., “A Comprehensive Survey of Neural Architecture Search: Challenges and Solutions,” ACM Computing Surveys, vol. 54, no. 4, pp. 1-34, 2021.
[CrossRef] [Google Scholar] [Publisher Link]
[16] Jiefeng Liu et al., “Power System Load Forecasting Using Mobility Optimization and Multi-Task Learning in COVID-19,” Applied Energy, vol. 310, 2022.
[CrossRef] [Google Scholar] [Publisher Link]
[17] Barun Mandal, and Provas kumar Roy, “Dynamic Economic Dispatch Problem in Hybrid Wind Based Power Systems Using Oppositional Based Chaotic Grasshopper Optimization Algorithm,” Journal of Renewable and Sustainable Energy, vol. 13, no. 1, 2021.
[CrossRef] [Google Scholar] [Publisher Link]
[18] Manisha Sharma, Manjaree Pandit, and Laxmi Srivastava, “Multi-Area Economic Dispatch with Tie-Line Constraints Employing Evolutionary Approach”, International Journal of Engineering, Science and Technology, vol. 2, no. 3, pp. 132-149, 2010.
[CrossRef] [Google Scholar] [Publisher Link]
[19] Mohd Khairuzzaman Mohd Zamani et al., “Multi-Area Economic Dispatch Performance Using Swarm Intelligence Technique Considering Voltage Stability,” International Journal on Advanced Science Engineering Information Technology, vol. 7, no. 1, pp. 1-7, 2017.
[CrossRef] [Google Scholar] [Publisher Link]
[20] Mohammad Jafar Mokarram et al., “Robust and Effective Parallel Process to Coordinate Multi-Area Economic Dispatch (MAED) Problems in the Presence of Uncertainty,” IET Generation, Transmission & Distribution, vol. 13, no. 18, pp. 4197-4205, 2019.
[CrossRef] [Google Scholar] [Publisher Link]
[21] Cai Jiejin et al., “Chaotic Particle Swarm Optimization for Economic Dispatch Considering the Generator Constraints,” Energy Conversion and Management, vol. 48, no. 2, pp. 645-653, 2007.
[CrossRef] [Google Scholar] [Publisher Link]
[22] Bo Liu et al., “Improved Particle Swarm Optimization Combined with Chaos,” Chaos, Solitons & Fractals, vol. 25, no. 5, pp. 1261-1271, 2005.
[CrossRef] [Google Scholar] [Publisher Link]
[23] Yu Zhu et al., “Hierarchical Economic Load Dispatch Based on Chaotic-particle Swarm Optimization,” 2013 Ninth International Conference on Natural Computation (ICNC), Shenyang, China, pp. 517-521, 2013.
[CrossRef] [Google Scholar] [Publisher Link]
[24] Hamed Soleimani, and Govindan Kannan, “A Hybrid Particle Swarm Optimization and Genetic Algorithm for Closedloop Supply Chain Network Design in Large-scale Networks,” Applied Mathematical Modelling, vol. 39, no. 14, pp. 3990-4012, 2015.
[CrossRef] [Google Scholar] [Publisher Link]
[25] Jiansheng Wu, Jin Long, and Mingzhe Liu, “Evolving RBF Neural Networks for Rainfall Prediction Using Hybrid Particle Swarm Optimisation and Genetic Algorithm,” Neurocomputing, vol. 148, pp. 136-142, 2015.
[CrossRef] [Google Scholar] [Publisher Link]
[26] V.P. Sakthivel, and P.D. Sathya, “Multi-Area Economic Environmental Dispatch Using Multi Objective Squirrel Search Algorithm,” Evolving Systems, vol. 13, pp. 183-199, 2022.
[CrossRef] [Google Scholar] [Publisher Link]
[27] Farzad Habibi et al., “Simultaneous Multi-area Economic-Environmental Load Dispatch Modeling in Presence of Wind Turbines by MOPSO,” Journal of Electrical Engineering and Technology, vol. 15, pp. 1059-1072, 2020.
[CrossRef] [Google Scholar] [Publisher Link]
[28] Vishal Chaudhary et al., Multi-Area Economic Dispatch with Stochastic Wind Power Using the Salp Swarm Algorithm,” Array, vol. 8, pp. 1-13, 2020.
[CrossRef] [Google Scholar] [Publisher Link]
[29] Peng Zhang et al., “Multi-Area Economic Dispatching Using an Improved Grasshopper Optimization Algorithm,” Evolving Systems, vol. 12, pp. 837-847, 2021.
[CrossRef] [Google Scholar] [Publisher Link]
[30] Mohsen Zare et al., Reserve Constrained Dynamic Economic Dispatch in Multi-Area Power Systems: An Improved Fireworks Algorithm,” International Journal of Electrical Power and Energy Systems, vol. 126, part A, 2021.
[CrossRef] [Google Scholar] [Publisher Link]
[31] Bangwu dai, Fuli Wang, and Yuqing Chang, “Multi-Objective Ecnomic Load Dispatch Method Based on Data Mining Technology for Large Coal -Fired Power Plants”, Control Engineering Practice, vol. 121, 2022.
[CrossRef] [Google Scholar] [Publisher Link]
[32] Fan Sun et al., “Load-Forecasting Method for IES Based on LSTM and Dynamic Similar Days with Multi-Features,” Global Energy Interconnection, vol. 6, no. 3, pp. 285-296, 2023.
[CrossRef] [Google Scholar] [Publisher Link]
[33] Esra Cakir, and Ziya Ulukan, “Fuzzy Multiobjective Decision Approach for Nuclear Power Plant Installation,” Journal of Intelligent & Fuzzy Systems, vol. 39, no. 5, pp. 6339-6350, 2020.
[CrossRef] [Google Scholar] [Publisher Link]
[34] Homayoun Berahmandpour, Shahram Montaser Kouhsari, and Hassan Rastegar, “A New Flexibility Based Probabilistic Economic Load Dispatch Solution Incorporating Wind Power,” International Journal of Electrical Power & Energy Systems, vol. 135, 2022.
[CrossRef] [Google Scholar] [Publisher Link]
[35] Abhishek Srivastava, and Dushmanta Kumar Das, “An Adaptive Chaotic Topper Optimization Technique to Solve Economic Load Dispatch and Emission Economic Dispatch Problem in Power System,” Soft Computing, vol. 26, pp. 2913-2934, 2022.
[CrossRef] [Google Scholar] [Publisher Link]
[36] Ali Azizivahed et al., “An Efficient Hybrid Approach to Solve Bi-objective Multi-area Dynamic Economic Emission Dispatch Problem,” Electric Power Components and Systems, vol. 48, no. 4-5, pp. 485-500, 2020.
[CrossRef] [Google Scholar] [Publisher Link]
[37] Raed Abu Zitar, Laith Abualigah, and Nidal A. Al-Dmour, “Review and Analysis for the Red Deer Algorithm,” Journal of Ambient Intelligence and Humanized computing, vol. 14, pp. 8375-8385, 2021.
[CrossRef] [Google Scholar] [Publisher Link]
[38] A.M. Fathollahi-Fard, M. Niaz Azari, and M. Hajiaghaei-Keshteli, “An Improved Red Deer Algorithm for Addressing a Current Brushless Motor Design Problem,” International Journal of Science & Technology, vol. 28, no. 3, pp. 1750-1764, 2021.
[CrossRef] [Google Scholar] [Publisher Link]