A Polynomial Series-Based Data Aggregation and Spectrum Aware Clustering Technique for a Combined Model of WSN and Cognitive Radios in IoT Applications
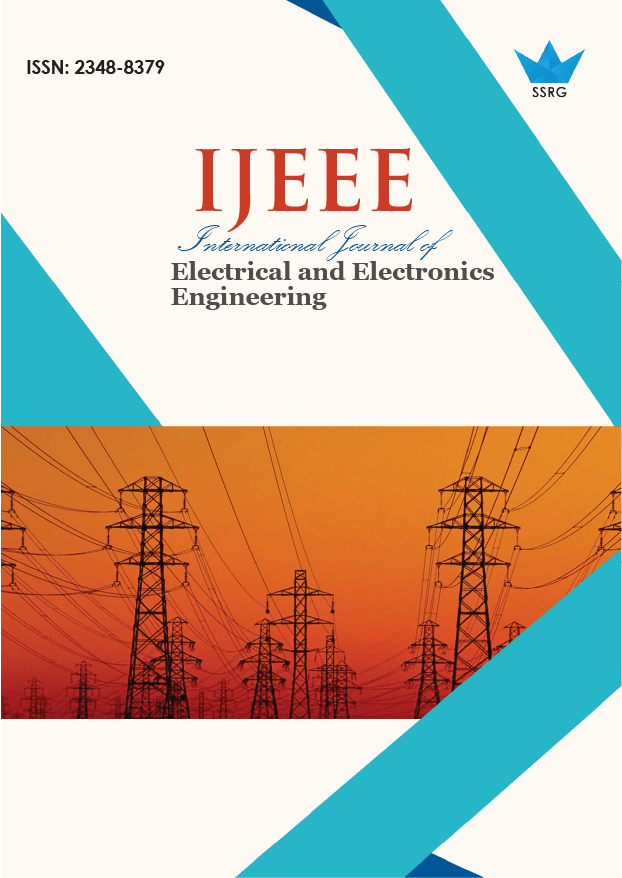
International Journal of Electrical and Electronics Engineering |
© 2024 by SSRG - IJEEE Journal |
Volume 11 Issue 7 |
Year of Publication : 2024 |
Authors : Shivaraj Sharanabasappa Kalburgi, M. Manimozhi |
How to Cite?
Shivaraj Sharanabasappa Kalburgi, M. Manimozhi, "A Polynomial Series-Based Data Aggregation and Spectrum Aware Clustering Technique for a Combined Model of WSN and Cognitive Radios in IoT Applications," SSRG International Journal of Electrical and Electronics Engineering, vol. 11, no. 7, pp. 258-270, 2024. Crossref, https://doi.org/10.14445/23488379/IJEEE-V11I7P123
Abstract:
The demand for wireless communication has surged due to the rise of IoT applications. Wireless Sensor Networks (WSNs) are crucial in this field but often operate in license-free spectrums, causing interference and impacting Quality of Service (QoS). Cognitive Radio (CR) technology offers a solution by enabling opportunistic access to licensed bands, thus reducing interference and enhancing system performance. However, integrating CR with IoT and WSNs poses challenges due to the high energy consumption of CR tasks and limited power and computation resources in sensor networks. Continuous data collection also leads to redundancy. To address these issues, data aggregation techniques can eliminate redundant data, and energy-aware routing can reduce energy consumption during data exchange. This study proposes a combined model of WSN and CR for IoT applications, utilizing a polynomial series-based data aggregation and spectrum-aware clustering technique. The proposed approach achieves a packet delivery rate of 95.47%, energy consumption of 5.216J, and a delay of 0.554 seconds, outperforming existing schemes in CRSNs.
Keywords:
Clustering, Cognitive Radios, Data aggregation, Spectrum management, Wireless Sensor Network.
References:
[1] Saleem Aslam, Waleed Ejaz, and Mohamed Ibnkahla, “Energy and Spectral Efficient Cognitive Radio Sensor Networks for Internet of Things,” IEEE Internet of Things Journal, vol. 5, no. 4, pp. 3220-3233, 2018.
[CrossRef] [Google Scholar] [Publisher Link]
[2] Kaneez Fizza et al., “QoE in IoT: A Vision, Survey and Future Directions,” Discover Internet of Things, vol. 1, pp. 1-14, 2021.
[CrossRef] [Google Scholar] [Publisher Link]
[3] Martin Bauer, Luis Sanchez, and JaeSeung Song, “IoT-Enabled Smart Cities: Evolution and Outlook,” Sensors, vol. 21, no. 13, pp. 1-29, 2021.
[CrossRef] [Google Scholar] [Publisher Link]
[4] Asfund Ausaf et al., “WLAN Aware Cognitive Medium Access Control Protocol for IoT Applications,” Future Internet, vol. 12, no. 1, pp. 1-21, 2020.
[CrossRef] [Google Scholar] [Publisher Link]
[5] Haitham Hassan Mahmoud et al., “Optimal Operational Parameters for 5G Energy Harvesting Cognitive Wireless Sensor Networks,” IETE Technical Review, vol. 34, no. sup1, pp. 62-72, 2017.
[CrossRef] [Google Scholar] [Publisher Link]
[6] Xuan Li et al., “Fault-Tolerant Topology Control towards K-Channel-Connectivity in Cognitive Radio Networks,” IEEE Access, vol. 6, pp. 65308-65320, 2018.
[CrossRef] [Google Scholar] [Publisher Link]
[7] Ram Narayan Yadav, and Rajiv Misra, “On K-Channel Connectivity in Cognitive Radio Networks through Channel Assignment,” AEU - International Journal of Electronics and Communications, vol. 77, pp. 118-129, 2017.
[CrossRef] [Google Scholar] [Publisher Link]
[8] Yan Shi et al., “Constructing a Robust Topology for Reliable Communications in Multi-Channel Cognitive Radio Ad Hoc Networks,” IEEE Communications Magazine, vol. 56, no. 4, pp. 172-179, 2018.
[CrossRef] [Google Scholar] [Publisher Link]
[9] Mohamad Rida Mortada et al., “In-Network Data Aggregation for Ad Hoc Clustered Cognitive Radio Wireless Sensor Network,” Sensors, vol. 21, no. 20, pp. 1-25, 2021.
[CrossRef] [Google Scholar] [Publisher Link]
[10] Sandeep Verma, “Energy-Efficient Routing Paradigm for Resource-Constrained Internet of Things-Based Cognitive Smart City,” Expert Systems, vol. 39, no. 5, 2022.
[CrossRef] [Google Scholar] [Publisher Link]
[11] Shivaraj Sharanabasappa Kalburgi, and M. Manimozhi, “Taylor-Spotted Hyena Optimization Algorithm for Reliable and Energy-Efficient Cluster Head Selection Based Secure Data Routing and Failure Tolerance in WSN,” Multimedia Tools and Applications, vol. 81, pp. 15815-15839, 2022.
[CrossRef] [Google Scholar] [Publisher Link]
[12] Rajendra Prajapat, Ram Narayan Yadav, and Rajiv Misra, “Energy-Efficient k-Hop Clustering in Cognitive Radio Sensor Network for Internet of Things,” IEEE Internet of Things Journal, vol. 8, no. 17, pp. 13593-13607, 2021.
[CrossRef] [Google Scholar] [Publisher Link]
[13] Anil Carie et al., “Cognitive Radio Assisted WSN with interference aware AODV Routing Protocol,” Journal of Ambient Intelligence and Humanized Computing, vol. 10, pp. 4033-4042, 2019.
[CrossRef] [Google Scholar] [Publisher Link]
[14] Haythem Bany Salameh, and Rasha Abusamra, “Intelligent Multicast Routing for Multimedia over Cognitive Radio Networks: A Probabilistic Approach,” Multimedia Tools and Applications, vol. 80, pp. 16731-16742, 2021.
[CrossRef] [Google Scholar] [Publisher Link]
[15] Ranjita Joon, and Parul Tomar, “Energy Aware Q-Learning AODV (EAQ-AODV) Routing for Cognitive Radio Sensor Networks,” Journal of King Saud University - Computer and Information Sciences, vol. 34, no. 9, pp. 6989-7000, 2022.
[CrossRef] [Google Scholar] [Publisher Link]
[16] S. Vimal et al., “Energy Enhancement Using Multiobjective Ant Colony Optimization with Double Q Learning Algorithm for IoT Based Cognitive Radio Networks,” Computer Communications, vol. 154, pp. 481-490, 2020.
[CrossRef] [Google Scholar] [Publisher Link]
[17] Nalluri Prophess Raj Kumar, and G. Josemin Bala, “A Cognitive Knowledged Energy-Efficient Path Selection Using Centroid and Ant-Colony Optimized Hybrid Protocol for WSN-Assisted IoT,” Wireless Personal Communications, vol. 124, pp. 1993-2028, 2022.
[CrossRef] [Google Scholar] [Publisher Link]
[18] Ram Narayan Yadav, Rajiv Misra, and Divya Saini, “Energy Aware Cluster Based Routing Protocol over Distributed Cognitive Radio Sensor Network,” Computer Communications, vol. 129, pp. 54-66, 2018.
[CrossRef] [Google Scholar] [Publisher Link]
[19] Yogesh Tripathi, Arun Prakash, and Rajeev Tripathi, “An Optimum Transmission Distance and Adaptive Clustering Based Routing Protocol for Cognitive Radio Sensor Network,” Wireless Personal Communications, vol. 116, pp. 907-926, 2021.
[CrossRef] [Google Scholar] [Publisher Link]
[20] K. Siddesha, G.V. Jayaramaiah, and C. Singh, “A Novel Deep Reinforcement Learning Scheme for Task Scheduling in Cloud Computing,” Cluster Computing, vol. 25, pp. 4171-4188, 2022.
[CrossRef] [Google Scholar] [Publisher Link]
[21] Yogita Thareja, and Kamal Kumar Sharma, “A Posterior Transition Probability-Based Model for Spectrum Sensing in Cognitive Radio Networks for Maximized Network Lifetime and Performance Enhancement,” International Journal of Communication Systems, vol. 34, no. 7, 2021.
[CrossRef] [Google Scholar] [Publisher Link]
[22] K. Siddesha, G.V. Jayaramaiah, and C. Singh, “A Novel Deep Reinforcement Learning Scheme for Task Scheduling in Cloud Computing,” Cluster Computing, vol. 25, pp. 4171-4188, 2022.
[CrossRef] [Google Scholar] [Publisher Link]
[23] Mustafa Ozger, and Ozgur B. Akan, “Event-Driven Spectrum-Aware Clustering in Cognitive Radio Sensor Networks,” 2013 Proceedings IEEE INFOCOM, Turin, Italy, pp. 1483-1491, 2013.
[CrossRef] [Google Scholar] [Publisher Link]
[24] Suat Ozdemir, and Yang Xiao, “Polynomial Regression Based Secure Data Aggregation for Wireless Sensor Networks,” 2011 IEEE Global Telecommunications Conference (GLOBECOM), Houston, TX, USA, pp. 1-5, 2011.
[CrossRef] [Google Scholar] [Publisher Link]
[25] Hyoil Kim, and Kang G. Shin, “Efficient Discovery of Spectrum Opportunities with MAC-Layer Sensing in Cognitive Radio Networks,” IEEE Transactions on Mobile Computing, vol. 7, no. 5, pp. 533-545, 2008.
[CrossRef] [Google Scholar] [Publisher Link]
[26] Jihong Wang, and Chang Liu, “An Imperfect Spectrum Sensing-Based Multi-Hop Clustering Routing Protocol for Cognitive Radio Sensor Networks,” Scientific Reports, vol. 13, pp. 1-16, 2023.
[CrossRef] [Google Scholar] [Publisher Link]
[27] D. Monica Satyavathi, and A. Ch. Sudhir, “OQ‐IICA: Optimal QoS‐Aware Intra‐Inter Cluster Data Aggregation Technique for IoT‐ Assisted WSNs Using Hybrid Optimization Techniques,” Concurrency and Computation: Practice and Experience, vol. 35, no. 22, 2023.
[CrossRef] [Google Scholar] [Publisher Link]
[28] V. Srividhya, and T. Shankar, “An Energy Efficient Distance-Based Spectrum Aware Hybrid Optimization Technique for Cognitive Radio Wireless Sensor Network,” Journal of the Institution of Engineers (India): Series B, vol. 104, no. 1, pp. 51-60, 2023.
[CrossRef] [Google Scholar] [Publisher Link]
[29] Sara Nasirian et al., “Pizzza: A Joint Sector Shape and Minimum Spanning Tree-Based Clustering Scheme for Energy Efficient Routing in Wireless Sensor Networks,” IEEE Access, vol. 11, pp. 68200-68215, 2023.
[CrossRef] [Google Scholar] [Publisher Link]