An Innovative Approach Involves Machine Learning Algorithms to Forecast Future Farmer Revenue
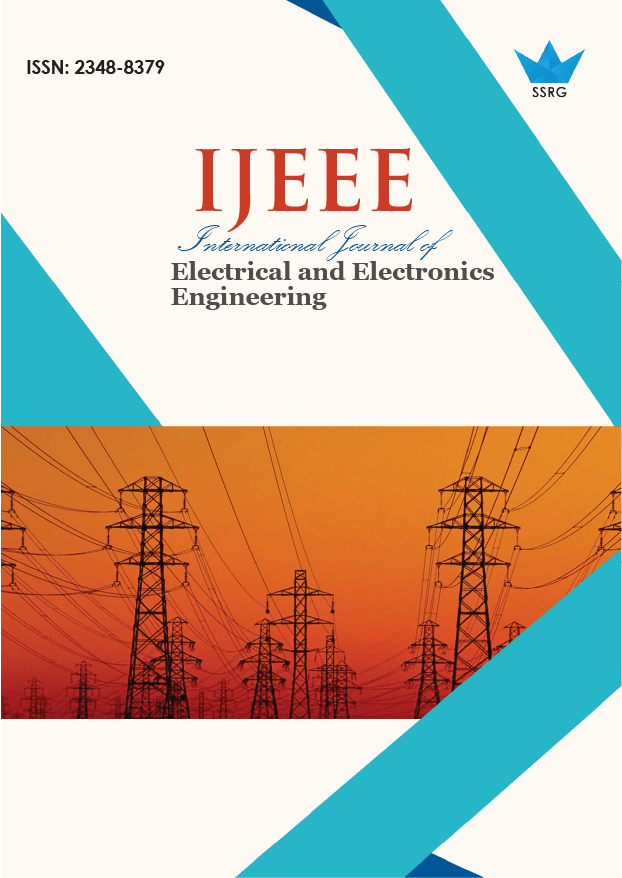
International Journal of Electrical and Electronics Engineering |
© 2024 by SSRG - IJEEE Journal |
Volume 11 Issue 8 |
Year of Publication : 2024 |
Authors : Saikat Banerjee, Abhoy Chand Mondal |
How to Cite?
Saikat Banerjee, Abhoy Chand Mondal, "An Innovative Approach Involves Machine Learning Algorithms to Forecast Future Farmer Revenue," SSRG International Journal of Electrical and Electronics Engineering, vol. 11, no. 8, pp. 59-71, 2024. Crossref, https://doi.org/10.14445/23488379/IJEEE-V11I8P106
Abstract:
Agriculture is crucial for meeting essential human needs and creating job opportunities worldwide. Agriculture provides many jobs and is essential to the economy in emerging countries like India. At this very moment, the features of weather patterns are experiencing unexpected changes due to increased pollution, climate change, and urbanization. The weather has a significant effect on how crops develop and grow. Precipitation, temperature swings, atmospheric moisture content, wind speed, and direction are some of the most essential local climatic factors that determine the success or failure of agricultural cultivation. Machine learning is a cutting-edge innovation that can solve real-world problems. Machine learning is a technique that allows computers to mimic human intelligence by learning from experience and analyzing various data sets. At this time, machine learning techniques are being used a lot in the agricultural sector. Using climatic data to predict crop yields, farmers may increase agricultural production, grow various crops, and use machine learning algorithms better. This research focuses on crop forecasting using weather data, including air temperature, humidity, rainfall, and sunshine hours. The suggested model predicts the maximum income in crops using change system agriculture. This paper proposes an intelligent agricultural system that uses machine learning to help farmers increase their income.
Keywords:
Crop, Machine Learning (ML), Economy, Minimum Support Price (MSP), Naïve Bayes (NB).
References:
[1] Subhrajit Mandal et al., “Adaption of Smart Applications in Agriculture to Enhance Production,” Smart Agricultural Technology, vol. 7, 2024.
[CrossRef] [Google Scholar] [Publisher Link]
[2] Rajesh Yamparla et al., “Crop Yield Prediction Using Random Forest Algorithm,” 2022 7th International Conference on Communication and Electronics Systems (ICCES), Coimbatore, India, pp. 1538-1543, 2022.
[CrossRef] [Google Scholar] [Publisher Link]
[3] Kranti G. Sapkal, and Avinash B. Kadam, “Random Forest Classifier for Crop Prediction Based on Soil Data,” Journal of Advanced Zoology, vol. 45, pp. 113-117, 2024.
[CrossRef] [Google Scholar] [Publisher Link]
[4] S. Rajeswari, and K. Suthendran, “C5.0: Advanced Decision Tree (ADT) Classification Model for Agricultural Data Analysis on Cloud,” Computers and Electronics in Agriculture, vol. 156, pp. 530-539, 2019.
[CrossRef] [Google Scholar] [Publisher Link]
[5] Mahmoud Y. Shams, Samah A. Gamel, and Fatma M. Talaat, “Enhancing Crop Recommendation Systems with Explainable Artificial Intelligence: A Study on Agricultural Decision-Making” Neural Computing and Applications, vol. 36, pp. 5695-5714, 2024.
[CrossRef] [Google Scholar] [Publisher Link]
[6] V. Vivek, and Rajat, “K-Nearest Neighbor (KNN), Soil Evaluation, Classifier and Accuracy,” 2022 5th International Conference on Contemporary Computing and Informatics (IC3I), Uttar Pradesh, India, pp. 698-705, 2022.
[CrossRef] [Google Scholar] [Publisher Link]
[7] Vimala Muninarayanappa, and Rajeev Ranjan, “Agriculture Data Analysis Using Parallel k-Nearest Neighbour Classification Algorithm,” International Journal of Reconfigurable and Embedded Systems, vol. 13, no. 2, pp. 332-340, 2024.
[CrossRef] [Publisher Link]
[8] Sudha Bishnoi, and B.K. Hooda, “Decision Tree Algorithms and their Applicability in Agriculture for Classification,” Journal of Experimental Agriculture International, vol. 44, no. 7, pp. 20-27, 2022.
[CrossRef] [Google Scholar] [Publisher Link]
[9] Lei Shi et al., “The Research of Support Vector Machine in Agricultural Data Classification,” Computer and Computing Technologies in Agriculture V, pp. 265-269, 2012.
[CrossRef] [Google Scholar] [Publisher Link]
[10] M. Rajakumaran et al., “Crop Yield Prediction Using Multi-Attribute Weighted Tree-Based Support Vector Machine,” Measurement: Sensors, vol. 31, 2024.
[CrossRef] [Google Scholar] [Publisher Link]
[11] National portal of India, Agriculture. [Online]. Available: https://www.india.gov.in/topics/agriculture
[12] West Bengal State Portal. [Online]. Available: https://wb.gov.in
[13] West Bengal State Agricultural Marketing Board [WBSAMB]. [Online]. Available: http://www.wbagrimarketingboard.gov.in
[14] Government of India, Ministry of Statistics & Programme Implementation, 2024. [Online]. Available: https://mospi.gov.in
[15] Purba Barddhaman, Agriculture, 2024. [Online]. Available: https://purbabardhaman.nic.in/agriculture
[16] District of Hooghly, 2020. [Online]. Available: https://hooghly.nic.in/
[17] Howrah, 2024. [Online]. Available: https://howrah.gov.in/
[18] Press Information Bureau, India, 2024. [Online]. Available: https://pib.gov.in/ & Department of Agriculture & Farmers Welfare, Govt. of India, 2023. [Online]. Available: https://agriwelfare.gov.in/
[19] Indian Agricultural Statistics Research Institute, 2024. [Online]. Available: https://iasri.icar.gov.in/ & Kisaan Helpline Assists Portal, 2024. [Online]. Available: https://www.kisaanhelpline.com
[20] Directorate of Economics & Statistics, Ministry of Agriculture and Farmers Welfare, Government of India, 2024. [Online]. Available: https://eands.da.gov.in/
[21] JioMart, 2024. [Online]. Available: https://www.jiomart.com/c/groceries/fruits-vegetables/fresh-vegetables
[22] Indiamart, 2024. [Online]. Available: https://dir.indiamart.com/impcat/freshvegetables.html
[23] Government of India, Ministry of Agriculture & Farmers Welfare, Department of Agriculture, Cooperation & Farmers Welfare, Directorate of Economics & Statistics, Pocket Book of Agricultural Statistics, 2017. from https://agriwelfare.gov.in/en/Agricultural_Statistics_at_a_Glance
[24] Gurdeep Singh Malhi, Manpreet Kaur, and Prashant Kaushik, “Impact of Climate Change on Agriculture and Its Mitigation Strategies: A Review,” Sustainability, vol. 13, no. 3, 2021.
[CrossRef] [Google Scholar] [Publisher Link]
[25] Sanjeev Kumar, and Sanjay Kumar Upadhyay, “Impact of Climate Change on Agricultural Productivity and Food Security in India: A State Level Analysis,” Indian Journal of Agricultural Research, vol. 53, no. 2, pp. 133-142, 2019.
[CrossRef] [Google Scholar] [Publisher Link]
[26] Sanjay Chaudhary, and P.K. Suri, “Framework for Agricultural e-Trading Platform Adoption Using Neural Networks,” International Journal of Information Technology, vol. 13, pp. 501-510, 2021.
[CrossRef] [Google Scholar] [Publisher Link]
[27] Rajeev Kumar Chaurasia, and Umesh Chandra Jaiswal, ‘Spatio-Temporal Based Video Anomaly Detection Using Deep Neural Networks,” International Journal of Information Technology, vol. 15, pp. 1569-1581, 2023.
[CrossRef] [Google Scholar] [Publisher Link]
[28] R. Vikram et al., “Crop Price Prediction Using Machine Learning Naive Bayes Algorithms,” Ubiquitous Intelligent Systems, pp. 27-34, 2022.
[CrossRef] [Google Scholar] [Publisher Link]
[29] Malti Bansal, Apoorva Goyal, and Apoorva Choudhary, “A Comparative Analysis of K-Nearest Neighbor, Genetic, Support Vector Machine, Decision Tree, and Long Short Term Memory Algorithms in Machine Learning,” Decision Analytics Journal, vol. 3, 2022.
[CrossRef] [Google Scholar] [Publisher Link]
[30] Amudha S. et al., “Deep Learning for Plant Disease Detection and Crop Yield Prediction Based on NPP-WPF Analysis in Smart Agriculture,” 2023 7th International Conference on I-SMAC (IoT in Social, Mobile, Analytics and Cloud) (I-SMAC), Kirtipur, Nepal, pp. 502-510, 2023.
[CrossRef] [Google Scholar] [Publisher Link]
[31] Sita Rani et al., “Machine Learning-Based Optimal Crop Selection System in Smart Agriculture,” Scientific Reports, vol. 13, 2023.
[CrossRef] [Google Scholar] [Publisher Link]