Hybrid Deep Learning Segmentation Method on Chest Radiograph Images for Lung Cancer Detection
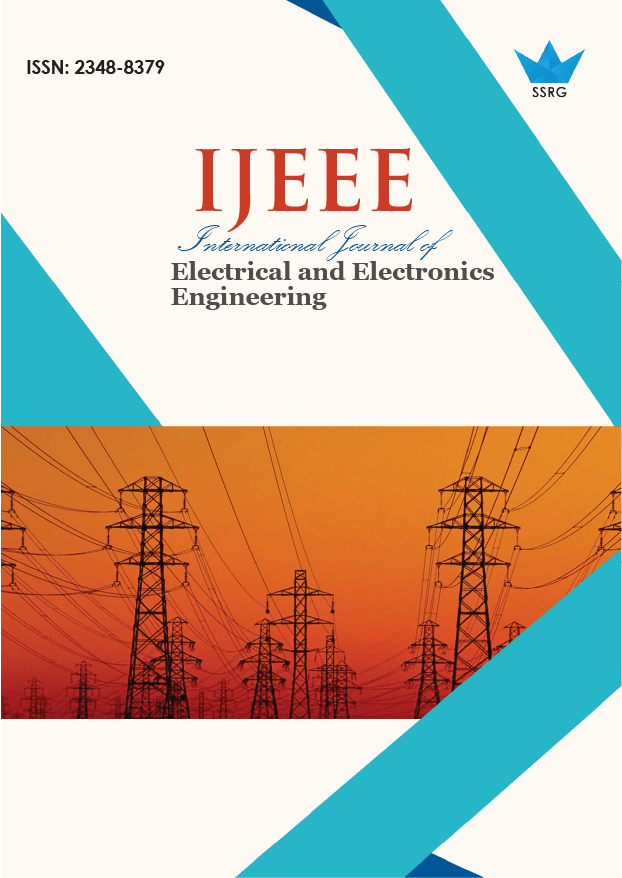
International Journal of Electrical and Electronics Engineering |
© 2024 by SSRG - IJEEE Journal |
Volume 11 Issue 8 |
Year of Publication : 2024 |
Authors : Raghuram Karla, Radhika Yalavarthi |
How to Cite?
Raghuram Karla, Radhika Yalavarthi, "Hybrid Deep Learning Segmentation Method on Chest Radiograph Images for Lung Cancer Detection," SSRG International Journal of Electrical and Electronics Engineering, vol. 11, no. 8, pp. 81-90, 2024. Crossref, https://doi.org/10.14445/23488379/IJEEE-V11I8P108
Abstract:
Chronic pulmonary diseases and lung cancer have become major respiratory concerns in the past decade. Their growing importance emphasizes their impact on public health and the need for better understanding, identification, and control. They increased deaths in India and abroad. High teen and adult smoking rates cause these events. Saving lives requires identifying lung cancer and COPD. Fast and effective diagnosis and treatment of the two disorders. This study employs chest radiographs, neural networks with artificial intelligence, machine learning algorithms, and deep learning techniques to accurately detect the two most lethal thoracic illnesses. Residual neural networks (ResNets) improve picture feature extraction and sickness classification. This approach analyzes chest radiograph imaging scan datasets with anomalies like tiny lobes or larger respiratory system capillaries better than lung imaging. Advanced AI and DL can provide healthcare monitoring systems with accurate insights and results. The dynamic field of oncology uses deep learning techniques. The research focuses on deep learning segmentation models. A model was created to improve chest radiography and lung cancer detection. Investigations using RID data. Model sensitivity and mean false positive are assessed independently. Compared to leading methods, RadiographNet has improved significantly.
Keywords:
Artificial Intelligence, Arterial infection, Lobes, Pulmonology, Smoking.
References:
[1] Yong Han et al., “Histologic Subtype Classification of Non-Small Cell Lung Cancer Using PET/CT Images,” European Journal of Nuclear Medicine and Molecular Imaging, vol. 48, pp. 350-360, 2021.
[CrossRef] [Google Scholar] [Publisher Link]
[2] Isaac Shiri et al., "Impact of Feature Harmonization on Radio Genomics Analysis: Prediction of EGFR and KRAS Mutations from Non-Small Cell Lung Cancer PET/CT Images,” Computers in Biology and Medicine, vol. 142, pp. 1-12, 2022.
[CrossRef] [Google Scholar] [Publisher Link]
[3] Tiening Zhang et al., “Simultaneous Identification of EGFR, KRAS, ERBB2, and TP53 Mutations in Patients with Non-Small Cell Lung Cancer by Machine Learning-Derived Three-Dimensional Radionics,” Cancers, vol. 13, no. 8, pp. 1-14, 2021.
[CrossRef] [Google Scholar] [Publisher Link]
[4] Marjolein A. Heuvelmans et al., “Lung Cancer Prediction by Deep Learning to Identify Benign Lung Nodules,” Lung Cancer, vol. 154, pp. 1-4, 2021.
[CrossRef] [Google Scholar] [Publisher Link]
[5] Beung-Chul Ahn et al., "Clinical Decision Support Algorithm Based on Machine Learning to Assess the Clinical Response to Anti-Programmed Death-1 Therapy in Patients with Non–Small-Cell Lung Cancer,” European Journal of Cancer, vol. 153, pp. 179-189, 2021.
[CrossRef] [Google Scholar] [Publisher Link]
[6] Margarita Kirienko et al., “Radiomics and Gene Expression Profiles to Characterise the Disease and Predict Outcomes in Patients with Lung Cancer,” European Journal of Nuclear Medicine and Molecular Imaging, vol. 48, pp. 3643-3655, 2021.
[CrossRef] [Google Scholar] [Publisher Link]
[7] Pragya Chaturvedi et al., “Prediction and Classification of Lung Cancer Using Machine Learning Techniques,” IOP Conference Series: Materials Science and Engineering, vol. 1099, 2021.
[CrossRef] [Google Scholar] [Publisher Link]
[8] Apurva Singh, Rhea Chitalia, and Despina Kontos, “Radiogenomics in Brain, Breast, and Lung Cancer: Opportunities and Challenges,” Journal of Medical Imaging, vol. 8, no. 3, 2021.
[CrossRef] [Google Scholar] [Publisher Link]
[9] Ichidai Tanaka, Taiki Furukawa, and Masahiro Morise, “The Current Issues and Future Perspective of Artificial Intelligence for Developing New Treatment Strategy in Non-Small Cell Lung Cancer: Harmonization of Molecular Cancer Biology and Artificial Intelligence,” Cancer Cell International, vol. 21, no. 1, pp. 1-14, 2021.
[CrossRef] [Google Scholar] [Publisher Link]
[10] Panagiotis Marentakis et al., “Lung Cancer Histology Classification from CT Images Based on Radiomics and Deep Learning Models,” Medical & Biological Engineering & Computing, vol. 59, pp. 215-226, 2021.
[CrossRef] [Google Scholar] [Publisher Link]
[11] Colton Ladbury et al., “Integration of Artificial Intelligence in Lung Cancer: Rise of the Machine,” Cell Reports Medicine, pp. 1-11, 2023.
[CrossRef] [Google Scholar] [Publisher Link]
[12] Hanaa Mohammed Elsayed Mohammed El-Brolsy et al., “Fighting Non-Small Lung Cancer Cells Using Optimal Functionalization of Targeted Carbon Quantum Dots Derived from Natural Sources Might Provide Potential Therapeutic and Cancer Bio-Image Strategies,” International Journal of Molecular Sciences, vol. 23, no. 21, pp. 1-23, 2022.
[CrossRef] [Google Scholar] [Publisher Link]
[13] Ilke Tunali, Robert J. Gillies, and Matthew B. Schabath, “Application of Radiomics and Artificial Intelligence for Lung Cancer Precision Medicine,” Cold Spring Harbor Perspectives in Medicine, vol. 14, no. 8, pp. 1-25, 2021.
[CrossRef] [Google Scholar] [Publisher Link]
[14] Chintakayala Tejaswini et al., “CNN Architecture for Lung Cancer Detection,” 2022 IEEE 11th International Conference on Communication Systems and Network Technologies (CSNT), Indore, India, pp. 346-350, 2022.
[CrossRef] [Google Scholar] [Publisher Link]
[15] Nanhang Zhu et al., “A Light-Up Fluorescence Resonance Energy Transfer Magnetic Aptamer Sensor for Ultra-Sensitive Lung Cancer Exosome Detection,” Journal of Materials Chemistry B, vol. 9, pp. 2483-2493, 2021.
[CrossRef] [Google Scholar] [Publisher Link]
[16] Malathi Murugesan et al., “A Hybrid Deep Learning Model for Effective Segmentation and Classification of Lung Nodules from CT Images,” Journal of Intelligent & Fuzzy Systems, vol. 42, no. 3, pp. 2667-2679, 2022.
[CrossRef] [Google Scholar] [Publisher Link]
[17] Saad Awadh Alanazi et al., “Boosting Breast Cancer Detection Using Convolutional Neural Network,” Journal of Healthcare Engineering, vol. 2021, pp. 1-11, 2021.
[CrossRef] [Publisher Link]
[18] Mehedi Masud et al., “A Machine Learning Approach to Diagnosing Lung and Colon Cancer Using A Deep Learning-Based Classification Framework,” Sensors, vol. 21, no. 3, pp. 1-20, 2021.
[CrossRef] [Google Scholar] [Publisher Link]
[19] Mehdi Amini et al., “Overall Survival Prognostic Modelling of Non-Small Cell Lung Cancer Patients Using Positron Emission Tomography/Computed Tomography Harmonised Radionics Features: the Quest for the Optimal Machine Learning Algorithm,” Clinical Oncology, vol. 34, no. 2, pp. 114-127, 2022.
[CrossRef] [Google Scholar] [Publisher Link]
[20] Shalini Wankhade, and S. Vigneshwari, “A Novel Hybrid Deep Learning Method for Early Detection of Lung Cancer Using Neural Networks,” Healthcare Analytics, vol. 3, pp. 1-13, 2023.
[CrossRef] [Google Scholar] [Publisher Link]
[21] G.M.F. Wallace et al., “Chest X-Rays in COPD Screening: Are they Worthwhile?,” Respiratory Medicine, vol. 103, no. 12, pp. 1862-1865, 2009.
[CrossRef] [Google Scholar] [Publisher Link]
[22] Giorgio Maria Agazzi et al., “CT Texture Analysis for Prediction of EGFR Mutational Status and ALK Rearrangement in Patients with Non-Small Cell Lung Cancer,” La Radiologia Medica, vol. 126, pp. 786-794, 2021.
[CrossRef] [Google Scholar] [Publisher Link]
[23] Daiju Ueda et al., “Artificial Intelligence-Supported Lung Cancer Detection by Multi-Institutional Readers with Multi-Vendor Chest Radiographs: A Retrospective Clinical Validation Study,” BMC Cancer, vol. 21, pp. 1-8, 2021.
[CrossRef] [Google Scholar] [Publisher Link]
[24] Samuel G. Armato III et al., “The Lung Image Database Consortium (LIDC) and Image Database Resource Initiative (IDRI): A Completed Reference Database of Lung Nodules on CT Scans,” The International Journal of Medical Physics Research and Practice, vol. 38, no. 2, pp. 915-931, 2011.
[CrossRef] [Google Scholar] [Publisher Link]
[25] Tafadzwa L. Chaunzwa et al., “Deep Learning Classification of Lung Cancer Histology Using CT Images,” Scientific Reports, vol. 11, pp. 1-12, 2021.
[CrossRef] [Google Scholar] [Publisher Link]