A Comprehensive Review of Deepfake and its Detection Techniques
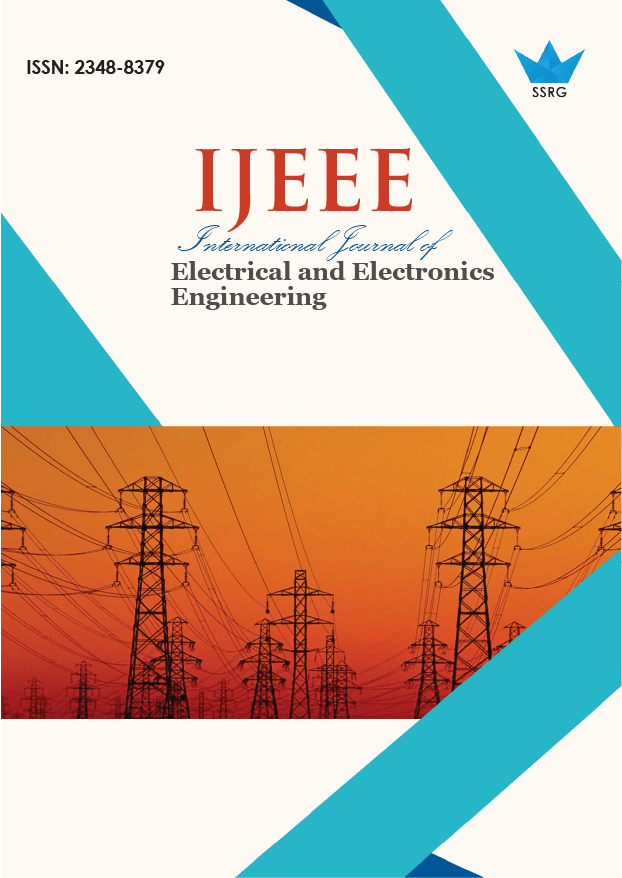
International Journal of Electrical and Electronics Engineering |
© 2024 by SSRG - IJEEE Journal |
Volume 11 Issue 8 |
Year of Publication : 2024 |
Authors : Tatwadarshi P. Nagarhalli, Ashwini Save, Sanket Patil, Uday Aswalekar |
How to Cite?
Tatwadarshi P. Nagarhalli, Ashwini Save, Sanket Patil, Uday Aswalekar, "A Comprehensive Review of Deepfake and its Detection Techniques," SSRG International Journal of Electrical and Electronics Engineering, vol. 11, no. 8, pp. 121-133, 2024. Crossref, https://doi.org/10.14445/23488379/IJEEE-V11I8P111
Abstract:
Deepfake technology has emerged as a significant concern in the era of digital media, posing threats to various sectors by enabling the creation of highly realistic synthetic content. This paper presents a comprehensive review of deepfake techniques and detection methods. It analyzes 14 research papers covering a range of approaches, including machine learning algorithms, computer vision techniques, and signal processing methods. Key aspects explored include face and voice manipulation, multimodal fusion, and the use of attention mechanisms. The review highlights the challenges in detecting deepfakes, such as dataset bias and the arms race between creators and detectors. Additionally, it discusses the limitations of current detection techniques and the need for robust, scalable solutions. Through a critical analysis of the literature, this review provides insights into the strengths and weaknesses of existing approaches and identifies areas for future research. The paper contributes to understanding deepfake technology and its implications for society, emphasizing the importance of developing effective detection mechanisms to combat the spread of synthetic media.
Keywords:
Deepfake, Deepfake detection, Face swap, Audio-video manipulation, Deep Learning, Voice spoofing, Synthetic media.
References:
[1] Manju M. et al., “Smart Fields: Enhancing Agriculture with Machine Learning,” 2024 2nd International Conference on Artificial Intelligence and Machine Learning Applications Theme: Healthcare and Internet of Things (AIMLA), Namakkal, India, pp. 1-5, 2024.
[CrossRef] [Google Scholar] [Publisher Link]
[2] Harini B. et al., “Advanced Sound Detection and Behavior Examination for Real-Time Intruder Detection Using Deep Learning: A Comprehensive Security Framework,” 2024 2nd International Conference on Artificial Intelligence and Machine Learning Applications Theme: Healthcare and Internet of Things (AIMLA), Namakkal, India, pp. 1-6, 2024.
[CrossRef] [Google Scholar] [Publisher Link]
[3] Smriti Sett, and Ajay Vikram Singh, “Applying Natural Language Processing in Healthcare Using Data Science,” 2024 11th International Conference on Reliability, Infocom Technologies and Optimization (Trends and Future Directions) (ICRITO), Noida, India, pp. 1-6, 2024.
[CrossRef] [Google Scholar] [Publisher Link]
[4] Tom M. Mitchel, Machine Learning, McGraw-Hill Science / Engineering / Math, 1997.
[Google Scholar]
[5] Yann LeCun, Yoshua Bengio, and Geoffrey Hinton, “Deep Learning,” Review Articles, vol. 521, pp. 436-444, 2015.
[CrossRef] [Google Scholar] [Publisher Link]
[6] Daniel Jurafsky, and James H. Martin, “Speech and Language Processing: An Introduction to Natural Language Processing, Computational Linguistics and Speech Recognition,” Computational Linguistics, vol. 26, no. 4, pp. 638-641, 2000. [CrossRef] [Google Scholar] [Publisher Link]
[7] Jacques Bughin et al., “Artificial Intelligence The Next Digital Frontier?,” McKinsey & Company, 2017.
[Google Scholar] [Publisher Link]
[8] Ian J. Goodfellow et al., “Generative Adversarial Networks,” Communications of the ACM, vol. 63, no. 11, pp. 139-144, 2020.
[CrossRef] [Google Scholar] [Publisher Link]
[9] Diederik P. Kingma, and Max Welling, “Auto-Encoding Variational Bayes,” arXiv, 2022.
[CrossRef] [Google Scholar] [Publisher Link]
[10] Gregory Barber, Deepfakes Are Getting Better, But They're Still Easy to Spot, WIRED, 2019. [Online]. Available: https://www.wired.com/story/deepfakes-getting-better-theyre-easy-spot/
[11] Diya Garg, and Rupali Gill, “Deepfake Generation and Detection - An Exploratory Study,” 2023 10th IEEE Uttar Pradesh Section International Conference on Electrical, Electronics and Computer Engineering (UPCON), Gautam Buddha Nagar, India, pp. 888-893, 2023.
[CrossRef] [Google Scholar] [Publisher Link]
[12] Chandrasekaran, “The Rise of Deepfake Technology: A Threat to the Future of Truth?,” Journal of Cybersecurity, vol. 6, no. 1, 2020.
[13] Aaron van den Oord et al., “WaveNet: A Generative Model for Raw Audio,” arXiv, 2016.
[CrossRef] [Google Scholar] [Publisher Link]
[14] Yuxuan Wang et al., “Tacotron: Towards End-to-End Speech Synthesis,” arXiv, 2017.
[CrossRef] [Google Scholar] [Publisher Link]
[15] Yuval Nirkin, Yosi Keller, and Tal Hassner, “FSGANv2: Improved Subject Agnostic Face Swapping and Reenactment,” IEEE Transactions on Pattern Analysis and Machine Intelligence, vol. 45, no. 1, pp. 560-575, 2023.
[CrossRef] [Google Scholar] [Publisher Link]
[16] Caroline Chan et al., “Everybody Dance Now,” 2019 IEEE/CVF International Conference on Computer Vision (ICCV), Seoul, Korea (South), pp. 5932-5941, 2019.
[CrossRef] [Google Scholar] [Publisher Link]
[17] Alec Radford et al., “Improving Language Understanding by Generative Pre-Training,” Preprint, 2018.
[Google Scholar]
[18] Tero Karras, Samuli Laine, and Timo Aila, “A Style-Based Generator Architecture for Generative Adversarial Networks,” IEEE Transactions on Pattern Analysis and Machine Intelligence, vol. 43, no. 12, pp. 4217-4228, 2021.
[CrossRef] [Google Scholar] [Publisher Link]
[19] Supasorn Suwajanakorn, Steven M. Seitz, and Ira Kemelmacher-Shlizerman, “Synthesizing Obama: Learning Lip Sync from Audio,” ACM Transactions on Graphics, vol. 36, no. 4, pp 1-13, 2017.
[CrossRef] [Google Scholar] [Publisher Link]
[20] H. Zhou, J. Zhang, and J. Zhang, “Audio-Visual Deepfakes,” arXiv, 2020.
[21] Andreas Rössler et al., “FaceForensics++: Learning to Detect Manipulated Facial Images,” arXiv, 2019.
[CrossRef] [Google Scholar] [Publisher Link]
[22] Sanskriti Chandra et al., “A Novel Framework for Detection of Digital Face Video Manipulation Using Deep Learning,” 2023 3rd International Conference on Computing and Information Technology (ICCIT), Tabuk, Saudi Arabia, pp. 348-352, 2023.
[CrossRef] [Google Scholar] [Publisher Link]
[23] Chenqi Kong, Haoliang Li, and Shiqi Wang, “Enhancing General Face Forgery Detection via Vision Transformer with Low-Rank Adaptation,” 2023 IEEE 6th International Conference on Multimedia Information Processing and Retrieval (MIPR), Singapore, pp. 102-107, 2023.
[CrossRef] [Google Scholar] [Publisher Link]
[24] C. Rathgeb et al., “Crowd-Powered Face Manipulation Detection: Fusing Human Examiner Decisions,” 2022 IEEE International Conference on Image Processing (ICIP), Bordeaux, France, pp. 181-185, 2022.
[CrossRef] [Google Scholar] [Publisher Link]
[25] Zhiyuan Ma, Xue Mei, and Jie Shen, “3D Attention Network for Face Forgery Detection,” 2023 4th Information Communication Technologies Conference (ICTC), Nanjing, China, pp. 396-401, 2023.
[CrossRef] [Google Scholar] [Publisher Link]
[26] Farman Hassan, and Ali Javed, “Voice Spoofing Countermeasure for Synthetic Speech Detection,” 2021 IEEE International Conference on Artificial Intelligence (ICAI), Islamabad, Pakistan, pp. 209-212, 2021.
[CrossRef] [Google Scholar] [Publisher Link]
[27] Hafiz Mallik, “Securing Voice-Driven Interfaces against Fake (Cloned) Audio Attacks,” 2019 IEEE Conference on Multimedia Information Processing and Retrieval (MIPR), San Jose, CA, USA, pp. 512-517, 2019.
[CrossRef] [Google Scholar] [Publisher Link]
[28] Aakriti Aggarwal et al., “IsSwap: Deep Fake Detection,” 2021 7th International Conference on Signal Processing and Communication (ICSC), Noida, India, pp. 194-199, 2021.
[CrossRef] [Google Scholar] [Publisher Link]
[29] Yuan Wang et al., “Dynamic Graph Learning with Content-guided Spatial-Frequency Relation Reasoning for Deepfake Detection,” 2023 IEEE/CVF Conference on Computer Vision and Pattern Recognition (CVPR), Vancouver, BC, Canada, pp. 7278-7287, 2023.
[CrossRef] [Google Scholar] [Publisher Link]
[30] Rui Shao, Tianxing Wu, and Ziwei Liu, “Detecting and Grounding Multi-Modal Media Manipulation,” IEEE/CVF Conference on Computer Vision and Pattern Recognition (CVPR), Vancouver, BC, Canada, pp. 6904-6913, 2023.
[Google Scholar] [Publisher Link]
[31] Qianyu Zhou et al., “Instance-Aware Domain Generalization for Face Anti-Spoofing,” IEEE/CVF Conference on Computer Vision and Pattern Recognition (CVPR), Vancouver, BC, Canada, pp. 20453-20463, 2023.
[CrossRef] [Google Scholar] [Publisher Link]
[32] Aman Parikh et al., “Audio-Visual Deepfake Detection System Using Multimodal Deep Learning,” 2023 IEEE 3rd International Conference on Intelligent Technologies (CONIT), Hubli, India, pp. 1-6, 2023.
[CrossRef] [Google Scholar] [Publisher Link]
[33] Chao Feng, Ziyang Chen, and Andrew Owens, “Self-Supervised Video Forensics by Audio-Visual Anomaly Detection,” 2023 IEEE/CVF Conference on Computer Vision and Pattern Recognition (CVPR), pp. 10491-10503, 2023.
[CrossRef] [Google Scholar] [Publisher Link]
[34] Yipin Zhou, and Ser-Nam Lim, “Joint Audio-Visual Deepfake Detection,” IEEE/CVF International Conference on Computer Vision (ICCV), Montreal, QC, Canada, pp. 14780-14789, 2021.
[CrossRef] [Google Scholar] [Publisher Link]
[35] Anders Boesen Lindbo Larsen et al., “Autoencoding Beyond Pixels Using a Learned Similarity Metric,” ICML'16: Proceedings of the 33rd International Conference on International Conference on Machine Learning, vol. 48, pp. 1558-1566, 2016. [Google Scholar] [Publisher Link]
[36] Kaggle, Deepfake Detection Challenge, Identify Videos with Facial or Voice Manipulations, 2020. [Online]. Available: https://www.kaggle.com/c/deepfake-detection-challenge
[37] Kaggle, Celeb DF (v2). [Online]. Available: https://www.kaggle.com/datasets/reubensuju/celeb-df-v2
[38] EndlessSora/DeeperForensics-1.0, [CVPR 2020] A Large-Scale Dataset for Real-World Face Forgery Detection, 2020. [Online]. Available: https://github.com/EndlessSora/DeeperForensics-1.0
[39] Nick Dufour, Contributing Data to Deepfake Detection Research, Google AI Blog, 2019. [Online]. Available: https://ai.googleblog.com/2019/09/contributing-data-to-deepfake-detection.html
[40] Bojia Zi et al., “WildDeepfake: A Challenging Real-World Dataset for Deepfake Detection,” Proceedings of the 28th ACM International Conference on Multimedia, pp. 2382-2390, 2020.
[CrossRef] [Google Scholar] [Publisher Link]