Evaluating the Culture and Tourism Integration Model of the Grand Canal National Cultural Park Using Machine Learning Algorithms
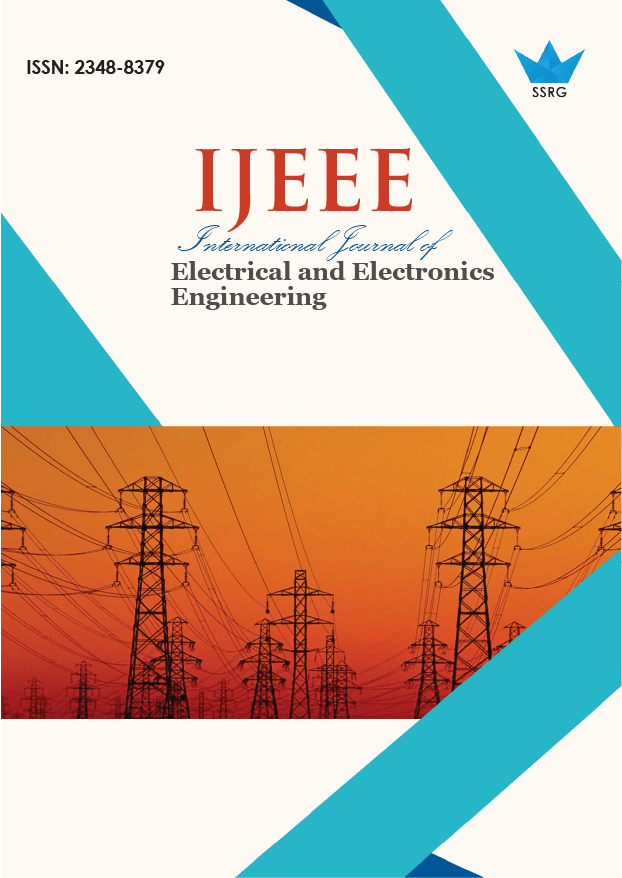
International Journal of Electrical and Electronics Engineering |
© 2024 by SSRG - IJEEE Journal |
Volume 11 Issue 9 |
Year of Publication : 2024 |
Authors : Xingyu Feng, Chunyun Wang, Tongqian Zou |
How to Cite?
Xingyu Feng, Chunyun Wang, Tongqian Zou, "Evaluating the Culture and Tourism Integration Model of the Grand Canal National Cultural Park Using Machine Learning Algorithms," SSRG International Journal of Electrical and Electronics Engineering, vol. 11, no. 9, pp. 151-159, 2024. Crossref, https://doi.org/10.14445/23488379/IJEEE-V11I9P113
Abstract:
The Grand Canal National Cultural Park's cultural and tourism integration approach is evaluated using machine learning techniques in this study. The goal is to enhance visitor experiences while preserving cultural heritage. It also covered visitor data collection through the use of surveys, social media analytics, and historical records to capture various dimensions of visitor behaviour and satisfaction. Extensive pre-processing of data collected includes NLP for social media text analysis, and feature engineering was carried out to bring forth relevant insights about visitors. Satisfaction and preferences of visitors are predicted using three machine-learning models: Random Forests, Decision Trees, and SVMs. The Random Forest model, however, topped with an accuracy of 88.7%, precision of 86.9%, recall of 87.3%, and an F1-Score of 87.1%. Since the model was based on ensemble learning, it was able to make strong predictions by taking in the collective intelligence of a set of multiple decision trees. In contrast, interpretability was provided by the Decision Tree model with its F1-Score of 79.8% and accuracy of 82.3%. Knowing that SVM tends to perform very well in high-dimensional spaces, the model reached an F1 score of 83.9% with an accuracy of 85.5%, hence proving to be competitive. It, therefore, underlines the potentiality of machine learning for taking cultural tourism management to the next level by allowing improved forecasts of visitors' behaviors and preferences. This, therefore, points to further research in refining predictive models with increased data while exploring adaptive management frameworks that would maintain resilient levels of cultural tourism practices at heritage sites such as the Grand Canal National Cultural Park.
Keywords:
Cultural tourism, Machine Learning, Visitor satisfaction, Heritage site management, Predictive analytics.
References:
[1] UNESCO, “The Grand Canal,” 2016. [Online]. Available: https://whc.unesco.org/en/list/1443/
[2] Zhao Yun, The Grand Canal and its National Cultural Park, City of Architecture and Heritage, 2024. [Online]. Available: https://www.citedelarchitecture.fr/fr/article/grand-canal-and-its-national-cultural-park#:~:text=Relying%20on%20important%20resources%20to%20establish%20the,a%20symbol%20of%20China's%20diversity%20and%20integration.
[3] Muyan Tang, and Hongzhang Xu, “Cultural Integration and Rural Tourism Development: A Scoping Literature Review,” Tourism and Hospitality, vol. 4, no. 1, pp. 75-90, 2023.
[CrossRef] [Google Scholar] [Publisher Link]
[4] Roman Egger, “Machine Learning in Tourism: A Brief Overview: Generation of Knowledge from Experience,” Applied Data Science in Tourism, Generation of Knowledge from Experience, pp. 85-107, 2022.
[CrossRef] [Google Scholar] [Publisher Link]
[5] Ying Chen, “The Hot Spots and Frontiers of Research on the Grand Canal Culture Belt in China: Literature and Academic Trends,” Humanities and Social Sciences Communications, vol. 9, 2022.
[CrossRef] [Google Scholar] [Publisher Link]
[6] Ioanna Farsari, “Exploring the Nexus between Sustainable Tourism Governance, Resilience and Complexity Research,” Tourism Recreation Research, vol. 48, no. 3, pp. 352-367, 2023.
[CrossRef] [Google Scholar] [Publisher Link]
[7] Rohit Agrawal et al., “Big Data Analytics and Sustainable Tourism: A Comprehensive Review and Network-Based Analysis for Potential Future Research,” International Journal of Information Management Data Insights, vol. 2, no. 2, 2022.
[CrossRef] [Google Scholar] [Publisher Link]
[8] Zohreh Doborjeh et al., “Artificial Intelligence: A Systematic Review of Methods and Applications in Hospitality and Tourism,” International Journal of Contemporary Hospitality Management, vol. 34, no. 3, pp. 1154-1176, 2022.
[CrossRef] [Google Scholar] [Publisher Link]
[9] Yuan Li et al., “A Review of the Tools and Techniques Used in the Digital Preservation of Architectural Heritage within Disaster Cycles,” Heritage Science, vol. 11, 2023.
[CrossRef] [Google Scholar] [Publisher Link]
[10] Fuad Mehraliyev, Irene Cheng Chu Chan, and Andrei Petrovich Kirilenko, “Sentiment Analysis in Hospitality and Tourism: A Thematic and Methodological Review,” International Journal of Contemporary Hospitality Management, vol. 34, no. 1, pp. 46-77, 2022.
[CrossRef] [Google Scholar] [Publisher Link]
[11] Elliot Mbunge, and Benhildah Muchemwa, “Deep Learning and Machine Learning Techniques for Analyzing Travellers’ Online Reviews: A Review,” Optimizing Digital Solutions for Hyper-Personalization in Tourism and Hospitality, 2022.
[CrossRef] [Google Scholar] [Publisher Link]
[12] Li Ying et al., “The Development of Digital Tourism in China,” Cogent Social Sciences, vol. 10, no. 1, 2024.
[CrossRef] [Google Scholar] [Publisher Link]
[13] Wen Zhen Li, and Hong Zhong, “Development of a Smart Tourism Integration Model to Preserve the Cultural Heritage of Ancient Villages in Northern Guangxi,” Heritage Science, vol. 10, 2022.
[CrossRef] [Google Scholar] [Publisher Link]
[14] Yiwen Li, and Bing Qiu, “Using Deep Learning Approaches to Quantify Landscape Preference of the Chinese Grand Canal: An Empirical Case Study of the Yangzhou Ancient Canal,” Sustainability, vol. 16, no. 9, 2024.
[CrossRef] [Google Scholar] [Publisher Link]
[15] Yinghua He, and Lei Wu, “Analysis on Spatial Development Mode of Eco-Sports Tourism in Grand Canal Landscape Environment Culture Belt,” Environmental Monitoring and Assessment, vol. 194, 2022.
[CrossRef] [Google Scholar] [Publisher Link]