Psychological Analysis of Social Media Visual Content Based on Image Recognition Algorithm
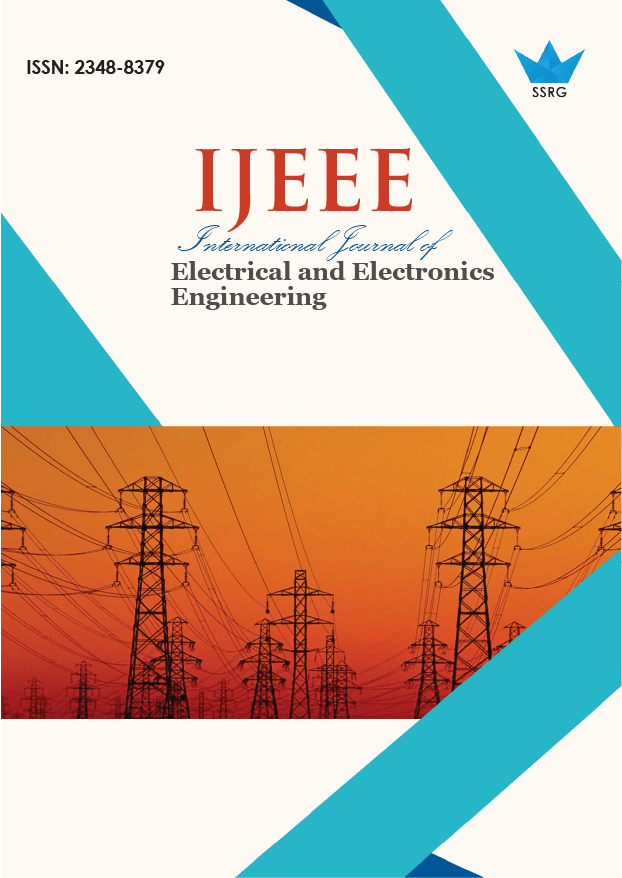
International Journal of Electrical and Electronics Engineering |
© 2024 by SSRG - IJEEE Journal |
Volume 11 Issue 9 |
Year of Publication : 2024 |
Authors : Zhaohao Jia |
How to Cite?
Zhaohao Jia, "Psychological Analysis of Social Media Visual Content Based on Image Recognition Algorithm," SSRG International Journal of Electrical and Electronics Engineering, vol. 11, no. 9, pp. 196-204, 2024. Crossref, https://doi.org/10.14445/23488379/IJEEE-V11I9P117
Abstract:
Technological advancement has seen social media airways availing a steady stream of visual content, which has compounded the need to create new and effective ways of uncovering the psychological aspects inherent to these images. This work aims to investigate the possibility of applying modern image processing techniques to classify the affective and referential features of social media images. To analyze and feature extract from a diverse set of images embracing social media applications, the study undertook the application of a Convolutional Neural Network (CNN), which was refined through transfer learning. The model’s performance was confirmed by its high accuracy levels of 92% for the thematic categories and 88% for the emotional content, together with Pearson coefficients for the comparison with human-coded benchmarks at r= 0. 84 for the emotional scores and r= 0. 79 for the thematic scores. The results suggest that such an approach is capable of recreating human decision-making processes with reasonable accuracy in the context of SEM interpretation relevant to digital media literacy, mental health, and marketing techniques. It also discusses the problems connected to ethical issues, confidentiality and anonymity of participants’ data preventing algorithmic bias and making it fair and inclusive in analyzing the data of the study. We consider certain limitations like selection bias and subjectivity of annotations; therefore, it suggests the future directions of the research like using larger scale and different types of data sets as well as using multimodal data. Therefore, this research validates the approach to synchronising image recognition technologies with psychological analysis theories for unpacking the complex psychological aspects of social media visuals. Thus, these methodologies will also be vital for the further advancement of research in the field of modern digital communication and the investigation of people’s interactions and emotions in this context.
Keywords:
Psychological analysis, Emotional content, Thematic classification, Social media, Image recognition.
References:
[1] Kunal Biswas et al., “Fuzzy and Genetic Algorithm Based Approach for Classification of Personality Traits Oriented Social Media Images,” Knowledge-Based Systems, vol. 241, 2022.
[CrossRef] [Google Scholar] [Publisher Link]
[2] Donghyuk Shin et al., “Enhancing Social Media Analysis with Visual Data Analytics: A Deep Learning Approach,” Management Information Systems Quarterly, vol. 44, no. 4, pp. 1459-1492, 2020.
[Google Scholar] [Publisher Link]
[3] Di Xue et al., “Deep Learning-Based Personality Recognition from Text Posts of Online Social Networks,” Applied Intelligence, vol. 48, no. 11, pp. 4232-4246, 2018.
[CrossRef] [Google Scholar] [Publisher Link]
[4] Mohammad Mahdi Dehshibi et al., “Vicsom: Visual Clues from Social Media for Psychological Assessment,” arXiv, 2019.
[CrossRef] [Google Scholar] [Publisher Link]
[5] Huijie Lin et al., “User-Level Psychological Stress Detection from Social Media Using Deep Neural Network,” Proceedings of the 22nd ACM International Conference on Multimedia, pp. 507-516, 2014.
[CrossRef] [Google Scholar] [Publisher Link]
[6] Anshu Malhotra, and Rajni Jindal, “Multimodal Deep Learning Based Framework for Detecting Depression and Suicidal Behaviour Study by Affective Analysis of Social Media Posts,” EAI Endorsed Transactions on Pervasive Health and Technology, vol. 6, no. 21, 2020.
[CrossRef] [Google Scholar] [Publisher Link]
[7] Daniel R. Richards, and Bige Tunçer, “Using Image Recognition to Automate the Assessment of Cultural Ecosystem Services from Social Media Photographs,” Ecosystem Services, vol. 31, pp. 318-325, 2018.
[CrossRef] [Google Scholar] [Publisher Link]
[8] Sebastian Scherr et al., “Detecting Intentional Self-Harm on Instagram: Development, Testing, and Validation of an Automatic Image-Recognition Algorithm to Discover Cutting-Related Posts,” Social Science Computer Review, vol. 38, no. 6, pp. 673-685, 2020.
[CrossRef] [Google Scholar] [Publisher Link]
[9] Lydia Manikonda, and Munmun De Choudhury, “Modeling and Understanding Visual Attributes of Mental Health Disclosures in Social Media,” Proceedings of the 2017 CHI Conference on Human Factors in Computing Systems, pp. 170-181, 2017.
[CrossRef] [Google Scholar] [Publisher Link]
[10] Suneet Gupta et al., “[Retracted] Homogeneous Decision Community Extraction Based on End-User Mental Behaviour on Social Media,” Computational Intelligence and Neuroscience, vol. 2022, 2022.
[CrossRef] [Google Scholar] [Publisher Link]
[11] Sicheng Zhao et al., “Predicting Personalized Image Emotion Perceptions in Social Networks,” IEEE Transactions on Affective Computing, vol. 9, no. 4, pp. 526-540, 2018.
[CrossRef] [Google Scholar] [Publisher Link]
[12] Irek Saitov et al., “Analysis of the Relationship between Studying the User’s Personality Traits and the Images they Post on Social Media,” Procedia Computer Science, vol. 193, pp. 155-162, 2021.
[CrossRef] [Google Scholar] [Publisher Link]
[13] Diana Ramírez-Cifuentes et al., “Detection of Suicidal Ideation on Social Media: Multimodal, Relational, and Behaviour Study Analysis,” Journal of Medical Internet Research, vol. 22, no. 7, 2020.
[CrossRef] [Google Scholar] [Publisher Link]
[14] Amir Hossein Yazdavar et al., “Multimodal Mental Health Analysis in Social Media,” Plos One, vol. 15, no. 4, 2020.
[CrossRef] [Google Scholar] [Publisher Link]
[15] Nirmal Varghese Babu, and E. Grace Mary Kanaga, “Sentiment Analysis in Social Media Data for Depression Detection Using Artificial Intelligence: A Review,” SN Computer Science, vol. 3, no. 1, 2022.
[CrossRef] [Google Scholar] [Publisher Link]
[16] Sicheng Zhao et al., “Affective Image Content Analysis: Two Decades Review and New Perspectives,” IEEE Transactions on Pattern Analysis and Machine Intelligence, vol. 44, no. 10, pp. 6729-6751, 2022.
[CrossRef] [Google Scholar] [Publisher Link]
[17] Leqi Liu et al., “Analyzing Personality through Social Media Profile Picture Choice,” Proceedings of the International AAAI Conference on Web and Social Media, vol. 10, no. 1, pp. 211-220, 2016.
[CrossRef] [Google Scholar] [Publisher Link]