Unveiling Precision in Abdominal Organ Segmentation: A Deep Dive into Emerging Deep Learning Paradigms for Single and Multi-modal Image Segmentation
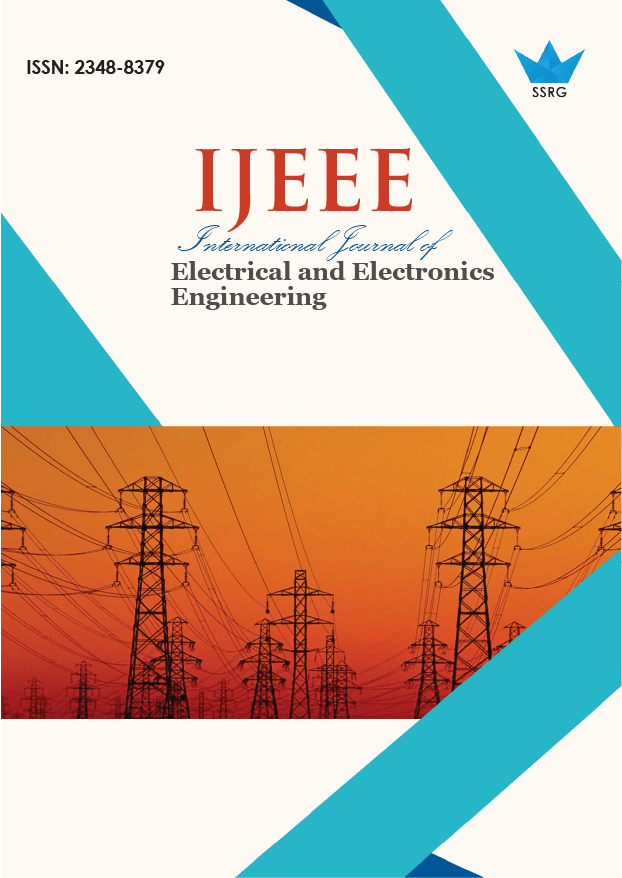
International Journal of Electrical and Electronics Engineering |
© 2024 by SSRG - IJEEE Journal |
Volume 11 Issue 10 |
Year of Publication : 2024 |
Authors : Snehal V. Laddha, Rohini S. Ochawar |
How to Cite?
Snehal V. Laddha, Rohini S. Ochawar, "Unveiling Precision in Abdominal Organ Segmentation: A Deep Dive into Emerging Deep Learning Paradigms for Single and Multi-modal Image Segmentation," SSRG International Journal of Electrical and Electronics Engineering, vol. 11, no. 10, pp. 10-21, 2024. Crossref, https://doi.org/10.14445/23488379/IJEEE-V11I10P102
Abstract:
Medical imaging is essential for diagnosing and managing diseases that impact human organs. Two widely used imaging techniques, Computed Tomography (CT) and Magnetic Resonance Imaging (MRI), offer valuable information about the structural aspects of organs. However, relying solely on a single imaging modality can sometimes limit disease detection accuracy. To address this limitation, the integration of multiple modalities followed by segmentation has gained traction, offering improved precision in assessing organ health. Accurate segmentation of organs from multi-modal medical images forms the cornerstone of modern healthcare, facilitating precise treatment planning, early disease detection, and personalized medicine. This paper offers an in-depth review of the latest trends and challenges in abdominal organ segmentation using deep learning approaches. It explores the use of attention mechanisms, Graph Neural Networks (GNNs) alongside traditional methods such as Convolutional Neural Networks (CNN), Fully Convolutional Networks (FCN), and Generative Adversarial Networks (GAN) within the scope of abdominal organ segmentation. The paper also addresses key challenges and opportunities in the field, highlighting the importance of continued innovation and collaboration to advance abdominal organ segmentation for improved clinical outcomes.
Keywords:
Deep learning, Multi-modal images, Segmentation, Abdominal organs, CT, MRI, Liver disease.
References:
[1] Simon R. Cherry, “Multimodality in Vivo Imaging Systems: Twice the Power or Double the Trouble?,” Annual Review of Biomedical Engineering, vol. 8, pp. 35-62, 2006.
[CrossRef] [Google Scholar] [Publisher Link]
[2] Ali Emre Kavur et al., CHAOS - Combined (CT-MR) Healthy Abdominal Organ Segmentation Challenge Data, Zenodo, 2019. [Online]. Available: https://zenodo.org/records/3431873
[3] Yang Lei et al., “Deep Learning in Multi-Organ Segmentation,” arXiv, 2001.
[CrossRef] [Google Scholar] [Publisher Link]
[4] U.S. Food & Drug Administration, Medical Imaging, 2018. [Online]. Available: https://www.fda.gov/radiation-emitting-products/radiation-emitting-products-and-procedures/medical-imaging
[5] Haoneng Lin et al., “Variance‐Aware Attention U‐Net for Multi‐Organ Segmentation,” Medical Physics, vol. 48, no. 12, pp. 7864-7876, 2021.
[CrossRef] [Google Scholar] [Publisher Link]
[6] Zhe Guo et al., “Medical Image Segmentation Based on the Multi-Modal Convolutional Neural Network: Study on Image Fusion Schemes,” 2018 IEEE 15th International Symposium on Biomedical Imaging (ISBI 2018), Washington, pp. 903-907, 2018.
[CrossRef] [Google Scholar] [Publisher Link]
[7] Vanya V. Valindria et al., “Small Organ Segmentation in Whole-Body MRI Using a Two-Stage FCN and Weighting Schemes,” Machine Learning in Medical Imaging, pp. 346-354, 2018.
[CrossRef] [Google Scholar] [Publisher Link]
[8] Olaf Ronneberger, Philipp Fischer, and Thomas Brox, “U-Net: Convolutional Networks for Biomedical Image Segmentation,” Proceedings of the International Conference on Medical Image Computing and Computer-Assisted Intervention, Munich, Germany, pp. 234-241, 2015.
[CrossRef] [Google Scholar] [Publisher Link]
[9] Holger R. Roth et al., “DeepOrgan: Multi-Level Deep Convolutional Networks for Automated Pancreas Segmentation,” Medical Image Computing and Computer-Assisted Intervention, pp. 556-564, 2015.
[CrossRef] [Google Scholar] [Publisher Link]
[10] William Thong et al., “Convolutional Networks for Kidney Segmentation in Contrast-Enhanced CT Scans,” Computer Methods in Biomechanics and Biomedical Engineering: Imaging & Visualization, vol. 6, no. 3, 2018.
[CrossRef] [Google Scholar] [Publisher Link]
[11] Jinzheng Cai et al., Pancreas Segmentation in MRI Using Graph-Based Decision Fusion on Convolutional Neural Networks, Medical Image Computing and Computer-Assisted Intervention, pp. 442‐450, 2016.
[CrossRef] [Google Scholar] [Publisher Link]
[12] Mans Larsson, Yuhang Zhang, and Fredrik Kahl, “Deepseg: Abdominal Organ Segmentation Using Deep Convolutional Neural Networks,” Swedish Symposium on Image Analysis, vol. 2016, 2016.
[Google Scholar] [Publisher Link]
[13] Peijun Hu et al., ‘Automatic Abdominal Multi-Organ Segmentation Using Deep Convolutional Neural Network and Time-Implicit Level Sets,” International Journal of Computer Assisted Radiology and Surgery, vol. 12, no. 3, pp. 399-411, 2016.
[CrossRef] [Google Scholar] [Publisher Link]
[14] Gabriel Efrain Humpire Mamani et al., “Organ Detection in Thorax Abdomen CT Using Multi-Label Convolutional Neural Networks,” Proceedings of the SPIE Medical Imaging 2017: Computer-Aided Diagnosis, vol. 10134, 2017.
[CrossRef] [Google Scholar] [Publisher Link]
[15] Xiaoming Liu et al., “Automatic Organ Segmentation for CT Scans Based on Super-Pixel and Convolutional Neural Networks,” Journal of Digital Imaging, vol. 31, pp. 748-760, 2016.
[CrossRef] [Google Scholar] [Publisher Link]
[16] Holger R. Roth et al., “A Multiscale Pyramid of 3D Fully Convolutional Networks for Abdominal Multi-organ Segmentation,” Medical Image Computing and Computer Assisted Intervention, pp. 417-425, 2018.
[CrossRef] [Google Scholar] [Publisher Link]
[17] Vanya V. Valindria et al., “Multi-Modal Learning from Unpaired Images: Application to Multi-organ Segmentation in CT and MRI,” IEEE Winter Conference on Applications of Computer Vision (WACV), USA, pp. 547-556, 2018.
[CrossRef] [Google Scholar] [Publisher Link]
[18] Ozan Oktay et al., “Attention U-Net: Learning Where to Look for the Pancreas,” arxiv, 2018.
[CrossRef] [Google Scholar] [Publisher Link]
[19] Nanyan Shen et al., “Multi-Organ Segmentation Network for Abdominal CT Images Based on Spatial Attention and Eformable Convolution,” Expert Systems with Applications, vol. 211, 2023.
[CrossRef] [Google Scholar] [Publisher Link]
[20] Hagar Louye Elghazy, and Mohamed Waleed Fakhr, “Dual‐and Triple‐Stream RESUNET/UNET Architectures for Multi‐Modal Liver Segmentation,” IET Image Processing, vol. 17, no. 4, pp. 1224-1235, 2023.
[CrossRef] [Google Scholar] [Publisher Link]
[21] Amit Aflalo et al., “Deepcut: Unsupervised Segmentation Using Graph Neural Networks Clustering,” Proceedings of the IEEE/CVF International Conference on Computer Vision workshops, pp. 32-41, 2023.
[Google Scholar] [Publisher Link]
[22] Juntao Jiang et al., “ViG-UNet: Vision Graph Neural Networks for Medical Image Segmentation,” IEEE 20th International Symposium on Biomedical Imaging (ISBI), Cartagena, Colombia, pp. 1-5, 2023.
[CrossRef] [Google Scholar] [Publisher Link]
[23] Si Zhang et al., “Graph Convolutional Networks: A Comprehensive Review,” Computational Social Networks, vol. 6, 2019.
[CrossRef] [Google Scholar] [Publisher Link]
[24] Hongyang Gao, and Shuiwang Ji, “Graph U-Nets,” International Conference on Machine Learning Research, pp. 2083-2092, 2019.
[Google Scholar] [Publisher Link]
[25] Shima Rafiei et al., “Liver Segmentation in CT Images Using Three-Dimensional to Two-Dimensional Fully Convolutional Network,” 25th IEEE International Conference on Image Processing (ICIP), Athens, Greece, pp. 2067-2071, 2018.
[CrossRef] [Google Scholar] [Publisher Link]
[26] Hideki Kakeya, Toshiyuki Okada, and Yukio Oshiro, “3D U-JAPA-Net: Mixture of Convolutional Networks for Abdominal Multi-Organ CT Segmentation,” Medical Image Computing and Computer Assisted Intervention, pp. 426-433, 2018.
[CrossRef] [Google Scholar] [Publisher Link]
[27] Eli Gibson et al., “NiftyNet: A Deep-Learning Platform for Medical Imaging, Computer Methods and Programs in Biomedicine,” Computer Methods and Programs in Biomedicine, vol. 158, pp. 113-122, 2018.
[CrossRef] [Google Scholar] [Publisher Link]
[28] Eli Gibson et al., “Automatic Multi-Organ Segmentation on Abdominal CT with Dense V-Networks,” IEEE Transactions on Medical Imaging, vol. 37, no. 8, pp. 1822-1834, 2018.
[CrossRef] [Google Scholar] [Publisher Link]
[29] Mubashir Ahmad et al., “Deep Belief Network Modeling for Automatic Liver Segmentation,” IEEE Access, vol. 7, pp. 20585-20595, 2019.
[CrossRef] [Google Scholar] [Publisher Link]
[30] Yucheng Tang et al., “Improving Splenomegaly Segmentation by Learning from Heterogeneous Multi-Source Labels,” Proceedings of SPIE - The International Society for Optical Engineering, 2019.
[CrossRef] [Google Scholar] [Publisher Link]
[31] Minyoung Chung et al., “Deeply Self-Supervised Contour Embedded Neural Network Applied to Liver Segmentation,” Computer Methods and Programs in Biomedicine, vol. 192, 2020.
[CrossRef] [Google Scholar] [Publisher Link]
[32] Yuyin Zhou et al., “Semi-Supervised 3D Abdominal Multi-Organ Segmentation via Deep Multi-Planar Co-Training,” IEEE Winter Conference on Applications of Computer Vision (Wacv), pp. 121-140, 2019.
[CrossRef] [Google Scholar] [Publisher Link]
[33] Nuo Tong, et al., “Self-Paced DenseNet with Boundary Constraint for Automated Multi-Organ Segmentation on Abdominal CT Images,” Physics in Medicine & Biology, vol. 65, no. 13, 2020.
[CrossRef] [Google Scholar] [Publisher Link]
[34] Minfeng Xu et al., “A New Probabilistic V-Net Model with Hierarchical Spatial Feature Transform for Efficient Abdominal Multi-Organ Segmentation,” arXiv, 2022.
[CrossRef] [Google Scholar] [Publisher Link]
[35] Beyza Kayhan, and Sait Ali Uymaz, “Abdominal Multi-Organ Segmentation Using 3D Unet Based on Early Fusion Mechanism,” SSRN, 2022.
[CrossRef] [Google Scholar] [Publisher Link]
[36] Moritz Gross et al., “Automated MRI Liver Segmentation for Anatomical Segmentation, Liver Volumetry, and the Extraction of Radiomics,” European Radiology, vol. 34, pp. 5056-5065, 2024.
[CrossRef] [Google Scholar] [Publisher Link]
[37] Meg F. Bobo et al., “Fully Convolutional Neural Networks Improve Abdominal Organ Segmentation,” Proceedings of the SPIE Medical Imaging 2018: Image Processing, vol. 10574, 2018.
[CrossRef] [Google Scholar] [Publisher Link]
[38] Supriti Mulay et al., “Liver Segmentation from Multi-modal Images Using HED-Mask R-CNN,” Multiscale Multi-modal Medical Imaging, pp. 68-75, 2019.
[CrossRef] [Google Scholar] [Publisher Link]
[39] Zongwei Zhou et al., “UNet++: Redesigning Skip Connections to Exploit Multiscale Features in Image Segmentation,” IEEE Transactions on Medical Imaging, vol. 39, no. 6, pp. 1856-1867, 2020.
[CrossRef] [Google Scholar] [Publisher Link]
[40] Pierre-Henri Conze et al., “Abdominal Multi-Organ Segmentation with Cascaded Convolutional and Adversarial Deep Networks,” Artificial Intelligence in Medicine, vol. 117, 2021.
[CrossRef] [Google Scholar] [Publisher Link]
[41] Pauline Luc et al., “Semantic Segmentation Using Adversarial Networks,” arXiv, 2016.
[CrossRef] [Google Scholar] [Publisher Link]