A Comprehensive Analysis of 5G Network Deployment Using Machine Learning Techniques
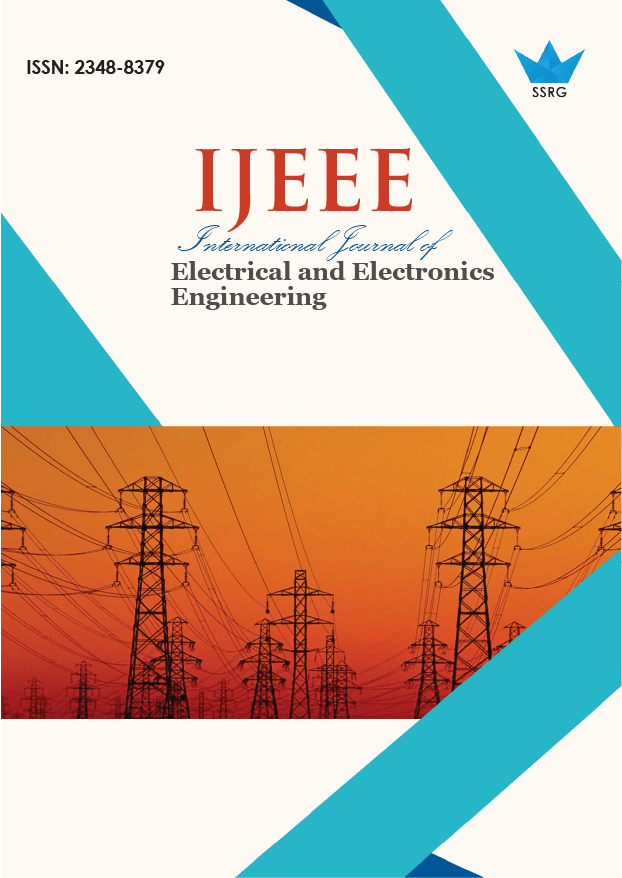
International Journal of Electrical and Electronics Engineering |
© 2024 by SSRG - IJEEE Journal |
Volume 11 Issue 10 |
Year of Publication : 2024 |
Authors : Abdijalil Abdullahi, Abdikadir Hussein Elmi, Mohamed Ali Bare |
How to Cite?
Abdijalil Abdullahi, Abdikadir Hussein Elmi, Mohamed Ali Bare, "A Comprehensive Analysis of 5G Network Deployment Using Machine Learning Techniques," SSRG International Journal of Electrical and Electronics Engineering, vol. 11, no. 10, pp. 22-29, 2024. Crossref, https://doi.org/10.14445/23488379/IJEEE-V11I10P103
Abstract:
In the realm of telecommunication, the deployment of 5G networks is pivotal for realizing the full potential of high-speed connectivity and the Internet of Things (IoT). This paper explores the application of Machine Learning (ML) techniques in analyzing and predicting the success of 5G network deployments. Through a comprehensive dataset encompassing deployment statuses, types, and operator profiles, we applied ML algorithms to interpret current trends and project future deployment outcomes. Our comparative analysis between Logistics Regression and Random Forest models two prominent ML approacheshighlights their respective predictive performances. Logistics Regression demonstrated exceptional proficiency, with an accuracy of 99.85%, Precision of 99.85%, recall at an almost perfect 99.99%, and an F1 Score of 99.92%. Meanwhile, the Random Forest model, upon adjustment, showed respectable results with an accuracy of 89.00%, precision of 87.00%, recall of 91.00%, and an F1 score matching its accuracy. These findings suggest that Logistics Regression may offer a more reliable predictive model for 5G deployment in various contexts, although Random Forest models have their merits in handling complex interactions. The study's outcomes provide valuable insights for telecom stakeholders aiming to optimize 5G network rollout strategies and reinforce the decision-making processes with robust data-driven support.
Keywords:
5G, Predicting, Machine Learning, Analysis, Logistics Regression, Random Forest.
References:
[1] Haris Haskić, and Amina Radončić, “The Effects of 5G Network on People and the Environment: A Machine Learning Approach to the Comprehensive Analysis,” World Journal of Advanced Engineering Technology and Sciences, vol. 11, no. 1, pp. 301-309, 2024.
[CrossRef] [Google Scholar] [Publisher Link]
[2] Amna Mughees et al., “Towards Energy Efficient 5G Networks Using Machine Learning: Taxonomy, Research Challenges, and Future Research Directions,” IEEE Access, vol. 8, pp. 187498-187522, 2020.
[CrossRef] [Google Scholar] [Publisher Link]
[3] Greeshma Arya, Ashish Bagwari, and Durg Singh Chauhan, “Performance Analysis of Deep Learning-Based Routing Protocol for an Efficient Data Transmission in 5G WSN Communication,” IEEE Access, vol. 10, pp. 9340-9356, 2022.
[CrossRef] [Google Scholar] [Publisher Link]
[4] Diogo Ferreira et al., “A Forecasting Approach to Improve Control and Management for 5G Networks,” IEEE Transactions on Network and Service Management, vol. 18, no. 2, pp. 1817-1831, 2021.
[CrossRef] [Google Scholar] [Publisher Link]
[5] Mohd Fazuwan Ahmad Fauzi et al., “Mobile Network Coverage Prediction Based on Supervised Machine Learning Algorithms,” IEEE Access, vol. 10, pp. 55782-55793, 2022.
[CrossRef] [Google Scholar] [Publisher Link]
[6] Ioannis A. Bartsiokas et al., “ML-Based Radio Resource Management in 5G and Beyond Networks: A Survey,” IEEE Access, vol. 10, pp. 83507-83528, 2022.
[CrossRef] [Google Scholar] [Publisher Link]
[7] M. Majid Butt, Anna Pantelidou, and István Z. Kovács, “ML-Assisted UE Positioning: Performance Analysis and 5G Architecture Enhancements,” IEEE Open Journal of Vehicular Technology, vol. 2, pp. 377-388, 2021.
[CrossRef] [Google Scholar] [Publisher Link]
[8] Yu Fu et al., “Artificial Intelligence to Manage Network Traffic of 5G Wireless Networks,” IEEE Network, vol. 32, no. 6, pp. 58-64, 2018.
[CrossRef] [Google Scholar] [Publisher Link]
[9] Imad Alawe et al., “Improving Traffic Forecasting for 5G Core Network Scalability: A Machine Learning Approach,” IEEE Network, vol. 32, no. 6, pp. 42-49, 2018.
[CrossRef] [Google Scholar] [Publisher Link]
[10] Helin Yang, Xianzhong Xie, and Michel Kadoch, “Machine Learning Techniques and A Case Study for Intelligent Wireless Networks,” IEEE Network, vol. 34, no. 3, pp. 208-215, 2020.
[CrossRef] [Google Scholar] [Publisher Link]
[11] Dario Bega et al., “A Machine Learning Approach to 5G Infrastructure Market Optimization,” IEEE Transactions on Mobile Computing, vol. 19, no. 3, pp. 498-512, 2020.
[CrossRef] [Google Scholar] [Publisher Link]
[12] Jani Suomalainen et al., “Machine Learning Threatens 5G Security,” IEEE Access, vol. 8, pp. 190822-190842, 2020.
[CrossRef] [Google Scholar] [Publisher Link]
[13] Jasneet Kaur et al., “Machine Learning Techniques for 5G and Beyond,” IEEE Access, vol. 9, pp. 23472-23488, 2021.
[CrossRef] [Google Scholar] [Publisher Link]
[14] Manuel Eugenio Morocho-Cayamcela, Haeyoung Lee, and Wansu Lim, “Machine Learning for 5G/B5G Mobile and Wireless Communications: Potential, Limitations, and Future Directions,” IEEE Access, vol. 7, pp. 137184-137206, 2019.
[CrossRef] [Google Scholar] [Publisher Link]
[15] Salih Sevgican et al., “Intelligent Network Data Analytics Function in 5G Cellular Networks Using Machine Learning,” Journal of Communications and Networks, vol. 22, no. 3, pp. 269-280, 2020.
[CrossRef] [Google Scholar] [Publisher Link]
[16] Rakibul Islam Rony, Elena Lopez-Aguilera, and Eduard Garcia-Villegas, “Dynamic Spectrum Allocation Following Machine Learning-Based Traffic Predictions in 5G,” IEEE Access, vol. 9, pp. 143458-143472, 2021.
[CrossRef] [Google Scholar] [Publisher Link]
[17] Anurag Thantharate et al., “DeepSlice: A Deep Learning Approach towards an Efficient and Reliable Network Slicing in 5G Networks,” 2019 IEEE 10th Annual Ubiquitous Computing, Electronics & Mobile Communication Conference (UEMCON), New York, USA, pp. 762-767, 2019.
[CrossRef] [Google Scholar] [Publisher Link]
[18] Bouziane Brik, Karim Boutiba, and Adlen Ksentini, “Deep Learning for B5G Open Radio Access Network: Evolution, Survey, Case Studies, and Challenges,” IEEE Open Journal of the Communications Society, vol. 3, pp. 228-250, 2022.
[CrossRef] [Google Scholar] [Publisher Link]
[19] Shailendra Rathore, Jong Hyuk Park, and Hangbae Chang, “Deep Learning and Blockchain-Empowered Security Framework for Intelligent 5G-Enabled IoT,” IEEE Access, vol. 9, pp. 90075-90083, 2021.
[CrossRef] [Google Scholar] [Publisher Link]
[20] Hajiar Yuliana, Iskandar, and Hendrawan, “Comparative Analysis of Machine Learning Algorithms for 5G Coverage Prediction: Identification of Dominant Feature Parameters and Prediction Accuracy,” IEEE Access, vol. 12, pp. 18939-18956, 2024.
[CrossRef] [Google Scholar] [Publisher Link]
[21] Susanna Schwarzmann et al., “ML-Based QoE Estimation in 5G Networks Using Different Regression Techniques,” IEEE Transactions on Network and Service Management, vol. 19, no. 3, pp. 3516-3532, 2022.
[CrossRef] [Google Scholar] [Publisher Link]
[22] S. Fan et al., “A Full-Scale Field Study for Evaluation of Simple Analytical Models of Cross Ventilation and Single-Sided Ventilation,” Building and Environment, vol. 187, 2020.
[CrossRef] [Google Scholar] [Publisher Link]
[23] Luong-Vy Le, Bao-Shuh Lin, and Sinh Do, “Applying Big Data, Machine Learning, and SDN/NFV for 5G Early-Stage Traffic Classification and Network QoS Control,” Transactions on Networks and Communications, vol. 6, no. 2, pp. 36-50, 2018. [CrossRef] [Google Scholar] [Publisher Link]
[24] Yaser Azimi et al., “Applications of Machine Learning in Resource Management for RAN-Slicing in 5G and Beyond Networks: A Survey,” IEEE Access, vol. 10, pp. 106581-106612, 2022.
[CrossRef] [Google Scholar] [Publisher Link]
[25] Hassan A. Alamr, Vijey Thayananthan, and Javad Yazdani, “Machine Learning for Securing SDN Based 5G Network,” International Journal of Computer Applications, vol. 174, no. 14, pp. 9-16, 2021.
[CrossRef] [Google Scholar] [Publisher Link]
[26] Alexandros Kaloxylos et al., “AI and ML - Enablers for Beyond 5G Networks,” Zenodo, 2021.
[CrossRef] [Google Scholar] [Publisher Link]
[27] Konstantinos Samdanis et al., “AI / ML Service Enablers & Model Maintenance for Beyond 5G Networks,” IEEE Network, vol. 37, no. 5, pp. 162-172, 2023.
[CrossRef] [Google Scholar] [Publisher Link]
[28] Parjanay Sharma et al., “Role of Machine Learning and Deep Learning in Securing 5G-Driven Industrial IoT Applications,” Ad Hoc Networks, vol. 123, 2021.
[CrossRef] [Google Scholar] [Publisher Link]
[29] Bo Ma, Weisi Guo, and Jie Zhang, “A Survey of Online Data-Driven Proactive 5G Network Optimisation Using Machine Learning,” IEEE Access, vol. 8, pp. 35606-35637, 2020.
[CrossRef] [Google Scholar] [Publisher Link]
[30] Michael S. Mollel et al., “A Survey of Machine Learning Applications to Handover Management in 5G and Beyond,” IEEE Access, vol. 9, pp. 45770-45802, 2021.
[CrossRef] [Google Scholar] [Publisher Link]
[31] M. Rezwanul Mahmood et al., “A Comprehensive Review on Artificial Intelligence/Machine Learning Algorithms for Empowering the Future IoT Toward 6G Era,” IEEE Access, vol. 10, pp. 87535-87562, 2022.
[CrossRef] [Google Scholar] [Publisher Link]
[32] Luong-Vy Le et al., “Applying Big Data, Machine Learning, and SDN/NFV to 5G Traffic Clustering, Forecasting, and Management,” 2018 4th IEEE Conference on Network Softwarization and Workshops (NetSoft), Montreal, Canada, pp. 168-176, 2018.
[CrossRef] [Google Scholar] [Publisher Link]
[33] Hitesh Keserwani et al., “Security Enhancement by Identifying Attacks Using Machine Learning for 5G Network,” International Journal of Communication Networks and Information Security (IJCNIS), vol. 14, no. 2, pp. 124-141, 2022.
[CrossRef] [Google Scholar] [Publisher Link]
[34] Tanujay Saha, Najwa Aaraj, and Niraj K. Jha, “Machine Learning Assisted Security Analysis of 5G-Network-Connected Systems,” IEEE Transactions on Emerging Topics in Computing, vol. 10, no. 4, pp. 2006-2024, 2022.
[CrossRef] [Google Scholar] [Publisher Link]
[35] David López-Pérez et al., “A Survey on 5G Radio Access Network Energy Efficiency: Massive MIMO, Lean Carrier Design, Sleep Modes, and Machine Learning,” IEEE Communications Surveys & Tutorials, vol. 24, no. 1, pp. 653-697, 2022.
[CrossRef] [Google Scholar] [Publisher Link]
[36] Muhammad Usama et al., “Examining Machine Learning for 5G and beyond through an Adversarial Lens,” IEEE Internet Computing, vol. 25, no. 2, pp. 26-34, 2021.
[CrossRef] [Google Scholar] [Publisher Link]
[37] Noman Haider, Muhammad Zeeshan Baig, and Muhammad Imran, “Artificial Intelligence and Machine Learning in 5G Network Security: Opportunities, Advantages, and Future Research Trends,” arXiv, pp. 1-7, 2020.
[CrossRef] [Google Scholar] [Publisher Link]
[38] Jordan Lam, and Robert Abbas, “Machine Learning Based Anomaly Detection for 5G Networks,” arXiv, pp. 1-12, 2020.
[CrossRef] [Google Scholar] [Publisher Link]
[39] Cheng-Xiang Wang et al., “Artificial Intelligence Enabled Wireless Networking for 5G and Beyond: Recent Advances and Future Challenges,” IEEE Wireless Communications, vol. 27, no. 1, pp. 16-23, 2020.
[CrossRef] [Google Scholar] [Publisher Link]
[40] Hongji Huang et al., “Deep Learning for Physical-Layer 5G Wireless Techniques: Opportunities, Challenges and Solutions,” IEEE Wireless Communications, vol. 27, no. 1, pp. 214-222, 2020.
[CrossRef] [Google Scholar] [Publisher Link]
[41] Jessica Moysen, and Lorenza Giupponi, “From 4G to 5G: Self-Organized Network Management Meets Machine Learning,” Computer Communications, vol. 129, pp. 248-268, 2018.
[CrossRef] [Google Scholar] [Publisher Link]
[42] Madanagopal Ramachandran et al., “5G Network Management System with Machine Learning Based Analytics,” IEEE Access, vol. 10, pp. 73610-73622, 2022.
[CrossRef] [Google Scholar] [Publisher Link]
[43] Nazih Salhab et al., “Machine Learning Based Resource Orchestration for 5G Network Slices,” 2019 IEEE Global Communications Conference (GLOBECOM), Waikoloa, HI, USA, pp. 1-6, 2019.
[CrossRef] [Google Scholar] [Publisher Link]
[44] A. Abdellah, and A. Koucheryavy, “Survey on Artificial Intelligence Techniques in 5G Networks,” Telecom IT, vol. 8, no. 1, pp. 1-10, 2020.
[CrossRef] [Google Scholar] [Publisher Link]