Drowsiness Detection System in Drivers Using Micro-Maneuvers on the Steering Wheel and Machine Learning to Prevent Nighttime Traffic Accidents
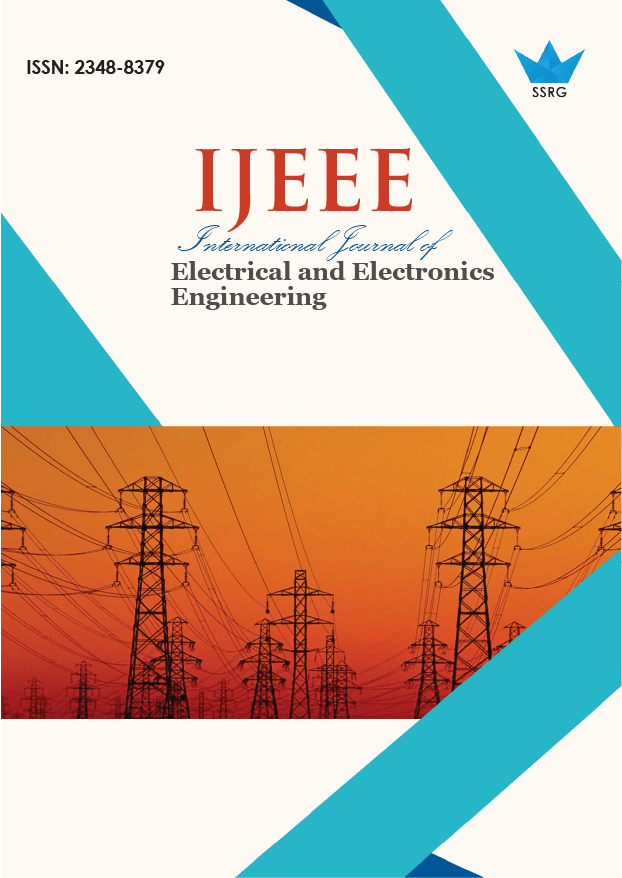
International Journal of Electrical and Electronics Engineering |
© 2024 by SSRG - IJEEE Journal |
Volume 11 Issue 11 |
Year of Publication : 2024 |
Authors : Charly Alvarez Heredia, Freddy Jamer Alvarez Huacasi, Yunior Edwin Vargas Apfata, Jesús Talavera Suarez |
How to Cite?
Charly Alvarez Heredia, Freddy Jamer Alvarez Huacasi, Yunior Edwin Vargas Apfata, Jesús Talavera Suarez, "Drowsiness Detection System in Drivers Using Micro-Maneuvers on the Steering Wheel and Machine Learning to Prevent Nighttime Traffic Accidents," SSRG International Journal of Electrical and Electronics Engineering, vol. 11, no. 11, pp. 114-121, 2024. Crossref, https://doi.org/10.14445/23488379/IJEEE-V11I11P112
Abstract:
Traditional techniques to identify drowsiness, such as physiological sensors and camera-based eye tracking, often encounter difficulties in practice due to their intrusiveness, cost and vulnerability to other influences. This paper presents a novel non-invasive sleep detection system that accurately detects driver sleep indicators by combining machine learning approaches with driving micro-maneuvers. This method uses high-precision turning data obtained from sensors embedded in the vehicle's steering wheel. The system uses this data to extract characteristics related to driver drowsiness, relating small variations in the turning of the steering wheel, which, depending on whether they are present or not, determine the driver's state and classify it as "alert" or "drowsy" thanks to the use of sophisticated autonomic learning techniques, such as Support Vector Machines (SVM) and Convolutional Neural Networks (CNN). In order to validate the system, several tests were performed in controlled scenarios, with various drivers with different states of drowsiness previously verified. The tests showed that the proposed system obtained an overall accuracy rate of more than 92%, which allows it to be used in real scenarios without discomfort to the driver as it is a non-invasive measurement technique.
Keywords:
Drowsiness detection system, Micro-maneuvers on the Steering wheel, Machine Learning, SVM, CNN.
References:
[1] World Health Organization, Global Status Report on Road Safety 2023, 2023. [Online]. Available: https://www.who.int/publications/i/item/9789240086517
[2] R. Grace et al., “A Drowsy Driver Detection System for Heavy Vehicles,” Proceedings of the 17th Digital Avionics Systems Conference, USA, vol. 2, pp. 136/1-136/8, 1998.
[CrossRef] [Google Scholar] [Publisher Link]
[3] H. Ueno, M. Kaneda, and M. Tsukino, “Development of Drowsiness Detection System,” Proceedings of Vehicle Navigation and Information Systems Conference, pp. 15-20, 1994.
[CrossRef] [Google Scholar] [Publisher Link]
[4] J.A. Healey, and R.W. Picard, “Detecting Stress During Real-World Driving Tasks Using Physiological Sensors,” IEEE Transactions on Intelligent Transportation Systems, vol. 6, no. 2, pp. 156-166, 2005.
[CrossRef] [Google Scholar] [Publisher Link]
[5] Arun Sahayadhas, Kenneth Sundaraj, and Murugappan Murugappan, “Detecting Driver Drowsiness Based on Sensors: A Review,” Sensors, vol. 12, no. 12, pp. 16937-16953, 2012.
[CrossRef] [Google Scholar] [Publisher Link]
[6] A.V. Desai, and M.A. Haque, “Vigilance Monitoring for Operator Safety: A Simulation Study on Highway Driving,” Journal of Safety Research, vol. 37, no. 2, pp. 139-147, 2006.
[CrossRef] [Google Scholar] [Publisher Link]
[7] Meng Zhang, and Fumin Zhang, “A Driver Fatigue Detection Method Based on Eye Aspect Ratio Compensated by Head Pose Estimation,” 2023 8th International Conference on Signal and Image Processing (ICSIP), Wuxi, China, pp. 161-165, 2023. [CrossRef] [Google Scholar] [Publisher Link]
[8] Budi Thomas Jap et al., “Using EEG Spectral Components to Assess Algorithms for Detecting Fatigue,” Expert Systems with Applications, vol. 36, no. 2, pp. 2352-2359, 2009.
[CrossRef] [Google Scholar] [Publisher Link]
[9] Rony Almiron et al., “Preventive Detection of Driver Drowsiness from EEG Signals Using Fuzzy Expert Systems,” Revista Mexicana De Ingenieria Biomedica, vol. 45, no. 1, pp. 6-20, 2024.
[CrossRef] [Google Scholar] [Publisher Link]
[10] Pengbo Zhao et al., “Driving Cognitive Alertness Detecting Using Evoked Multimodal Physiological Signals Based on Uncertain Self-Supervised Learning,” IEEE Transactions on Neural Systems and Rehabilitation Engineering, vol. 32, pp. 2165-2176, 2024.
[CrossRef] [Google Scholar] [Publisher Link]
[11] Rohit Gusain, and Nancy Kansal, “Drowsiness Alertness for Driver Safety,” 2022 1st International Conference on Computational Science and Technology (ICCST), pp. 424-428, 2022.
[CrossRef] [Google Scholar] [Publisher Link]
[12] Federico Del Pup, and Manfredo Atzori, “Applications of Self-Supervised Learning to Biomedical Signals: A Survey,” IEEE Access, vol. 11, pp. 144180-144203, 2023.
[CrossRef] [Google Scholar] [Publisher Link]
[13] Anuva Chowdhury et al., “Sensor Applications and Physiological Features in Drivers’ Drowsiness Detection: A Review,” IEEE Sensors Journal, vol. 18, no. 8, pp. 3055-3067, 2018.
[CrossRef] [Google Scholar] [Publisher Link]
[14] Yang Song et al., “A Spatial Coupling Model to Study Dynamic Performance of Pantograph-Catenary with Vehicle-Track Excitation,” Mechanical Systems and Signal Processing, vol. 151, 2021.
[CrossRef] [Google Scholar] [Publisher Link]
[15] Dohun Kim et al., “Real-Time Driver Monitoring System with Facial Landmark-Based Eye Closure Detection and Head Pose Recognition,” Scientific Reports, vol. 13, 2023.
[CrossRef] [Google Scholar] [Publisher Link]
[16] Muhammad Awais, Nasreen Badruddin, and Micheal Drieberg, “A Hybrid Approach to Detect Driver Drowsiness Utilizing Physiological Signals to Improve System Performance and Wearability,” Sensors, vol. 17, no. 9, 2017.
[CrossRef] [Google Scholar] [Publisher Link]
[17] Islam A. Fouad, “A Robust and Efficient EEG-Based Drowsiness Detection System Using Different Machine Learning Algorithms,” Ain Shams Engineering Journal, vol. 14, no. 3, 2023.
[CrossRef] [Google Scholar] [Publisher Link]
[18] Ruben Florez et al., “A CNN-Based Approach for Driver Drowsiness Detection by Real-Time Eye State Identification,” Applied Sciences, vol. 13, no. 13, 2023.
[CrossRef] [Google Scholar] [Publisher Link]