DL-Enhanced GAIT Analysis for Rehabilitation: A Comprehensive Survey
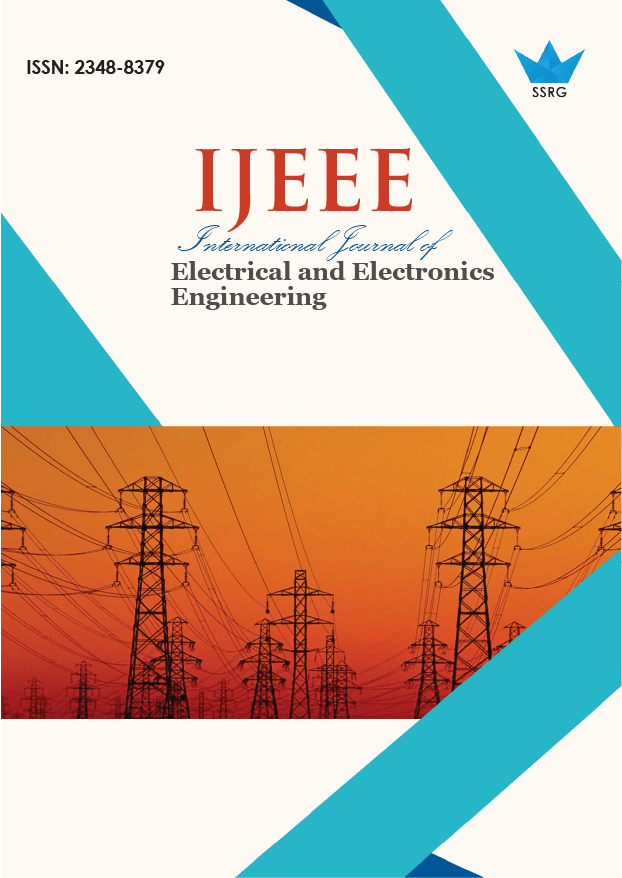
International Journal of Electrical and Electronics Engineering |
© 2024 by SSRG - IJEEE Journal |
Volume 11 Issue 11 |
Year of Publication : 2024 |
Authors : O. Pushpalatha, R. Premkumar |
How to Cite?
O. Pushpalatha, R. Premkumar, "DL-Enhanced GAIT Analysis for Rehabilitation: A Comprehensive Survey," SSRG International Journal of Electrical and Electronics Engineering, vol. 11, no. 11, pp. 203-221, 2024. Crossref, https://doi.org/10.14445/23488379/IJEEE-V11I11P121
Abstract:
Integrating DL techniques has revolutionized gait analysis, enhancing the accuracy and efficiency of detecting and characterizing gait abnormalities. This paper surveys recent studies employing Deep Learning algorithms (DL), such as Convolutional Neural Networks (CNNs) and Recurrent Neural Networks (RNNs), to analyze gait patterns from diverse data sources with wearable sensors, video footage, and motion capture systems. The advantages of DL in handling complex, high-dimensional gait data and its potential to uncover subtle patterns indicative of disease or recovery status are discussed. Furthermore, the clinical applications of DL-based gait analysis, emphasizing its role in personalized rehabilitation programs and real-time monitoring, are explored. The paper also addresses the challenges of implementing DL in clinical settings, such as the need for large, annotated datasets, computational resources, and interdisciplinary collaboration. In conclusion, this survey highlights the transformative potential of DL methods in gait analysis for fracture and Parkinson's disease patients. By providing a detailed overview of current research and identifying key trends and challenges, this work seems to inform and inspire further advancements in this field, ultimately enhancing rehabilitation outcomes and quality of life for affected individuals.
Keywords:
CNN, DL techniques, Gait analysis, RNN.
References:
[1] Chee Hong Koh, “Development of Classification Algorithms of Human Gait,” Doctoral Dissertation, UTAR, 2022.
[Google Scholar] [Publisher Link]
[2] Yogesh Singh, and Vineet Vashista, “Gait Classification with Gait Inherent Attribute Identification from Ankle’s Kinematics,” IEEE Transactions on Neural Systems and Rehabilitation Engineering, vol. 30, pp. 833-842, 2022.
[CrossRef] [Google Scholar] [Publisher Link]
[3] Lazzaro di Biase et al., “Gait Analysis in Parkinson’s Disease: An Overview of the Most Accurate Markers for Diagnosis and Symptoms Monitoring,” Sensors, vol. 20, no. 12, 2020.
[CrossRef] [Google Scholar] [Publisher Link]
[4] Dimple Sethi, Chandra Prakash, Sourabh Bharti, “Multi-Feature Gait Analysis Approach Using Deep Learning in Constraint-Free Environment,” Expert Systems, vol. 41, no. 7, 2024.
[CrossRef] [Google Scholar] [Publisher Link]
[5] Christina Salchow-Hömmen et al., “Emerging Portable Technologies for Gait Analysis in Neurological Disorders,” Frontiers in Human Neuroscience, vol. 16, 2022.
[CrossRef] [Google Scholar] [Publisher Link]
[6] Steffi L. Colyer et al., “A Review of the Evolution of Vision-Based Motion Analysis and the Integration of Advanced Computer Vision Methods Toward Developing a Marker Less System,” Sports Medicine-Open, vol. 4, 2018.
[CrossRef] [Google Scholar] [Publisher Link]
[7] Alvaro Muro-de-la-Herran, Begonya Garcia-Zapirain, and Amaia Mendez-Zorrilla, “Gait Analysis Methods: An Overview of Wearable and Non-Wearable Systems, Highlighting Clinical Applications,” Sensors, vol. 14, no. 2, pp. 3362-3394, 2014.
[CrossRef] [Google Scholar] [Publisher Link]
[8] Rijun Liao et al., “PoseMapGait: A Model-Based Gait Recognition Method with Pose Estimation Maps and Graph Convolutional Networks,” Neurocomputing, vol. 501, pp. 514-528, 2022.
[CrossRef] [Google Scholar] [Publisher Link]
[9] Timothy McGrath, and Leia Stirling, “Body-Worn IMU Human Skeletal Pose Estimation Using a Factor Graph-Based Optimization Framework,” Sensors, vol. 20, no. 23, 2020.
[CrossRef] [Google Scholar] [Publisher Link]
[10] Weizhi An et al., “Performance Evaluation of Model-Based Gait on Multi-View Very Large Population Database with Pose Sequences,” IEEE Transactions on Biometrics, Behavior, and Identity Science, vol. 2, no. 4, pp. 421-430, 2020.
[CrossRef] [Google Scholar] [Publisher Link]
[11] Rijun Liao et al., “A Model-Based Gait Recognition Method with Body Pose and Human Prior Knowledge,” Pattern Recognition, vol. 98, 2020.
[CrossRef] [Google Scholar] [Publisher Link]
[12] Hanqing Chao et al., “Gait Set: Cross-View Gait Recognition through Utilizing Gait as a Deep Set,” IEEE Transactions on Pattern Analysis and Machine Intelligence, vol. 44, no. 7, pp. 3467-3478, 2021.
[CrossRef] [Google Scholar] [Publisher Link]
[13] Angel Peinado-Contreras, and Mario Munoz-Organero, “Gait-Based Identification Using Deep Recurrent Neural Networks and Acceleration Patterns,” Sensors, vol. 20, no. 23, 2020.
[CrossRef] [Google Scholar] [Publisher Link]
[14] Maria De Marsico, and Alessio Mecca, “A Survey on Gait Recognition via Wearable Sensors,” ACM Computing Surveys, vol. 52, no. 4, pp. 1-39, 2019.
[CrossRef] [Google Scholar] [Publisher Link]
[15] Jasvinder Pal Singh et al., “Vision-Based Gait Recognition: A Survey,” IEEE Access, vol. 6, pp. 70497-70527, 2018.
[CrossRef] [Google Scholar] [Publisher Link]
[16] Pa Pa Min, Shohel Sayeed, and Thian Song Ong, “Gait Recognition Using Deep Convolutional Features,” 2019 7th International Conference on Information and Communication Technology (ICoICT), Kuala Lumpur, Malaysia, pp. 1-5, 2019.
[CrossRef] [Google Scholar] [Publisher Link]
[17] An-Bang Liu, and Che-Wei Lin, “Multiscale Approximate Entropy for Gait Analysis in Patients with Neurodegenerative Diseases,” Entropy, vol. 21, no. 10, 2019.
[CrossRef] [Google Scholar] [Publisher Link]
[18] Saif M.J. Haider et al., “Simulation of the Gait Cycle in the Sagittal Plane for an Above-Knee Prosthesis,” AIP Conference Proceedings, vol. 2386, no. 1, 2022.
[CrossRef] [Google Scholar] [Publisher Link]
[19] Nikolaos V. Boulgouris, and Zhiwei X. Chi, “Gait Recognition Using Radon Transform and Linear Discriminant Analysis,” IEEE Transactions on Image Processing,” vol. 16, no. 3, pp. 731-740, 2007.
[CrossRef] [Google Scholar] [Publisher Link]
[20] Imran Ali, “New Generation Adsorbents for Water Treatment,” Chemical Reviews, vol. 112, no. 10, pp. 5073-5091, 2012.
[CrossRef] [Google Scholar] [Publisher Link]
[21] Tanmay T. Verlekar, Luís D. Soares, and Paulo L. Correia, “Automatic Classification of Gait Impairments Using a Marker Less 2D Video-Based System,” Sensors, vol. 18, no. 9, 2018.
[CrossRef] [Google Scholar] [Publisher Link]
[22] Elif Surer, and Alper Kose, “Methods and Technologies for Gait Analysis,” Computer Analysis of Human Behavior, pp. 105-123, 2011.
[CrossRef] [Google Scholar] [Publisher Link]
[23] Chandra Prakash, Rajesh Kumar, and Namita Mittal, “Recent Developments in Human Gait Research: Parameters, Approaches, Applications, Machine Learning Techniques, Datasets, and Challenges,” Artificial Intelligence Review, vol. 49, pp. 1-40, 2018.
[CrossRef] [Google Scholar] [Publisher Link]
[24] Hiroaki Ishikawa, and Wallace F. Marshall, “Intraflagellar Transport and Ciliary Dynamics,” Cold Spring Harbor Perspectives in Biology, vol. 9, no. 3, 2017.
[CrossRef] [Google Scholar] [Publisher Link]
[25] Roberta de Melo Roiz et al., “Gait Analysis Comparing Parkinson's Disease with Healthy Elderly Subjects,” Arquivos de Neuro-Psiquiatria, vol. 68, no. 1, pp. 81-86, 2010.
[CrossRef] [Google Scholar] [Publisher Link]
[26] Xiangyu Zhang et al., “Shufflenet: An Extremely Efficient Convolutional Neural Network for Mobile Devices,” 2018 IEEE/CVF Conference on Computer Vision and Pattern Recognition (CVPR), pp. 6848-6856, 2018.
[CrossRef] [Google Scholar] [Publisher Link]
[27] Ioannis Pachoulakis, and Konstantinos Kourmoulis, “Building a Gait Analysis Framework for Parkinson's Disease Patients: Motion Capture and Skeleton 3D Representation,” 2014 International Conference on Telecommunications and Multimedia (TEMU), Heraklion, Greece, pp. 220-225, 2014.
[CrossRef] [Google Scholar] [Publisher Link]
[28] Weiling Cui et al., “Effects of Toe-Out and Toe-In Gaits on Lower-Extremity Kinematics, Dynamics, and Electromyography,” Applied Sciences, vol. 9, no. 23, 2019.
[CrossRef] [Google Scholar] [Publisher Link]
[29] Kathryn E. Shaw et al., “The Effects of Shoe-Worn Insoles on Gait Biomechanics in People with Knee Osteoarthritis: A Systematic Review and Meta-Analysis,” British Journal of Sports Medicine, vol. 52, no. 4, pp. 238-253, 2018.
[CrossRef] [Google Scholar] [Publisher Link]
[30] Aleš Procházka et al., “Deep Learning for Accelerometric Data Assessment and Ataxic Gait Monitoring,” IEEE Transactions on Neural Systems and Rehabilitation Engineering, vol. 29, pp. 360-367, 2021.
[CrossRef] [Google Scholar] [Publisher Link]
[31] Lacramioara Dranca et al., “Using Kinect to Classify Parkinson’s Disease Stages Related to the Severity of Gait Impairment,” BMC Bioinformatics, vol. 19, 2018.
[CrossRef] [Google Scholar] [Publisher Link]
[32] Sha Zhu et al., “Gait Analysis with Wearables is a Potential Progression Marker in Parkinson’s Disease,” Brain Sciences, vol. 12, no. 9, 2022.
[CrossRef] [Google Scholar] [Publisher Link]
[33] Margarita Kotti et al., “Detecting Knee Osteoarthritis and Its Discriminating Parameters Using Random Forests,” Medical Engineering & Physics, vol. 43, pp. 19-29, 2017.
[CrossRef] [Google Scholar] [Publisher Link]
[34] Neila Mezghani et al., “Biomechanical Analysis to Characterize the Impact of Knee Osteoarthritis on Hip, Knee, and Ankle Kinematics,” Journal of Biomedical Engineering and Informatics, vol. 3, no. 2, pp. 36-42, 2017.
[CrossRef] [Google Scholar] [Publisher Link]
[35] Md. Akhtaruzzaman, Amir A. Shafie, and Md. Raisuddin Khan, “Representation of Human Gait Trajectory through Temporospatial Image Modelling,” ARPN Journal of Engineering and Applied Sciences, vol. 11, no. 6, pp. 4105-4110, 2016.
[Google Scholar] [Publisher Link]
[36] C.L. Hubley-Kozey et al., “Longitudinal Changes in Knee Joint Gait Mechanics and Muscle Activation Patterns in Individuals with Medial Compartment Knee Osteoarthritis,” Osteoarthritis and Cartilage, vol. 28, pp. S58-S59, 2020.
[CrossRef] [Google Scholar] [Publisher Link]
[37] Yulian Pristanti Sani Putri, and Atika Yulianti, “Effectiveness of Kinesio Taping and Retro Walking Exercise on the Knee Range of Motion in Older People with Osteoarthritis,” Physical Therapy Journal of Indonesia, vol. 1, no. 2, pp. 23-25, 2020.
[CrossRef] [Google Scholar] [Publisher Link]
[38] Lin Shu et al., “In-Shoe Plantar Pressure Measurement and Analysis System Based on Fabric Pressure Sensing Array,” IEEE Transactions on Information Technology in Biomedicine, vol. 14, no. 3, pp. 767-775, 2010.
[CrossRef] [Google Scholar] [Publisher Link]
[39] Wei Zeng et al., “Classification of Asymptomatic and Osteoarthritic Knee Gait Patterns Using Gait Analysis via Deterministic Learning,” Artificial Intelligence Review, vol. 52, pp. 449-467, 2019.
[CrossRef] [Google Scholar] [Publisher Link]
[40] Georg Bergmann et al., “Loading of the Hip and Knee Joints during Whole-Body Vibration Training,” PLoS One, vol. 13, no. 12, 2018.
[CrossRef] [Google Scholar] [Publisher Link]
[41] Y. Okuma, H. Mitoma, and M. Yoneyama, “Detection and Quantification of Freezing of Gait and Falls in Parkinson's Disease Patients Using a Body-Worn Sensor,” Journal of the Neurological Sciences, vol. 357, 2015.
[CrossRef] [Google Scholar] [Publisher Link]
[42] Jasper Huang, Fabio Di Troia, and Mark Stamp, “Acoustic Gait Analysis Using Support Vector Machines,” Proceedings of the 4th International Conference on Information Systems Security and Privacy, vol. 1, pp. 545-552, 2018.
[CrossRef] [Google Scholar] [Publisher Link]
[43] Negin Karimi Hosseini, and Md Jan Nordin, “Human Gait Recognition: A Silhouette-Based Approach,” Journal of Automation and Control Engineering, vol. 1, no. 2, pp. 103-105, 2013.
[CrossRef] [Google Scholar] [Publisher Link]
[44] Yui Shigeki et al., “Directional Characteristics Evaluation of Silhouette-Based Gait Recognition,” IPSJ Transactions on Computer Vision and Applications, vol. 10, 2018.
[CrossRef] [Google Scholar] [Publisher Link]
[45] Md. Zasim Uddin et al., “Spatio-Temporal Silhouette Sequence Reconstruction for Gait Recognition against Occlusion,” IPSJ Transactions on Computer Vision and Applications, vol. 11, 2019.
[CrossRef] [Google Scholar] [Publisher Link]
[46] Sachin Shetty, and Y.S. Rao, “SVM-Based Machine Learning Approach to Identify Parkinson's Disease Using Gait Analysis,” 2016 International Conference on Inventive Computation Technologies (ICICT), Coimbatore, India, pp. 1-5, 2016.
[CrossRef] [Google Scholar] [Publisher Link]
[47] Yingpeng Wang et al., “The Validity and Reliability of a New Intelligent Three-Dimensional Gait Analysis System in Healthy Subjects and Patients with Post-Stroke,” Sensors, vol. 22, no. 23, 2022.
[CrossRef] [Google Scholar] [Publisher Link]
[48] Aybuke Kececi et al., “Implementation of Machine Learning Algorithms for Gait Recognition,” Engineering Science and Technology, an International Journal, vol. 23, no. 4, pp. 931-937, 2020.
[CrossRef] [Google Scholar] [Publisher Link]
[49] Jucheol Moon et al., “Multimodal Few-Shot Learning for Gait Recognition,” Applied Sciences, vol. 10, no. 21, 2020.
[CrossRef] [Google Scholar] [Publisher Link]
[50] Lei Wang et al., “Two Shank-Mounted IMUs-Based Gait Analysis and Classification for Neurological Disease Patients,” IEEE Robotics and Automation Letters, vol. 5, no. 2, pp. 1970-1976, 2020.
[CrossRef] [Google Scholar] [Publisher Link]
[51] Xuerong Shao et al., “Impaired Ankle Inversion Proprioception during Walking is Associated with Fear of Falling in Older Adults,” Frontiers in Aging Neuroscience, vol. 14, 2022.
[CrossRef] [Google Scholar] [Publisher Link]
[52] Mihaela Hnatiuc et al., “Human Signature Identification Using IoT Technology and Gait Recognition,” Electronics, vol. 10, no. 7, 2021.
[CrossRef] [Google Scholar] [Publisher Link]
[53] Nooritawati Md. Tahir, and Hany Hazfiza Manap, “Parkinson's Disease Gait Classification Based on Machine Learning Approach,” Journal of Applied Sciences, vol. 12, no. 2, pp. 180-185, 2012.
[CrossRef] [Google Scholar] [Publisher Link]
[54] Abdul Saboor et al., “Latest Research Trends in Gait Analysis Using Wearable Sensors and Machine Learning: A Systematic Review,” IEEE Access, vol. 8, pp. 167830-167864, 2020.
[CrossRef] [Google Scholar] [Publisher Link]
[55] Xiuhui Wang, and Ke Yan, “Gait Classification through CNN-Based Ensemble Learning,” Multimedia Tools and Applications, vol. 80, no. 1, pp. 1565-1581, 2021.
[CrossRef] [Google Scholar] [Publisher Link]
[56] Xiuhui Wang, and Jiajia Zhang, “Gait Feature Extraction and Gait Classification Using Two-Branch CNN,” Multimedia Tools and Applications, vol. 79, no. 3, pp. 2917-2930, 2020.
[CrossRef] [Google Scholar] [Publisher Link]
[57] Mohamad Wazzeh et al., “CRSFL: Cluster-Based Resource-Aware Split Federated Learning for Continuous Authentication,” Journal of Network and Computer Applications, vol. 231, 2024.
[CrossRef] [Google Scholar] [Publisher Link]
[58] Ali Rohan et al., “Human Pose Estimation-Based Real-Time Gait Analysis Using Convolutional Neural Network,” IEEE Access, vol. 8, pp. 191542-191550, 2020.
[CrossRef] [Google Scholar] [Publisher Link]
[59] Qin Zou et al., “Deep Learning-Based Gait Recognition Using Smartphones in the Wild,” IEEE Transactions on Information Forensics and Security, vol. 15, pp. 3197-3212, 2020.
[CrossRef] [Google Scholar] [Publisher Link]
[60] Wei Shao et al., “A Multi-Modal Gait Analysis-Based Detection System of the Risk of Depression,” IEEE Journal of Biomedical and Health Informatics, vol. 26, no. 10, pp. 4859-4868, 2022.
[CrossRef] [Google Scholar] [Publisher Link]
[61] Joohwan Sung et al., “Prediction of Lower Extremity Multi-Joint Angles during Overground Walking by Using a Single IMU with a Low Frequency Based on an LSTM Recurrent Neural Network,” Sensors, vol. 22, no. 1, 2021.
[CrossRef] [Google Scholar] [Publisher Link]
[62] Tirthendu Prosad Chakravorty et al., “Interpretable Violence Detection Using Separable Convolution and Bidirectional LSTM,” 2023 IEEE Asia-Pacific Conference on Computer Science and Data Engineering (CSDE), Nadi, Fiji, pp. 01-06, 2023.
[CrossRef] [Google Scholar] [Publisher Link]
[63] Alexander Turner, and Stephen Hayes, “The Classification of Minor Gait Alterations Using Wearable Sensors and Deep Learning,” IEEE Transactions on Biomedical Engineering, vol. 66, no. 11, pp. 3136-3145, 2019.
[CrossRef] [Google Scholar] [Publisher Link]
[64] Fangzheng Wang, Lei Yan, and Jiang Xiao, “Recognition of the Gait Phase Based on New Deep Learning Algorithm Using Multisensor Information Fusion,” Sensors & Materials, vol. 31, no. 10(1), pp. 3041-3054, 2019.
[CrossRef] [Google Scholar] [Publisher Link]
[65] Xiaoqian Zhang, Xiyin Rong, and Hanwen Luo, “Optimizing Lower Limb Rehabilitation: The Intersection of Machine Learning and Rehabilitative Robotics,” Frontiers in Rehabilitation Sciences, vol. 5, 2024.
[CrossRef] [Google Scholar] [Publisher Link]
[66] Hyun-Joon Yoo et al., “Deep Learning-Based Prediction Model for Gait Recovery after a Spinal Cord Injury,” Diagnostics, vol. 14, no. 6, 2024.
[CrossRef] [Google Scholar] [Publisher Link]
[67] Yoshifumi Mizuguchi et al., “Machine Learning-Based Gait Analysis to Predict Clinical Frailty Scale in Elderly Patients with Heart Failure,” European Heart Journal-Digital Health, vol. 5, no. 2, pp. 152-162, 2024.
[CrossRef] [Google Scholar] [Publisher Link]
[68] Suhun Jung et al., “Machine-Learning-Based Coordination of Powered Ankle-Foot Orthosis and Functional Electrical Stimulation for Gait Control,” Frontiers in Bioengineering and Biotechnology, vol. 11, 2024.
[CrossRef] [Google Scholar] [Publisher Link]
[69] Albara Ah Ramli et al., “Gait Characterization in Duchenne Muscular Dystrophy (DMD) Using a Single-Sensor Accelerometer: Classical Machine Learning and Deep Learning Approaches,” Sensors, vol. 24, no. 4, 2024.
[CrossRef] [Google Scholar] [Publisher Link]
[70] Alireza Ettefagh, and Atena Roshan Fekr, “Enhancing Automated Lower Limb Rehabilitation Exercise Task Recognition through Multi-Sensor Data Fusion in Telerehabilitation,” Biomedical Engineering Online, vol. 23, 2024.
[CrossRef] [Google Scholar] [Publisher Link]
[71] Abdulwahab Alazeb et al., “Effective Gait Abnormality Detection in Parkinson Patients for Multi-Sensors Surveillance System,” IEEE Access, vol. 12, pp. 48686-48698, 2024.
[CrossRef] [Google Scholar] [Publisher Link]
[72] Zhengyang Lan et al., “Towards a Diagnostic Tool for Neurological Gait Disorders in Childhood Combining 3D Gait Kinematics and Deep Learning,” Computers in Biology and Medicine, vol. 171, 2024.
[CrossRef] [Google Scholar] [Publisher Link]
[73] Yu Jing et al., “Deep Learning-Assisted Gait Parameter Assessment for Neurodegenerative Diseases: Model Development and Validation,” Journal of Medical Internet Research, vol. 25, 2023.
[CrossRef] [Google Scholar] [Publisher Link]
[74] João Monge et al., “AI-Based Smart Sensing and AR for Gait Rehabilitation Assessment,” Information, vol. 14, no. 7, 2023.
[CrossRef] [Google Scholar] [Publisher Link]
[75] Rytis MaskeliĆ«nas et al., “BiomacVR: A Virtual Reality-Based System for Precise Human Posture and Motion Analysis in Rehabilitation Exercises Using Depth Sensors,” Electronics, vol. 12, no. 2, 2023.
[CrossRef] [Google Scholar] [Publisher Link]
[76] Ciro Mennella et al., “A Deep Learning System to Monitor and Assess Rehabilitation Exercises in Home-Based Remote and Unsupervised Conditions,” Computers in Biology and Medicine, vol. 166, 2023.
[CrossRef] [Google Scholar] [Publisher Link]
[77] Svonko Galasso et al., “Predicting Physical Activity Levels from Kinematic Gait Data Using Machine Learning Techniques,” Engineering Applications of Artificial Intelligence, vol. 123, 2023.
[CrossRef] [Google Scholar] [Publisher Link]
[78] Michael Tschuggnall et al., “Machine Learning Approaches to Predict Rehabilitation Success Based on Clinical and Patient-Reported Outcome Measures,” Informatics in Medicine Unlocked, vol. 24, 2021.
[CrossRef] [Google Scholar] [Publisher Link]
[79] Ilya Sutskever, Oriol Vinyals, and Quoc V. Le, ‘‘Sequence to Sequence Learning with Neural Networks,” Proceedings of the 27th International Conference on Neural Information Processing Systems, vol. 2, pp. 3104-3112, 2014.
[Google Scholar] [Publisher Link]
[80] Dana Utebayeva, Lyazzat Ilipbayeva, and Eric T. Matson, “Practical Study of Recurrent Neural Networks for Efficient Real-Time Drone Sound Detection: A Review,” Drones, vol. 7, no. 1, 2023.
[CrossRef] [Google Scholar] [Publisher Link]
[81] Adil Khan et al., ‘‘Treatment Outcome Prediction Using Multi-Task Learning: Application to Botulinum Toxin in Gait Rehabilitation,” Sensors, vol. 22, no. 21, 2022.
[CrossRef] [Google Scholar] [Publisher Link]
[82] Chandrasen Pandey et al., “GaitRec-Net: A Deep Neural Network for Gait Disorder Detection Using Ground Reaction Force,” PPAR Research, 2022.
[CrossRef] [Google Scholar] [Publisher Link]
[83] Darshan Jani et al., “An Efficient Gait Abnormality Detection Method Based on Classification,” Journal of Sensor and Actuator Networks, vol. 11, no. 3, 2022.
[CrossRef] [Google Scholar] [Publisher Link]
[84] Nazia Ejaz et al., “Automatic Gait Classification Model Empowered by Machine Learning for People with and without Osteoporosis Using Smart Walker,” Applied Sciences, vol. 14, no. 9, 2024.
[CrossRef] [Google Scholar] [Publisher Link]
[85] Xiaohong Li et al., “Stroke Rehabilitation: From Diagnosis to Therapy,” Frontiers in Neurology, vol. 15, 2024.
[CrossRef] [Google Scholar] [Publisher Link]
[86] Silong Lyu, and Rafael Bidarra, “Procedural Generation of Challenges for Personalized Gait Rehabilitation,” Proceedings of the 18th International Conference on the Foundations of Digital Games, pp. 1-11, 2023.
[CrossRef] [Google Scholar] [Publisher Link]