Machine Learning and Smart Devices for Prediction of Heart Disease, Diabetes, and Obesity: Systematic Review
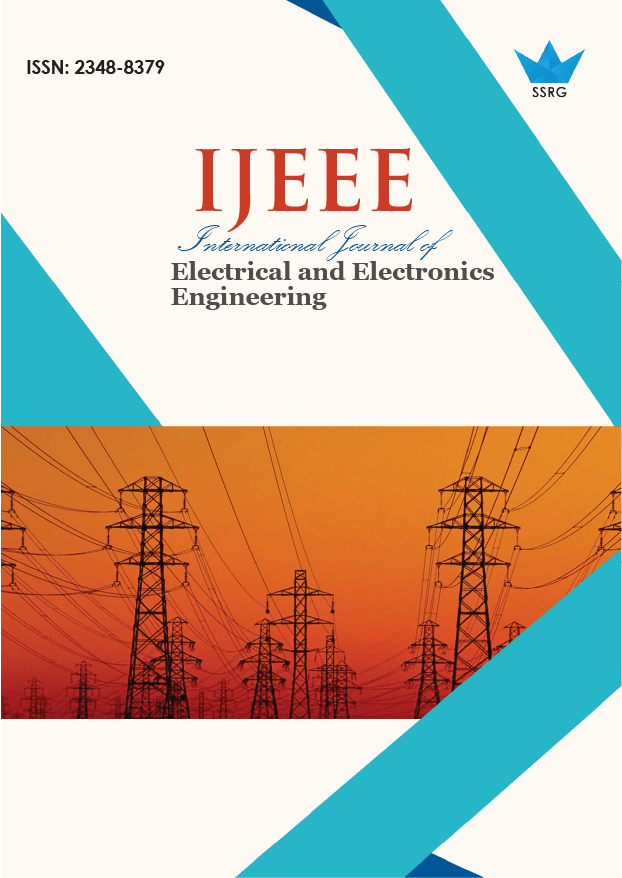
International Journal of Electrical and Electronics Engineering |
© 2024 by SSRG - IJEEE Journal |
Volume 11 Issue 11 |
Year of Publication : 2024 |
Authors : Kirti Gupta, Pardeep Kumar, Shuchita Upadhyaya, Shalini Aggarwal |
How to Cite?
Kirti Gupta, Pardeep Kumar, Shuchita Upadhyaya, Shalini Aggarwal, "Machine Learning and Smart Devices for Prediction of Heart Disease, Diabetes, and Obesity: Systematic Review," SSRG International Journal of Electrical and Electronics Engineering, vol. 11, no. 11, pp. 222-230, 2024. Crossref, https://doi.org/10.14445/23488379/IJEEE-V11I11P122
Abstract:
Heart disease, diabetes, and obesity are major global health issues and are increasing day by day. There is a need for effective predictive strategies. Machine learning is vital for early detection and diagnosis. It provides timely and individualized healthcare interventions. This analysis evaluates optimal ML algorithms for heart disease, diabetes, and obesity. It identifies key risk factors and highlights the role of AI in healthcare. The study follows PRISMA guidelines. A broad search using Google Scholar and PubMed identified 30 studies from 2014 to 2024. The article explores the symptoms and aftereffects of diabetes, obesity, and heart disease. It reviews effective ML methods for forecasting these issues. AI-based methods help medical professionals diagnose diseases. Research shows that AI tools improve diagnosis speed and accuracy. This leads to more personalized treatment and better outcomes.
Keywords:
Diabetes, Healthcare, Heart disease, Machine Learning (ML), Obesity.
References:
[1] Yogesh Kumar et al., “Artificial Intelligence in Disease Diagnosis: A Systematic Literature Review, Synthesizing Framework and Future Research Agenda,” Journal of Ambient Intelligence and Humanized Computing, vol. 14, no. 7, pp. 8459-8486, 2023.
[CrossRef] [Google Scholar] [Publisher Link]
[2] Mahmood Safaei et al., “A Systematic Literature Review on Obesity: Understanding the Causes & Consequences of Obesity and Reviewing Various Machine Learning Approaches Used to Predict Obesity,” Computers in Biology and Medicine, vol. 136, 2021.
[CrossRef] [Google Scholar] [Publisher Link]
[3] Mohammed Amine Makroum et al., “Machine Learning and Smart Devices for Diabetes Management: Systematic Review,” Sensors, vol. 22, no. 5, 2022.
[CrossRef] [Google Scholar] [Publisher Link]
[4] Serena Zanelli et al., “Diabetes Detection and Management through Photoplethysmographic and Electrocardiographic Signals Analysis: A Systematic Review,” Sensors, vol. 22, no. 13, 2022.
[CrossRef] [Google Scholar] [Publisher Link]
[5] Rahul Katarya, and Polipireddy Srinivas, “Predicting Heart Disease at Early Stages Using Machine Learning: A Survey,” 2020 International Conference on Electronics and Sustainable Communication Systems (ICESC), Coimbatore, India, pp. 302-305, 2020.
[CrossRef] [Google Scholar] [Publisher Link]
[6] Farida Mohsen et al., “A Scoping Review of Artificial Intelligence-Based Methods for Diabetes Risk Prediction,” npj Digital Medicine, vol. 6, no. 1, 2023.
[CrossRef] [Google Scholar] [Publisher Link]
[7] Aishwarya Mujumdar, and V. Vaidehi, “Diabetes Prediction Using Machine Learning Algorithms,” Procedia Computer Science, vol. 165, pp. 292-299, 2019.
[CrossRef] [Google Scholar] [Publisher Link]
[8] K.M. Jyoti Rani, “Diabetes Prediction Using Machine Learning,” International Journal of Scientific Research in Computer Science, Engineering and Information Technology, vol. 6, no. 4, pp. 294-305, 2020.
[CrossRef] [Google Scholar] [Publisher Link]
[9] Abid Sarwar et al., “Diagnosis of Diabetes Type-II Using Hybrid Machine Learning Based Ensemble Model,” International Journal of Information Technology, vol. 12, no. 2, pp. 419-428, 2020.
[CrossRef] [Google Scholar] [Publisher Link]
[10] Orlando Iparraguirre-Villanueva et al., “Application of Machine Learning Models for Early Detection and Accurate Classification of Type 2 Diabetes,” Diagnostics, vol. 13, no. 14, 2023.
[CrossRef] [Google Scholar] [Publisher Link]
[11] Harleen Kaur, and Vinita Kumari, “Predictive Modelling and Analytics for Diabetes Using a Machine Learning Approach,” Applied Computing and Informatics, vol. 18, no. 1/2, pp. 90-100, 2022.
[CrossRef] [Google Scholar] [Publisher Link]
[12] Salleh Sonko et al., “Predicting Long-Term Type 2 Diabetes with Artificial Intelligence (AI): A Scoping Review,” Studies in Health Technology and Informatics, vol. 305, pp. 652-655, 2023.
[CrossRef] [Google Scholar] [Publisher Link]
[13] Jayakumar Kaliappan et al., “Analyzing Classification and Feature Selection Strategies for Diabetes Prediction across Diverse Diabetes Datasets,” Frontiers in Artificial Intelligence, vol. 7, 2024.
[CrossRef] [Google Scholar] [Publisher Link]
[14] Henock M. Deberneh, and Intaek Kim, “Prediction of Type 2 Diabetes Based on Machine Learning Algorithm,” International Journal of Environmental Research and Public Health, vol. 18, no. 6, 2021.
[CrossRef] [Google Scholar] [Publisher Link]
[15] K. Gaurav et al., “Human Disease Prediction Using Machine Learning Techniques and Real-life Parameters,” International Journal of Engineering, vol. 36, no. 6, pp. 1092-1098, 2023.
[CrossRef] [Google Scholar] [Publisher Link]
[16] Anita Bhatia et al., “Modeling Obesity in Complex Food Systems: Systematic Review,” Frontiers in Endocrinology, vol. 13, 2022.
[CrossRef] [Google Scholar] [Publisher Link]
[17] Xiaobei Zhou, Lei Chen, and Hui-Xin Liu, “Applications of Machine Learning Models to Predict and Prevent Obesity: A Mini-Review,” Frontiers in Nutrition, vol. 9, 2022.
[CrossRef] [Google Scholar] [Publisher Link]
[18] Aamir Javaid et al., “Medicine 2032: The Future of Cardiovascular Disease Prevention with Machine Learning and Digital Health Technology,” American Journal of Preventive Cardiology, vol. 12, 2022.
[CrossRef] [Google Scholar] [Publisher Link]
[19] Dahlak Daniel Solomon et al., “Hybrid Majority Voting: Prediction and Classification Model for Obesity,” Diagnostics, vol. 13, no. 15, 2023.
[CrossRef] [Google Scholar] [Publisher Link]
[20] Amin Gasmi, “Machine Learning and Bioinformatics for Diagnosis Analysis of Obesity Spectrum Disorders,” arXiv, 2022.
[CrossRef] [Google Scholar] [Publisher Link]
[21] Zhaoji Fu et al., “Artificial-Intelligence-Enhanced Mobile System for Cardiovascular Health Management,” Sensors, vol. 21, no. 3, 2021.
[CrossRef] [Google Scholar] [Publisher Link]
[22] Jessica Torres-Soto, and Euan A. Ashley, “Multi-Task Deep Learning for Cardiac Rhythm Detection in Wearable Devices,” npj Digital Medicine, vol. 3, no. 1, 2020.
[CrossRef] [Google Scholar] [Publisher Link]
[23] Aditya Khamparia, and Karan Mehtab Singh, “A Systematic Review on Deep Learning Architectures and Applications,” Expert Systems, vol. 36, no. 3, 2019.
[CrossRef] [Google Scholar] [Publisher Link]
[24] Francesco Goretti et al., “Deep Learning for Predicting Congestive Heart Failure,” Electronics, vol. 11, no. 23, 2022.
[CrossRef] [Google Scholar] [Publisher Link]
[25] Balbir Singh, and Hissam Tawfik, “A Machine Learning Approach for Predicting Weight Gain Risks in Young Adults,” 2019 10th International Conference on Dependable Systems, Services and Technologies (DESSERT), Leeds, UK, pp. 231-234, 2019.
[CrossRef] [Google Scholar] [Publisher Link]
[26] T.M. Dugan et al., “Machine Learning Techniques for Prediction of Early Childhood Obesity,” Applied Clinical Informatics, vol. 6, no. 3, pp. 506-520, 2015.
[CrossRef] [Google Scholar] [Publisher Link]
[27] P. Fergus et al., “A Machine Learning Approach to Measure and Monitor Physical Activity in Children to Help Fight Overweight and Obesity,” Intelligent Computing Theories and Methodologies, pp. 676-688, 2015.
[CrossRef] [Google Scholar] [Publisher Link]
[28] Todd Lingren et al., “Developing an Algorithm to Detect Early Childhood Obesity in Two Tertiary Pediatric Medical Centers,” Applied Clinical Informatics, vol. 7, no. 3, pp. 693-706, 2016.
[CrossRef] [Google Scholar] [Publisher Link]
[29] Muhammed Kürşad Uçar et al., “Estimation of Body Fat Percentage Using Hybrid Machine Learning Algorithms,” Measurement, vol. 167, 2021.
[CrossRef] [Google Scholar] [Publisher Link]
[30] Tania Fernández-Navarro et al., “Exploring the Interactions between Serum Free Fatty Acids and Fecal Microbiota in Obesity through a Machine Learning Algorithm,” Food Research International, vol. 121, pp. 533-541, 2019.
[CrossRef] [Google Scholar] [Publisher Link]
[31] G. Swapna, R. Vinayakumar, and K.P. Soman, “Diabetes Detection Using Deep Learning Algorithms,” ICT Express, vol. 4, no. 4, pp. 243-246, 2018.
[CrossRef] [Google Scholar] [Publisher Link]