Analyze the Performance of Massive MIMO System Utilizing One Bit ADCs: Deep Learning-Based Approach with Varying SNR and Limited Pilot Resources
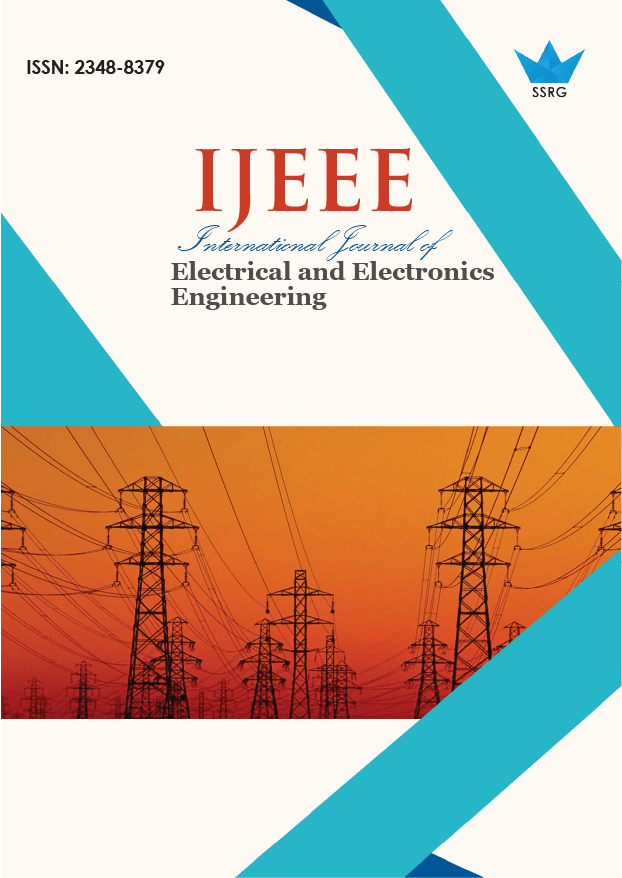
International Journal of Electrical and Electronics Engineering |
© 2024 by SSRG - IJEEE Journal |
Volume 11 Issue 11 |
Year of Publication : 2024 |
Authors : Raksha Thakur, Vineeta Saxena Nigam |
How to Cite?
Raksha Thakur, Vineeta Saxena Nigam, "Analyze the Performance of Massive MIMO System Utilizing One Bit ADCs: Deep Learning-Based Approach with Varying SNR and Limited Pilot Resources," SSRG International Journal of Electrical and Electronics Engineering, vol. 11, no. 11, pp. 284-294, 2024. Crossref, https://doi.org/10.14445/23488379/IJEEE-V11I11P127
Abstract:
One of the essential technologies announced for 5G wireless networks is “Massive Multiple-Input Multiple-Output (MIMO)”. This technology incorporates many antennas at the base station, enabling more information signals to be transmitted and received simultaneously on a common radio channel. Massive-MIMO technology empowers deployments such as signal detection, beamforming, channel estimation, etc. Using conventional methods, the requirement of very long pilot sequences is a prevalent issue in obtaining accurate channel estimation. The main objective of this work is to investigate Deep Learning (DL)-centered hybrid Convolution Neural Network with Multi-Layer Perceptron (CNN-MLP) based channel estimation facilitated with one-bit “Analog-to-Digital Converters” (ADCs) in an uplink Massive MIMO scenario. Initially, preprocessing and data preparation are done to evaluate the model. This involves gathering, normalizing, and dividing considered data into training, validation, and test sets. Then, a CNN-MLP architecture will be designed using a deep learning framework with an input layer, several hidden layers, and an output layer for NMSE prediction. By proper training of the proposed model, efficient channel parameters are calculated with fewer pilot lengths to reduce overhead and increase spectral efficiency. By implementing the proposed model, channel estimation accuracy is enhanced efficiently. Simulation achieves better results according to performance metrics such as NMSE and attainable SNR per antenna. Results showed that “NMSE” performance approach as low as -22.2dB as the number of antennas increases while “SNR per antenna” achieves 99.5% gain for varying received SNRs of 0dB, 10dB, and 20 dB even at smaller pilot length.
Keywords:
Massive MIMO, One-bit ADCs, Channel estimation, Deep Learning (DL), CNN-MLP.
References:
[1] J. Guerreiro, R. Dinis, and P. Montezuma, “Use of 1-Bit Digital-to-Analogue Converters in Massive MIMO Systems,” Electronics Letters, vol. 52, no. 9, pp. 778-779, 2016.
[CrossRef] [Google Scholar] [Publisher Link]
[2] Jiayi Zhang et al., “On Low-Resolution ADCs in Practical 5G Millimeter-Wave Massive MIMO Systems,” IEEE Communications Magazine, vol. 56, no. 7, pp. 205-211, 2018.
[CrossRef] [Google Scholar] [Publisher Link]
[3] Erik G. Larsson et al., “Massive MIMO for Next Generation Wireless Systems,” IEEE Communications Magazine, vol. 52, no. 2, pp. 186-195, 2014.
[CrossRef] [Google Scholar] [Publisher Link]
[4] Jaspreet Singh, Onkar Dabeer, and Upamanyu Madhow, “Capacity of the Discrete-Time AWGN Channel under Output Quantization,” 2008 IEEE International Symposium on Information Theory, Toronto, ON, Canada, pp. 1218-1222, 2008.
[CrossRef] [Google Scholar] [Publisher Link]
[5] Jaspreet Singh, Onkar Dabeer, and Upamanyu Madhow, “Transceiver Design with Low-Precision Analog-to-Digital Conversion: An Information-Theoretic Perspective,” arXiv, 2008.
[CrossRef] [Google Scholar] [Publisher Link]
[6] Jun Liu, Zhongqiang Luo, and Xingzhong Xiong, “Low-Resolution ADCs for Wireless Communication: A Comprehensive Survey,” IEEE Access, vol. 7, pp. 91291-91324, 2019.
[CrossRef] [Google Scholar] [Publisher Link]
[7] Mahdi Fozi, Ahmad R. Sharafat, and Mehdi Bennis, “Fast MIMO Beamforming via Deep Reinforcement Learning for High Mobility mmWave Connectivity,” IEEE Journal on Selected Areas in Communications, vol. 40, no. 1, pp. 127-142, 2022.
[CrossRef] [Google Scholar] [Publisher Link]
[8] Jun Zhang et al., “Large System Achievable Rate Analysis of RIS-Assisted MIMO Wireless Communication with Statistical CSIT,” IEEE Transactions on Wireless Communications, vol. 20, no. 9, pp. 5572-5585, 2021.
[CrossRef] [Google Scholar] [Publisher Link]
[9] Shuaifei Chen et al., “Wireless Powered IoE for 6G: Massive Access Meets Scalable Cell-Free Massive MIMO,” China Communications, vol. 17, no. 12, pp. 92-109, 2020.
[CrossRef] [Google Scholar] [Publisher Link]
[10] L. Guishan, and Z. Wen, “Analysis and Research on the Influence of ADC Error on System Performance,” Measurement and Control Technology, vol. 22, no. 4, pp. 52-55, 2003.
[Google Scholar]
[11] B. Widrow, “A Study of Rough Amplitude Quantization by Means of Nyquist Sampling Theory,” IRE Transactions on Circuit Theory, vol. 3, no. 4, pp. 266-276, 1956.
[CrossRef] [Google Scholar] [Publisher Link]
[12] David Neumann, Thomas Wiese, and Wolfgang Utschick, “Learning the MMSE Channel Estimator,” IEEE Transactions on Signal Processing, vol. 66, no. 11, pp. 2905-2917, 2018.
[CrossRef] [Google Scholar] [Publisher Link]
[13] Eren Balevi, and Jeffrey G. Andrews, “One-Bit OFDM Receivers via Deep Learning,” IEEE Transactions on Communications, vol. 67, no. 6, pp. 4326-4336, 2019.
[CrossRef] [Google Scholar] [Publisher Link]
[14] Aldebaro Klautau et al., “Detection and Channel Equalization with Deep Learning for Low Resolution MIMO Systems,” 2018 52nd Asilomar Conference on Signals, Systems, and Computers, Pacific Grove, CA, USA, pp. 1836-1840, 2018.
[CrossRef] [Google Scholar] [Publisher Link]
[15] Shen Gao et al., “Deep Learning-Based Channel Estimation for Massive MIMO with Mixed-Resolution ADCs,” IEEE Communications Letters, vol. 23, no. 11, pp. 1989-1993, 2019.
[CrossRef] [Google Scholar] [Publisher Link]
[16] Yu Zhang, Muhammad Alrabeiah, and Ahmed Alkhateeb, “Deep Learning for Massive MIMO with 1-Bit ADCs: When More Antennas Need Fewer Pilots,” IEEE Wireless Communications Letters, vol. 9, no. 8, pp. 1273-1277, 2020.
[CrossRef] [Google Scholar] [Publisher Link]
[17] Akash Doshi, and Jeffrey G. Andrews, “One-Bit mmWave MIMO Channel Estimation Using Deep Generative Networks,” IEEE Wireless Communications Letters, vol. 12, no. 9, pp. 1593-1597, 2023.
[CrossRef] [Google Scholar] [Publisher Link]
[18] Yudi Dong, Huaxia Wang, and Yu-Dong Yao, “Channel Estimation for One-Bit Multiuser Massive MIMO Using Conditional GAN,” IEEE Communications Letters, vol. 25, no. 3, pp. 854-858, 2021.
[CrossRef] [Google Scholar] [Publisher Link]
[19] Islam Helmy, Pulok Tarafder, and Wooyeol Choi, “LSTM-GRU Model-Based Channel Prediction for One-Bit Massive MIMO System,” IEEE Transactions on Vehicular Technology, vol. 72, no. 8, pp. 11053-11057, 2023.
[CrossRef] [Google Scholar] [Publisher Link]
[20] Md Habibur Rahman et al. “Deep Learning Based One Bit-ADCs Efficient Channel Estimation Using Fewer Pilots Overhead for Massive MIMO System,” IEEE Access, vol. 12, pp. 64823-64836, 2024.
[CrossRef] [Google Scholar] [Publisher Link]
[21] Ly V. Nguyen, Duy H. N. Nguyen, and A. Lee Swindlehurst, “Deep Learning for Estimation and Pilot Signal Design in Few-Bit Massive MIMO Systems,” IEEE Transactions on Wireless Communications, vol. 22, no. 1, pp. 379-392, 2023.
[CrossRef] [Google Scholar] [Publisher Link]
[22] Muhammad Alrabeiah, and Ahmed Alkhateeb, “Deep Learning for TDD and FDD Massive MIMO: Mapping Channels in Space and Frequency,” 2019 53rd Asilomar Conference on Signals, Systems, and Computers, Pacific Grove, CA, USA, pp. 1465-1470, 2019.
[CrossRef] [Google Scholar] [Publisher Link]
[23] Ahmed Alkhateeb et al., “Deep Learning Coordinated Beamforming for Highly- Mobile Millimeter Wave Systems,” IEEE Access, vol. 6, pp. 37328-37348, 2018.
[CrossRef] [Google Scholar] [Publisher Link]
[24] Ahmed Alkhateeb, “DeepMIMO: A Generic Deep Learning Dataset for Millimeter Wave and Massive MIMO Applications,” arXiv, 2019.
[CrossRef] [Google Scholar] [Publisher Link]
[25] The Remcom Wireless InSite Website: RemCom, Wireless InSite. [Online]. Available: https://www.remcom.com/wireless-insite