An Overview of ECG Signal Processing and Analysis Techniques for Categorization of Cardiac Diseases
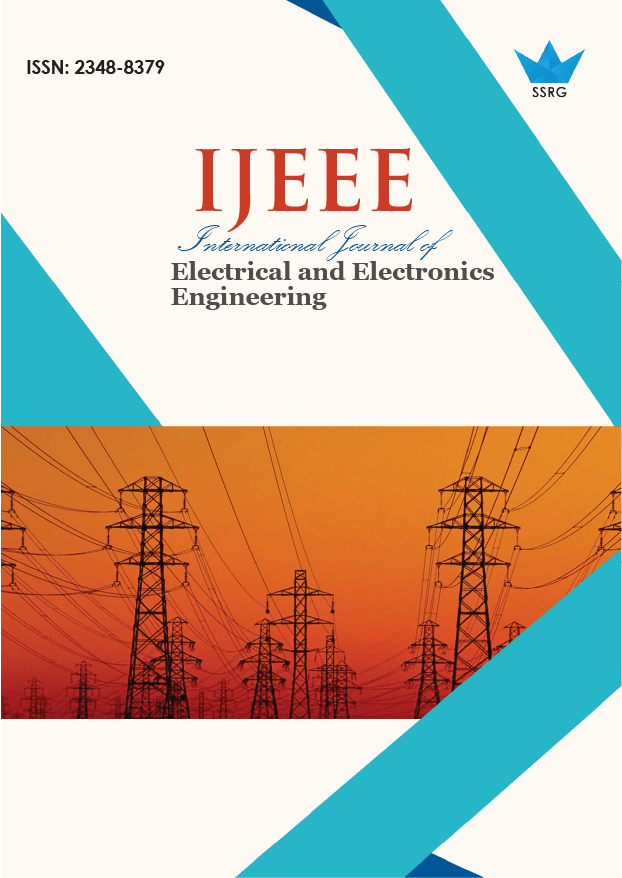
International Journal of Electrical and Electronics Engineering |
© 2024 by SSRG - IJEEE Journal |
Volume 11 Issue 11 |
Year of Publication : 2024 |
Authors : Anurag Kumar Mishra, Asha Ambhaikar, Naveen Kumar Dewangan |
How to Cite?
Anurag Kumar Mishra, Asha Ambhaikar, Naveen Kumar Dewangan, "An Overview of ECG Signal Processing and Analysis Techniques for Categorization of Cardiac Diseases," SSRG International Journal of Electrical and Electronics Engineering, vol. 11, no. 11, pp. 326-340, 2024. Crossref, https://doi.org/10.14445/23488379/IJEEE-V11I11P131
Abstract:
An essential diagnostic technique for assessing cardiac health is an Electrocardiogram (ECG). The heart's electrical activity is captured in this recording. The need to share the workload among physicians and relieve pressure on them has led to the development of automatic detection and classification techniques for heart arrhythmias and other abnormalities as the number of heart patients has increased. All detection and classification techniques operate in the following stages: signal preprocessing, which includes denoising, extracting features, and categorising features. Recently, several methods have been used to denoise, extract features, and categorize ECG signals. The preprocessing of the ECG signal is necessary before the extraction phase because numerous noise sources in a medical setting can deteriorate the signal. The present study reviews ECG signal analysis, feature extraction, and denoising techniques. Frequency domain filters adaptive, and Wavelet Transform (WT) based filters are commonly used to denoise ECG signals. For the ultimate classification task, various morphological, temporal, and statistical features, Fourier transform, and wavelet-based coefficients are frequently extracted from the ECG signals. Findings show that deep learning methods are best among the others for the classification task and that hybrid features increase detection efficacy. Most authors have attempted to categorize ECG into five classes. There is scope to identify the features that combine most effectively to provide better performance in categorising more heart diseases. Also, there is a scope for developing a classifier that performs better to classify a more significant number of heart arrhythmias or diseases.
Keywords:
Analysis, Classification, Deep learning, Feature extraction, Hybrid features.
References:
[1] Global Status Report on Non-Communicable Diseases, 2010. [Online] Available: https://healthyoupromo.wordpress.com/
[2] Philip de Chazal, O‟Dwyer Maria, and B. Reilly Richard, “Automatic Classification of Heartbeats Using ECG Morphology and Heartbeat Interval Features,” IEEE Transactions on Biomedical Engineering, vol. 51, no. 7, pp. 1196-1206, 2004.
[CrossRef] [Google Scholar] [Publisher Link]
[3] Rangaraj M. Rangayyan, Biomedical Signal Analysis: A Case Study approach, Wiley-IEEE Press, 2001.
[Google Scholar] [Publisher Link]
[4] Qi Haibing, Liu Xiongfei, and Pan Chao, “Discrete Wavelet Soft Threshold Denoise Processing for ECG Signal,” 2010 International Conference on Intelligent Computation Technology and Automation, Changsha, China, pp. 126-129, 2010.
[CrossRef] [Google Scholar] [Publisher Link]
[5] Nitish V. Thakor, and Yi-Sheng Zhu, “Application of Adaptive Filtering to ECG Analysis: Noise Cancellation and Arrythmia Detection,” IEEE Transaction on Biomedical Engineering, vol. 38, no. 8, pp. 785-794, 1991.
[CrossRef] [Google Scholar] [Publisher Link]
[6] D.L. Donoho, “De-Noising by Soft Thresholding,” IEEE Transaction on Information Theory, vol. 41, no. 3, pp. 613-627, 1995.
[CrossRef] [Google Scholar] [Publisher Link]
[7] A.K. Ziarani, and A. Konrad, “A Nonlinear Adaptive Method of Elimination of Power Line Interference in ECG Signals,” IEEE Transactions on Biomedical Engineering, vol. 49, no. 6, pp. 540-547, 2002.
[CrossRef] [Google Scholar] [Publisher Link]
[8] Paul S. Addison, “Wavelet Transforms and the ECG: A review,” Physiological Measurement, vol. 26, 2005.
[CrossRef] [Google Scholar] [Publisher Link]
[9] Mohammad Ali Tinati, and Behzad Mozaffary, “A Wavelet Packets Approach to Electrocardiograph Baseline Drift Cancellation,” International Journal of Biomedical Imaging, vol. 2006, 2006.
[CrossRef] [Google Scholar] [Publisher Link]
[10] Mikhled Alfaouri, and Khaled Daqrouq, “ECG Signal Denoising by Wavelet Transform Thresholding,” American Journal of Applied Sciences, vol. 5, no. 3, pp. 276-281, 2008.
[CrossRef] [Google Scholar] [Publisher Link]
[11] Wei Zhang, and Linin Ge, “A Method for Reduction of Noise in the ECG,” 2008 2nd International Conference on Bioinformatics and Biomedical Engineering, Shanghai, China, pp. 2119-2122, 2008.
[CrossRef] [Google Scholar] [Publisher Link]
[12] C. Saritha, V. Sukanya, and Y. Narasimha Murthy, “ECG Signal Analysis Using Wavelet Transforms,” Bulgarian Journal of Physics, vol. 35, no. 1, pp. 68-77, 2008.
[Google Scholar] [Publisher Link]
[13] Yue-Der Lin, and Yu Hen Hu, “Power Line Interference Detection and Suppression in ECG Signal Processing,” IEEE Transaction on Biomedical Engineering, vol. 55, no. 1, pp. 354-357, 2008.
[CrossRef] [Google Scholar] [Publisher Link]
[14] Abdel-Rahman Al-Qawasmi, and Khaled Daqrouq, “ECG Signal Enhancement Using Wavelet Transform,” WEAS Transaction on Biology and Medicine, vol. 7, no. 2, pp. 62-70, 2010.
[Google Scholar] [Publisher Link]
[15] Jiapu Pan, and Willis J. Tompkins, “A Real-Time QRS Detection Algorithm,” IEEE Transactions on Biomedical Engineering, vol. BME-32, no. 3, pp. 230-236, 1985.
[CrossRef] [Google Scholar] [Publisher Link]
[16] I.K. Duskalov, I.A. Dotsinsky, and I.I. Christov, “Developments in ECG Acquisition, Preprocessing, Parameter Measurement, and Recording,” IEEE Engineering in Medicine and Biology, vol. 17, no. 2, pp. 50-58, 1998.
[CrossRef] [Google Scholar] [Publisher Link]
[17] S.Z. Mahmoodabadi, A. Ahmadian, and M.D. Abolhasani, “ECG Feature Extraction Using Daubechies Wavelets,” Proceeding of the Fifth IASTED International Conference, Visualization, Imaging, and Image Processing, Benidorn, Spain, pp. 343-348, 2005.
[Google Scholar]
[18] S. Sumathi, and M.Y. Sanavullah, “Comparative Study of QRS Complex Detection in ECG Based on Discrete Wavelet Transform,” International Journal of Recent Trends in Engineering, vol. 2, no. 5, pp. 273-277, 2009.
[Google Scholar]
[19] Ruchita Gautam, and Anil Kumar Sharma, “Detection of QRS Complexes of ECG Recording Based on Wavelet Transform using MATLAB,” International Journal of Engineering Science and Technology, vol. 2, no. 7, pp. 3038-3044, 2010.
[Google Scholar]
[20] Swati Banerjee, and Madhuchhanda Mitra, “ECG Signal Denoising and QRS Complex Detection by Wavelet Transform Based Thresholding,” Sensors & Transducers Journal, vol. 119, no. 8, pp. 207-214, 2010.
[Google Scholar] [Publisher Link]
[21] K.V.L. Narayana, and A. Bhujanga Rao, “Wavelet Based QRS Detection in ECG Using MATLAB,” Innovative Systems Design and Engineering, vol. 2, no. 7, pp. 60-69, 2011.
[Google Scholar] [Publisher Link]
[22] P. Sasikala, and R.S.D. Wahida Banu, “Extraction of P Wave and T Wave in Electrocardiogram Using Wavelet Transform,” International Journal of Computer Science and Information Technologies, vol. 2, no. 1, pp. 489-493, 2011.
[Google Scholar] [Publisher Link]
[23] D.A. Coast et al., “An Approach to Cardiac Arrhythmia Analysis Using Hidden Markov Models,” IEEE Transactions on Biomedical Engineering, vol. 37, no. 9, pp. 826-836, 1990.
[CrossRef] [Google Scholar] [Publisher Link]
[24] P. Ranjith, P.C. Baby, and P. Joseph, “ECG Analysis Using Wavelet Transform: Application to Myocardial Ischemia Detection,” ITBM-RBM, vol. 24, pp. 44-47, 2003.
[CrossRef] [Google Scholar] [Publisher Link]
[25] G.G. Herrero et al., “Feature Extraction for Heartbeat Classification Using Independent Component Analysis and Matching Pursuits,” Proceedings of 2005 IEEE International Conference on Acoustics, Speech, and Signal Processing, Philadelphia, PA, USA, vol. 4, pp. iv/725-iv/728, 2005.
[CrossRef] [Google Scholar] [Publisher Link]
[26] K.S. Park et al., “Hierarchical Support Vector Machine Based Heartbeat Classification Using Higher Order Statistics and Hermite Basis Function,” 2008 Computers in Cardiology, Bologna, Italy, pp. 229-232, 2008.
[CrossRef] [Google Scholar] [Publisher Link]
[27] Maedeh Kiani Sarkaleh, and Asadollah Shahbahrami, “Classification of ECG Arrhythmias Using Discrete Wavelet Transform and Neural Networks,” International Journal of Computer Science, Engineering and Applications, vol. 2, no. 1, pp. 1-13, 2012.
[CrossRef] [Google Scholar] [Publisher Link]
[28] Indu Saini, and B.S. Saini, “Cardia Arrhythmia Classification Using Error Back Propagation Method,” International Journal of Computer Theory and Engineering, vol. 4, no. 3, pp. 462-464, 2012.
[CrossRef] [Google Scholar] [Publisher Link]
[29] Roshan Joy Martis, U. Rajendra Acharya, and Lim Choo Min, “ECG Beat Classification Using PCA, LDA, ICA and Discrete Wavelet Transform,” Biomedical Signal Processing and Control, vol. 8, no. 5, pp. 437-448, 2013.
[CrossRef] [Google Scholar] [Publisher Link]
[30] Juyoung Park, and Kang Kyungtae, “PcHD: Personalized Classification of Heartbeat Types Using a Decision Tree,” Computers in Biology and Medicine, vol. 54, pp. 79-88, 2014.
[CrossRef] [Google Scholar] [Publisher Link]
[31] Naveen Kumar Dewangan, and S.P. Shukla, “A Survey on ECG Signal Feature Extraction and Analysis Techniques,” International Journal of Innovative Research in Electrical, Electronics, Instrumentation and Control Engineering, vol. 3 no. 6, pp. 12-19, 2015.
[CrossRef] [Google Scholar] [Publisher Link]
[32] Mahmoud M. Bassiouni et al., “Intelligent Hybrid Approaches for Human ECG Signals Identification,” Signal, Image and Video Processing, vol. 12, pp. 941-949, 2018.
[CrossRef] [Google Scholar] [Publisher Link]
[33] Vedavathi Gauribidanur Rangappa, Varaprasad Sahani Venkata Appala, and Alok Agarwal, “Classification of Cardiac Arrhythmia Stages Using Hybrid Features Extraction with K-Nearest Neighbour Classifier of ECG Signals,” International Journal of Intelligent Engineering & Systems, vol. 11, no. 6, pp. 21-32, 2018.
[CrossRef] [Google Scholar] [Publisher Link]
[34] Kourosh Kiani, and Maghsoudi Farzane, “Classification of 7 Arrhythmias from ECG Using Fractal Dimensions,” Journal of Bioinformatics and Systems Biology, vol. 2, no. 3, pp. 053-065, 2019.
[CrossRef] [Google Scholar] [Publisher Link]
[35] Sahil Dalal, and Virendra P. Vishwakarma, “Classification of ECG Signals Using Multi-Cumulants Based Evolutionary Hybrid Classifier,” Scientific Reports, vol. 11, no. 1, 2021.
[CrossRef] [Google Scholar] [Publisher Link]
[36] Halemirle Appaji Deepak, and Thippeswamy Vijayakumar, “ECG Signal Classification with Hybrid Features Using Bayesian Optimized K-Nearest Neighbors Classifier,” International Journal of Intelligent Engineering & Systems, vol. 14, no. 6, pp. 50-65, 2021.
[CrossRef] [Google Scholar] [Publisher Link]
[37] Eftal Sehirli, and Muhammed Kamil Turan, “A Novel Method for the Segmentation of QRS Complex on ECG Signals and Classify Cardiovascular Diseases via a Hybrid Model Based on Machine Learning,” International Journal of Intelligent Systems and Applications in Engineering, vol. 9, no. 1, pp. 1-10, 2021.
[CrossRef] [Google Scholar] [Publisher Link]
[38] Mengze Wu et al., “A Study on Arrhythmia via ECG Signal Classification Using the Convolutional Neural Network,” Frontiers in Computational Neuroscience, vol. 14, 2021.
[CrossRef] [Google Scholar] [Publisher Link]
[39] Santanu Sahoo et al., “Deep Learning-Based System to Predict Cardiac Arrhythmia Using Hybrid Features of Transform Techniques,” Intelligent Systems with Applications, vol. 16, 2022.
[CrossRef] [Google Scholar] [Publisher Link]
[40] Surbhi Bhatia et al., “Classification of Electrocardiogram Signals Based on Hybrid Deep Learning Models,” Sustainability, vol. 14, no. 24, 2022.
[CrossRef] [Google Scholar] [Publisher Link]
[41] Hari Mohan Rai, Anurag Trivedi, and Shailja Shukla, “ECG Signal Processing for Abnormalities Detection Using Multi-Resolution Wavelet Transform and Artificial Neural Network Classifier,” Measurement, vol. 46, no. 9, pp. 3238-3246, 2013.[CrossRef] [Google Scholar] [Publisher Link]