Optimized DL Approach for Microcalcification Analysis in Digital Breast Tomosynthesis
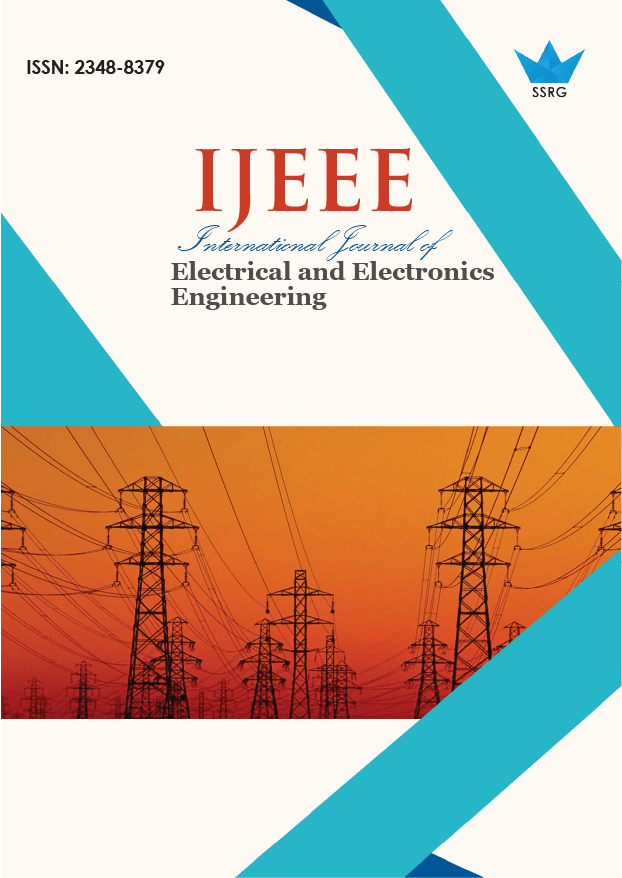
International Journal of Electrical and Electronics Engineering |
© 2024 by SSRG - IJEEE Journal |
Volume 11 Issue 11 |
Year of Publication : 2024 |
Authors : K. Jasna, S. Albert Jerome |
How to Cite?
K. Jasna, S. Albert Jerome, "Optimized DL Approach for Microcalcification Analysis in Digital Breast Tomosynthesis," SSRG International Journal of Electrical and Electronics Engineering, vol. 11, no. 11, pp. 409-423, 2024. Crossref, https://doi.org/10.14445/23488379/IJEEE-V11I11P138
Abstract:
Breast cancer is the primary cause of cancer-related deaths in women globally, and increased survival rates and effective treatment depend on early identification. One of the earliest indications of breast cancer is frequently Microcalcifications (MC), making their accurate classification vital in breast cancer diagnosis. In order to classify MCs using data from Digital Breast Tomosynthesis (DBT), this study suggests an effective Deep Learning (DL) algorithm. Stacked Long Short-Term Memory (LSTM) with Bayesian Optimization was developed and evaluated on the DBT slice. Various features associated with MCs are extracted from the DBT slices with the help of image processing techniques. Extensive Exploratory Data Analysis (EDA) was performed on the DBT dataset to extract key features and identify patterns related to MC classification. Preprocessing techniques were applied to enhance image quality and remove noise. The proposed LSTM model achieved an accuracy of 97.27% and demonstrated superior classification performance, achieving better accuracy than traditional methods. The approach improves breast cancer detection speed and accuracy by automating the categorization process, which presents a possible path for early diagnosis and better patient outcomes. These results advance DL methods in medicine, especially in terms of better radiographic imaging analysis for breast cancer screening.
Keywords:
Microcalcification, Stacked long short-term model, Digital breast tomosynthesis, Explanatory data analysis, Ductal carcinoma.
References:
[1] Asita Elengoe et al., “A Short Review on Breast Cancer,” International Journal of Biotechnology and Biomedicine, vol. 1, no. 1, pp. 1-11, 2024.
[CrossRef] [Google Scholar] [Publisher Link]
[2] Kyril L. Cole et al., “Assessing Survival in Non-Small Cell Lung Cancer Brain Metastases after Stereotactic Radiosurgery: Before and after the Start of the Targetable Mutation Era,” Journal of Neuro-Oncology, vol. 169, no. 3, pp. 671-681, 2024.
[CrossRef] [Google Scholar] [Publisher Link]
[3] J. Haskell, “Raman Spectroscopy Techniques for the Detection and Management of Breast Cancer,” Doctoral Dissertation, University of Exeter, United Kingdom, 2024.
[Google Scholar]
[4] M.T. Ramli Hamid et al., “Abbreviated Breast Magnetic Resonance Imaging (MRI) or Digital Breast Tomosynthesis for Breast Cancer Detection in Dense Breasts? A Retrospective Preliminary Study with Comparable Results,” Clinical Radiology, vol. 79, no. 4, pp. e524-e531, 2024.
[CrossRef] [Google Scholar] [Publisher Link]
[5] Ashish Shiwlani et al., “BI-RADS Category Prediction from Mammography Images and Mammography Radiology Reports Using DL: A Systematic Review,” Jurnal Ilmiah Computer Science, vol. 3, no. 1, pp. 30-49, 2024.
[CrossRef] [Google Scholar] [Publisher Link]
[6] Francesco Prinzi et al., “Interpretable Radiomic Signature for Breast Microcalcification Detection and Classification,” Journal of Imaging Informatics in Medicine, vol. 37, pp. 1038-1053, 2024.
[CrossRef] [Google Scholar] [Publisher Link]
[7] Marwa Ben Ammar et al., “Harnessing Deep Learning for Early Breast Cancer Diagnosis: A Review of Datasets, Methods, Challenges, and Future Directions,” International Journal of Computing and Digital Systems, vol. 16, no. 1, pp. 1643-1661, 2024.
[Google Scholar]
[8] Laxman Singh, and Altaf Alam, “An Efficient Hybrid Methodology for an Early Detection of Breast Cancer in Digital Mammograms,” Journal of Ambient Intelligence and Humanized Computing, vol. 15, no. 1, pp. 337-360, 2024.
[CrossRef] [Google Scholar] [Publisher Link]
[9] Paul Terrassin et al., “Annotation-Free Deep-Learning Framework for Microcalcifications Detection on Mammograms,” Medical Imaging 2024: Computer-Aided Diagnosis, vol. 12927, 2024.
[CrossRef] [Google Scholar] [Publisher Link]
[10] Jinhua Wang et al., “Discrimination of Breast Cancer with Microcalcifications on Mammography by Deep Learning,” Scientific Reports, vol. 6, no. 1, 2016.
[CrossRef] [Google Scholar] [Publisher Link]
[11] Redona Brahimetaj et al., “Improved Automated Early Detection of Breast Cancer Based on High Resolution 3D Micro-CT Microcalcification Images,” BMC Cancer, vol. 22, no. 1, 2022.
[CrossRef] [Google Scholar] [Publisher Link]
[12] Koushlendra Kumar Singh et al., “Deep Learning Capabilities for the Categorization of Microcalcification,” International Journal of Environmental Research and Public Health, vol. 19, no. 4, 2022.
[CrossRef] [Google Scholar] [Publisher Link]
[13] Chrysostomos Marasinou et al., “Improving the Quantitative Analysis of Breast Microcalcifications: A Multiscale Approach,” Journal of Digital Imaging, vol. 36, no. 3, pp. 1016-1028, 2023.
[CrossRef] [Google Scholar] [Publisher Link]
[14] Ehtsham Rasool et al., “Breast Microcalcification Detection in Digital Mammograms Using Deep Transfer Learning Approaches,” Proceedings of the 2023 9th International Conference on Computing and Data Engineering, pp. 58-65, 2023.
[CrossRef] [Google Scholar] [Publisher Link]
[15] Qing Lin et al., “Artificial Intelligence-Based Diagnosis of Breast Cancer by Mammography Microcalcification,” Fundamental Research, 2023.
[CrossRef] [Google Scholar] [Publisher Link]
[16] Zahra Maghsoodzadeh Sarvestani et al., “A Novel Machine Learning Approach on Texture Analysis for Automatic Breast Microcalcification Diagnosis Classification of Mammogram Images,” Journal of Cancer Research and Clinical Oncology, vol. 149, no. 9, pp. 6151-6170, 2023.
[CrossRef] [Google Scholar] [Publisher Link]
[17] Ana M. Mota et al., “Automatic Classification of Simulated Breast Tomosynthesis Whole Images for the Presence of Microcalcification Clusters Using Deep CNNs,” Journal of Imaging, vol. 8, no. 9, 2022.
[CrossRef] [Google Scholar] [Publisher Link]
[18] Nicholas T. Smith, Jonathan W. Merritt, and Emrys R. Phillips, “High-Resolution 3D Geological Modelling of Heterogeneity in Poorly Exposed Glacial Deposits Using Sedimentary and Glaciotectonic Architectural Element Analysis: A Case Example from Sellafield in West Cumbria, UK,” Quarterly Journal of Engineering Geology and Hydrogeology, vol. 56, no. 1, 2022.
[CrossRef] [Google Scholar] [Publisher Link]
[19] Barsha Abhisheka et al., “Recent Trend in Medical Imaging Modalities and their Applications in Disease Diagnosis: A Review,” Multimedia Tools and Applications, vol. 83, no. 14, pp. 43035-43070, 2024.
[CrossRef] [Google Scholar] [Publisher Link]
[20] Deepak S. Uplaonkar, Virupakshappa, and Nagabhushan Patil, “Modified Otsu Thresholding-Based Level Set and Local Directional Ternary Pattern Technique for Liver Tumor Segmentation,” International Journal of System Assurance Engineering and Management, vol. 15, no. 1, pp. 73-83, 2024.
[CrossRef] [Google Scholar] [Publisher Link]
[21] Taoufik Saidani et al., “Design and Implementation of a Real-Time Image Processing System Based on Sobel Edge Detection Using Model-Based Design Methods,” International Journal of Advanced Computer Science & Applications, vol. 15, no. 3, pp. 273-278, 2024.
[CrossRef] [Google Scholar] [Publisher Link]
[22] Mohan Raparthi et al., “Exploratory Data Analysis Techniques-A Comprehensive Review: Reviewing various Exploratory Data Analysis Techniques and their Applications in Uncovering Insights from Raw Data,” Australian Journal of Machine Learning Research & Applications, vol. 4, no. 1, pp. 215-225, 2024.
[Google Scholar] [Publisher Link]
[23] Enrique R. Sebastian, Julio Esparza, and Liset M. de la Prida, “Quantifying the Distribution of Feature Values over Data Represented in Arbitrary Dimensional Spaces,” PLOS Computational Biology, vol. 20, no. 1, 2024.
[CrossRef] [Google Scholar] [Publisher Link]
[24] Oladosu Oyebisi Oladimeji, Ian McLoughlin, and Saritha Unnikrishnan, “Volumetric Attention Mechanism-Based Deep Learning for Breast Cancer Diagnosis in Digital Breast Tomosynthesis,” Proceedings of Ninth International Congress on Information and Communication Technology, pp. 231-241, 2024.
[CrossRef] [Google Scholar] [Publisher Link]
[25] Adam Mračko, Ivan Cimrák, and Lucia Vanovcanová, “Enhancing Breast Microcalcification Classification: From Binary to Three-Class Classifier,” 2024 35th Conference of Open Innovations Association (FRUCT), Tampere, Finland, pp. 473-481, 2024.
[CrossRef] [Google Scholar] [Publisher Link]
[26] Jing Ru Teoh et al., “Enhancing Early Breast Cancer Diagnosis through Automated Microcalcification Classification Using an Optimized Ensemble Deep Learning Framework,” PeerJ Computer Science, vol. 10, 2024.
[CrossRef] [Google Scholar] [Publisher Link]
[27] Filippo Pesapane et al., “Deep Learning Performance for Classification and Classification of Microcalcifications on Mammography,” European Radiology Experimental, vol. 7, no. 1, 2023.
[CrossRef] [Google Scholar] [Publisher Link]
[28] M.C. Shanker, and M. Vadivel, “Micro-Calcification Classification Analysis in Mammogram Images with Aid of Hybrid Technique Analysis,” Wireless Personal Communications, vol. 128, no. 2, pp. 1287-1307, 2022.
[CrossRef] [Google Scholar] [Publisher Link]
[29] Zi Wang et al., “Pre-Trained Gaussian Processes for Bayesian Optimization,” Journal of Machine Learning Research, vol. 25, pp. 1-83, 2024.
[Google Scholar] [Publisher Link]
[30] Arpita Maharatha et al., “Employing Sequence-to-Sequence Stacked LSTM Autoencoder Architecture to Forecast Indian Weather,” Procedia Computer Science, vol. 235, pp. 2258-2268, 2024.
[CrossRef] [Google Scholar] [Publisher Link]