A Novel Design and Implementation of Fertilizer Recommendation System Based on Hybrid Machine Learning Models
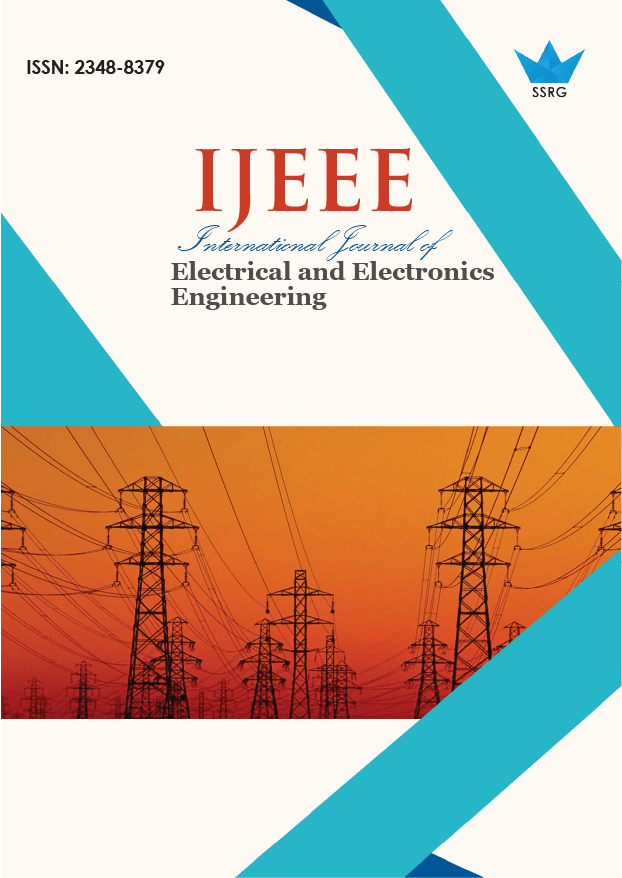
International Journal of Electrical and Electronics Engineering |
© 2024 by SSRG - IJEEE Journal |
Volume 11 Issue 11 |
Year of Publication : 2024 |
Authors : G. Mamatha, Jyothi S. Nayak |
How to Cite?
G. Mamatha, Jyothi S. Nayak, "A Novel Design and Implementation of Fertilizer Recommendation System Based on Hybrid Machine Learning Models," SSRG International Journal of Electrical and Electronics Engineering, vol. 11, no. 11, pp. 448-460, 2024. Crossref, https://doi.org/10.14445/23488379/IJEEE-V11I11P141
Abstract:
India is mostly a farming country. Many of the GDP of emerging nations like India comes from agriculture, so the sector is important to these countries’ economies. The demand for food has skyrocketed due to the increase in population. Crop quality, yield, and profitability can all take a hit when farmers choose their crops, fertilizers, and pesticides without considering factors like soil type, water requirement, temperature conditions, and crop profitability analysis for a specific area. The development of computational technology has prompted scientists to consider various issues, including identifying diseases and pesticides and selecting fertilizers and crops based on soil quality, water needs, and market viability. An essential and fundamental aspect of farming is selecting the appropriate fertilizer for soil and crop production. India has a reputation as an agricultural powerhouse, with traditional practices still used to advise farmers on the best fertilizer to use. Communication between farmers and specialists is currently the basis for suggestions, and various experts have different recommendations. The prohibitive cost of lab technology for determining soil supplement levels is a major concern. Present frameworks for determining soil nutrient content and fertilizer recommendations are ineffective and inefficient. In order to estimate the nutritional dimension in soil and provide suitable fertilizer, this article presents an attractive, novel fertilizer recommendation system, ‘FertRec’. In this proposed system, soil samples are analyzed to identify the deficiency of nutrients, thus preparing the datasets used for training the machine learning models. The best accuracy model recommends a suitable fertilizer based on the soil nutrients. The main goal is to create an effective fertilizer recommendation system to help farmers optimize their fertilizer use. Compared to current benchmark recommendation methods, the proposed system performs four times better on 500 soil samples from the Telangana region in India, using soil features, and it effectively recommends fertilizer with 99.98% accuracy. This will maximize production, and farmers greatly benefit from this method, which involves selecting the appropriate fertilizer at the beginning of the product cycle.
Keywords:
Agriculture, Soil nutrients, Fertilizer recommendation, Machine learning models.
References:
[1] Alejandro Castañeda-Miranda, and Victor M. Castaño-Meneses, “Internet of Things for Smart Farming and Frost Intelligent Control in Greenhouses,” Computers and Electronics in Agriculture, vol. 176, 2020.
[CrossRef] [Google Scholar] [Publisher Link]
[2] Yakindra Prasad Timilsena et al., “Enhanced Efficiency Fertilisers: A Review of Formulation and Nutrient Release Patterns,” Journal of the Science of Food and Agriculture, vol. 95, no. 6, pp. 1131-1142, 2015.
[CrossRef] [Google Scholar] [Publisher Link]
[3] Poreddy Ishika Reddy et al., “Automated Plant Disease Detection Using Convolutional Neural Networks: Enhancing Accuracy and Scalability for Sustainable Agriculture,” International Journal of Computer Engineering in Research Trends, vol. 11, no. 9, pp. 1-10, 2024.
[Publisher Link]
[4] Xiaohui Chen et al., “What has Caused the Use of Fertilizers to Skyrocket in China?,” Nutrient Cycling in Agroecosystems, vol. 110, pp. 241-255, 2018.
[CrossRef] [Google Scholar] [Publisher Link]
[5] Huang Shao-Wen et al., “Reducing Potential of Chemical Fertilizers and Scientific Fertilization Countermeasure in Vegetable Production in China,” Journal of Plant Nutrition and Fertilizers, vol. 23, no. 6, pp. 1480-1493, 2017.
[CrossRef] [Google Scholar] [Publisher Link]
[6] Yuanzhi Guo, and Jieyong Wang, “Spatiotemporal Changes of Chemical Fertilizer Application and Its Environmental Risks in China from 2000 to 2019,” International Journal of Environmental Research and Public Health, vol. 18, no. 22, 2021.
[CrossRef] [Google Scholar] [Publisher Link]
[7] Walter Ocimati, Sivalingam Elayabalan, and Nancy Safari, “Leveraging Deep Learning for Early and Accurate Pre-diction of Banana Crop Diseases: A Classification and Risk Assessment Framework,” International Journal of Computer Engineering in Research Trends, vol. 11, no. 4, pp. 46-57, 2024.
[Publisher Link]
[8] Xiang-de Yang et al., “Effects of Long-Term Nitrogen Application on Soil Acidification and Solution Chemistry of a Tea Plantation in China,” Agriculture, Ecosystems & Environment, vol. 252, pp. 74-82, 2018.
[CrossRef] [Google Scholar] [Publisher Link]
[9] TANG Han et al., “Research Progress Analysis on Key Technology of Chemical Fertilizer Reduction and Efficiency Increase,” Transactions of the Chinese Society of Agricultural Machinery, vol. 50, no. 4, 2019.
[Google Scholar] [Publisher Link]
[10] Xiaoying Yang, and Shubo Fang, “Practices, Perceptions, and Implications of Fertilizer Use in East-Central China,” Ambio, vol. 44, pp. 647-652, 2015.
[CrossRef] [Google Scholar] [Publisher Link]
[11] Wenchao Li et al., “Comprehensive Environmental Impacts of Fertilizer Application vary among Different Crops: Implications for the Adjustment of Agricultural Structure Aimed to Reduce Fertilizer Use,” Agricultural Water Management, vol. 210, pp. 1-10, 2018.
[CrossRef] [Google Scholar] [Publisher Link]
[12] Krupa Patel, and Hiren B. Patel, “Multi-Criteria Agriculture Recommendation System Using Machine Learning for Crop and Fertilizesrs Prediction,” Current Agriculture Research Journal, vol. 11, no. 1, pp. 137-149, 2023.
[CrossRef] [Google Scholar] [Publisher Link]
[13] Chaitanya B.N. et al., “Features Identification for Growth of Certain Crops in Indian Agriculture,” International Journal of Creative Research Thoughts, vol. 9, no. 8, pp. 735-739, 2021.
[Publisher Link]
[14] Online Fertilizer Recommendations. [Online]. Available: http://stcr.gov.in/Farmer/main.aspx
[15] Kiran Shinde, Jerrin Andrei, and Amey Oke, “Web Based Recommendation System for Farmers,” International Journal on Recent and Innovation Trends in Computing and Communication, vol. 3, no. 3, pp. 1444-1448, 2015.
[Publisher Link]
[16] R. G. V. Bramley, and J. Ouzman, “Farmer Attitudes to the Use of Sensors and Automation in Fertilizer Decision-Making: Nitrogen Fertilization in the Australian Grains Sector,” Precision Agriculture, vol. 20, pp. 157-175, 2019.
[CrossRef] [Google Scholar] [Publisher Link]
[17] Rashmi Priya, Dharavath Ramesh, and Ekaansh Khosla, “Crop Prediction on the Region Belts of India: A Naïve Bayes MapReduce Precision Agricultural Model,” 2018 International Conference on Advances in Computing, Communications and Informatics (ICACCI), Bangalore, India, pp. 99-104, 2018.
[CrossRef] [Google Scholar] [Publisher Link]
[18] G. Suresh et al., “Efficient Crop Yield Recommendation System Using Machine Learning for Digital Farming,” International Journal of Modern Agriculture, vol. 10, no. 1, pp. 906-914, 2021.
[Google Scholar] [Publisher Link]
[19] Amit Kumar Sharma, Sandeep Chaurasia, and Devesh Kumar Srivastava, “Supervised Rainfall Learning Model Using Machine Learning Algorithms,” The International Conference on Advanced Machine Learning Technologies and Applications, pp. 275-283, 2018.
[CrossRef] [Google Scholar] [Publisher Link]
[20] Huma Khan, and S.M. Ghosh, “Crop Yield Prediction from Meteorological Data Using Efficient Machine Learning Model,” Proceedings of International Conference on Wireless Communication, pp. 565-574, 2020.
[CrossRef] [Google Scholar] [Publisher Link]
[21] R.S. Loomis, J. Rockström, and M. Bhavsingh, “Synergistic Approaches in Aquatic and Agricultural Modeling for Sustainable Farming,” Synthesis: A Multidisciplinary Research Journal, vol. 1, no. 1, pp. 32-41, 2023.
[Google Scholar] [Publisher Link]
[22] Elma Hot, and Vesna Popović-Bugarin, “Soil Data Clustering by Using K-Means and Fuzzy K-Means Algorithm,” 2015 23rd Telecommunications Forum Telfor (TELFOR), Belgrade, Serbia, pp. 890-893, 2015.
[CrossRef] [Google Scholar] [Publisher Link]
[23] H. Navarro-Hellín et al., “A Decision Support System for Managing Irrigation in Agriculture,” Computers and Electronics in Agriculture, vol. 124, pp. 121-131, 2016.
[CrossRef] [Google Scholar] [Publisher Link]
[24] Kasula Kedhari Priya et al., “Towards a Greener Tomorrow: The Role of Data Science in Shaping Sustainable Farming Practices,” International Journal of Computer Engineering in Research Trends, vol. 11, no. 4, pp. 12-19, 2024.
[Publisher Link]
[25] R.S. Dinesh, and B. Ramamoorthy, “Soil Test Crop Response Based Online Fertiliser Recommendations,” IISS, Bhopal, 1968.
[Publisher Link]
[26] Anu Bala, “Machine Learning Approaches for Crop Yield Prediction-Review,” International Journal of Computer Engineering and Technology, vol. 11, no. 1, pp. 23-27, 2020.
[Google Scholar] [Publisher Link]
[27] U.M. Prakash et al., “KNN-Based Crop and Fertilizer Prediction,” International Journal of Engineering and Advanced Technology, vol. 9, no. 4, pp. 1453-1456, 2020.
[CrossRef] [Publisher Link]
[28] M. Bhavsingh, Y. Alotaibi, and S. Alghamdi, “Fusion of Convolutional Neural Networks and Gradient Boosting Machines for Spinach Leaf Classification and Prediction,” International Journal of Computer Engineering in Research Trends, vol. 11, no. 3, pp. 38-45, 2024.
[Publisher Link]
[29] Tanmay Thorat, B.K. Patle, and Sunil Kumar Kashyap, “Intelligent Insecticide and Fertilizer Recommendation System Based on TPF-CNN for Smart Farming,” Smart Agricultural Technology, vol. 3, 2023.
[CrossRef] [Google Scholar] [Publisher Link]