An Intelligent Antenna Synthesis Method Based on Random Forest Machine Learning Model
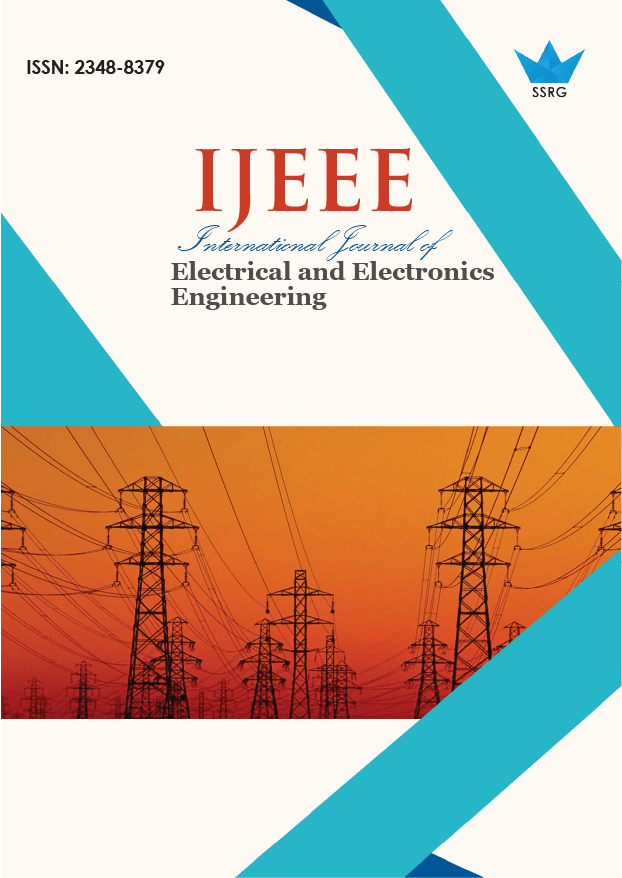
International Journal of Electrical and Electronics Engineering |
© 2024 by SSRG - IJEEE Journal |
Volume 11 Issue 12 |
Year of Publication : 2024 |
Authors : Archana Tiwari, Khushi Prajapati, Arti Patel, Bharti Masram, Nita Nimbarte |
How to Cite?
Archana Tiwari, Khushi Prajapati, Arti Patel, Bharti Masram, Nita Nimbarte, "An Intelligent Antenna Synthesis Method Based on Random Forest Machine Learning Model," SSRG International Journal of Electrical and Electronics Engineering, vol. 11, no. 12, pp. 1-10, 2024. Crossref, https://doi.org/10.14445/23488379/IJEEE-V11I12P101
Abstract:
Using antennas in telecommunications and signal processing has undergone a paradigm shift with implementing Machine Learning (ML) methods and techniques. Implementing ML in antenna design addresses the challenges of performance enhancement, design parameter optimization, and adaptive functionalities. This paper evaluates the transformative impact of ML on antenna systems, emphasizing the use of the random forest regression algorithm in the proposed design. A major challenge in this process is the requirement for extensive training datasets, necessitating the simulation of designs using tools like High-Frequency Structure Simulator (HFSS). These simulations must consider various parameters, such as the dimensions of the substrate and patch. Despite these challenges, integrating ML techniques has optimized the design process, resulting in superior antenna performance. Experimental validations are presented to demonstrate the efficacy of ML-driven antenna design methodologies across different frequency bands and application scenarios. The paper highlights the need for interpretable ML models and scalable optimization for complex antenna systems. Ultimately, the research includes the optimization of antenna Design Parameters.
Keywords:
Flag-shaped antenna, Medical application, Microstrip patch, Roggers RT/Duroid 5880, Machine Learning (ML).
References:
[1] Senthil Kumar Jagatheesaperumal et al., “Explainable AI over the Internet of Things (IoT): Overview, State-of-the-Art and Future Directions,” IEEE Open Journal of the Communications Society, vol. 3, pp. 2106-2136, 2022.
[CrossRef] [Google Scholar] [Publisher Link]
[2] Abdulkadir Celik et al., “A Top-Down Survey on Optical Wireless Communications for the Internet of Things,” IEEE Communications Surveys & Tutorials, vol. 25, no. 1, pp. 1-45, 2023.
[CrossRef] [Google Scholar] [Publisher Link]
[3] Ijaz Ahmad et al., “The Challenges of Artificial Intelligence in Wireless Networks for the Internet of Things: Exploring Opportunities for Growth,” IEEE Industrial Electronics Magazine, vol. 15, no. 1, pp. 16-29, 2021.
[CrossRef] [Google Scholar] [Publisher Link]
[4] Kebonyethebe Ramahatla et al., “Multiband Reconfigurable Antennas for 5G Wireless and CubeSat Applications: A Review,” IEEE Access, vol. 10, pp. 40910-40931, 2022.
[CrossRef] [Google Scholar] [Publisher Link]
[5] Fahad Shamshad, and Muhammad Amin, “Simulation Comparison between HFSS and CST for Design of Conical Horn Antenna,” Journal of Expert Systems, vol. 1, no. 4, pp. 84-90, 2012.
[Google Scholar]
[6] Nayan Sarker et al., “Applications of Machine Learning and Deep Learning in Antenna Design, Optimization, and Selection: A Review,” IEEE Access, vol. 11, pp. 103890-103915, 2023.
[CrossRef] [Google Scholar] [Publisher Link]
[7] Ahmed M. Montaser, “Machine Learning Based Design of Pattern Reconfigurable Antenna,” IEEE Access, vol. 11, pp. 33121-33133, 2023.
[CrossRef] [Google Scholar] [Publisher Link]
[8] Rovin Tiwari, Raghavendra Sharma, and Rahul Dubey, “Microstrip Patch Antenna Parameter Optimization Prediction Model Using Machine Learning Techniques,” International Journal on Recent and Innovation Trends in Computing and Communication, vol. 10, no. 9, pp. 53-59, 2022.
[CrossRef] [Google Scholar] [Publisher Link]
[9] M.C. Bailey, and M.D. Deshpande, “Analysis of Rectangular Microstrip Antennas,” NASA Technical Paper 2276, 1984.
[Google Scholar] [Publisher Link]
[10] Gérard Biau, “Analysis of a Random Forests Model,” Journal of Machine Learning Research, vol. 13, no. 38, pp. 1063-1095, 2012.
[Google Scholar] [Publisher Link]
[11] Yi Tong et al., “Machine Learning-Based Theoretical Optimization of Antenna Design,” Highlights in Science, Engineering and Technology, vol. 27, pp. 681-690, 2022.
[CrossRef] [Google Scholar] [Publisher Link]
[12] Hilal M. El Misilmani, Tarek Naous, and Salwa K. Al Khatib, “A Review on the Design and Optimization of Antennas Using Machine Learning Algorithms and Techniques,” International Journal of RF and Microwave Computer-Aided Engineering, vol. 30, no. 10, 2020.
[CrossRef] [Google Scholar] [Publisher Link]
[13] Francesco Andriulli et al., “Guest Editorial Machine Learning in Antenna Design, Modeling, and Measurements,” IEEE Transactions on Antennas and Propagation, vol. 70, no. 7, pp. 4948-4952, 2022.
[CrossRef] [Google Scholar] [Publisher Link]
[14] Houda Werfelli et al., “Design of Rectangular Microstrip Patch Antenna,” 2016 2nd International Conference on Advanced Technologies for Signal and Image Processing (ATSIP), Monastir, Tunisia, pp. 798-803, 2016.
[CrossRef] [Google Scholar] [Publisher Link]
[15] Jitendra Kumar Jaiswal, and Rita Samikannu, “Application of Random Forest Algorithm on Feature Subset Selection and Classification and Regression,” 2017 World Congress on Computing and Communication Technologies (WCCCT), Tiruchirappalli, India, pp. 65-68, 2017.
[CrossRef] [Google Scholar] [Publisher Link]
[16] Shaik Rizwan et al., “A Compact Textile Monopole Antenna for Monitoring the Healing of Bone Fractures Using Unsupervised Machine Learning Algorithm,” IEEE Access, vol. 11, pp. 101195-101204, 2023.
[CrossRef] [Google Scholar] [Publisher Link]
[17] Jingchang Nan et al., “Design of UWB Antenna Based on Improved Deep Belief Network and Extreme Learning Machine Surrogate Models,” IEEE Access, vol. 9, pp. 126541-126549, 2021.
[CrossRef] [Google Scholar] [Publisher Link]
[18] Ahmed M. Montaser, “Machine Learning Based Design of Pattern Reconfigurable Antenna,” IEEE Access, vol. 11, pp. 33121-33133, 2023.
[CrossRef] [Google Scholar] [Publisher Link]
[19] Mohammad Mahmudul Hasan, and Michael Cheffena, “Adaptive Antenna Impedance Matching Using Low-Complexity Shallow Learning Model,” IEEE Access, vol. 11, pp. 74101-74111, 2023.
[CrossRef] [Google Scholar] [Publisher Link]
[20] Ibrahim N. Alquaydheb et al., “Modeling, Characterization, and Machine Learning Algorithm for Rectangular Choke Horn Antennas,” IEEE Access, vol. 12, pp. 61697-61707, 2024.
[CrossRef] [Google Scholar] [Publisher Link]