Exploring Sentiment and Emotion Analysis: A Systematic Review and Future Directions
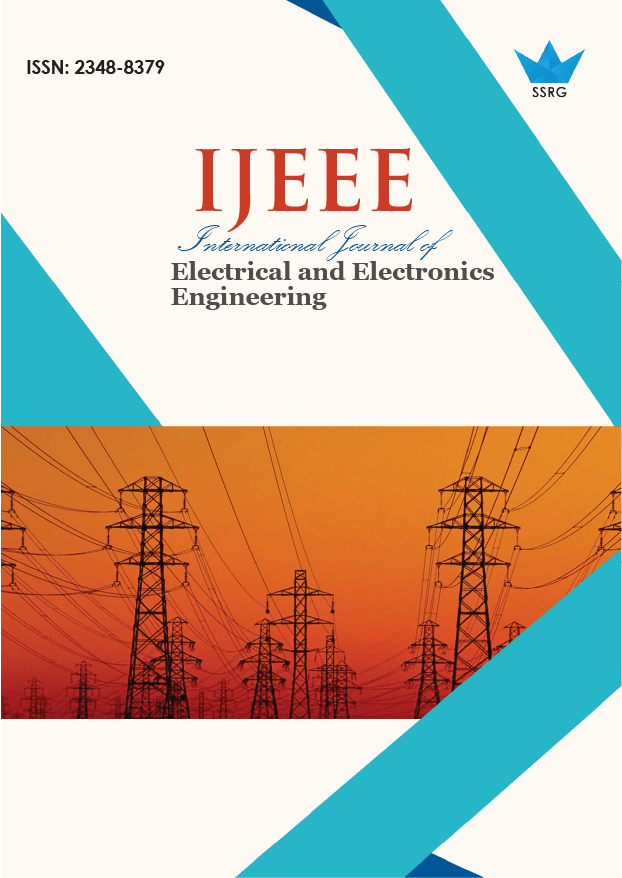
International Journal of Electrical and Electronics Engineering |
© 2024 by SSRG - IJEEE Journal |
Volume 11 Issue 12 |
Year of Publication : 2024 |
Authors : Nisha, Rakesh Kumar |
How to Cite?
Nisha, Rakesh Kumar, "Exploring Sentiment and Emotion Analysis: A Systematic Review and Future Directions," SSRG International Journal of Electrical and Electronics Engineering, vol. 11, no. 12, pp. 76-93, 2024. Crossref, https://doi.org/10.14445/23488379/IJEEE-V11I12P107
Abstract:
Human communication often carries emotional undertones expressed verbally, through written texts, and non-vocally via gestures and facial expressions. With the widespread adoption of texting and the rapid growth of social media, digital communication has become more prevalent. The Internet is crucial in the modern era of technology, providing a vast repository of information that necessitates thorough examination to extract relevant insights. Sentiment analysis, which involves computationally analyzing sentiments, viewpoints, and the subjective aspects of text, is crucial for uncovering hidden information and accurately classifying emotions. However, obtaining suitable datasets and applying precise classifiers pose significant challenges. The function of artificial intelligence technology in automated text sentiment analysis is the primary subject of this article, which also examines other uses, difficulties, and approaches to sentiment analysis. Drawing attention to obstacles, constraints, and potential avenues for further study adds to what is already known and helps academics and professionals choose the best approaches.
Keywords:
Emotion detection, Machine Learning, Natural Language Processing, Sentiment classification, Text analysis.
References:
[1] Anton Borg, and Martin Boldt, “Using VADER Sentiment and SVM for Predicting Customer Response Sentiment,” Expert Systems with Applications, vol. 162, 2020.
[CrossRef] [Google Scholar] [Publisher Link]
[2] Pansy Nandwani, and Rupali Verma, “A Review on Sentiment Analysis and Emotion Detection from Text,” Social Network Analysis and Mining, vol. 11, 2021.
[CrossRef] [Google Scholar] [Publisher Link]
[3] Klaifer Garcia, and Lilian Berton, “Topic Detection and Sentiment Analysis in Twitter Content Related to COVID-19 from Brazil and the USA,” Applied Soft Computing, vol. 101, 2021.
[CrossRef] [Google Scholar] [Publisher Link]
[4] Rui Ren, Desheng Dash Wu, and Tianxiang Liu, “Forecasting Stock Market Movement Direction Using Sentiment Analysis and Support Vector Machine,” IEEE Systems Journal, vol. 13, no. 1, pp. 760-770, 2019.
[CrossRef] [Google Scholar] [Publisher Link]
[5] T. Tulasi Sasidhar, Premjith B., and Soman K.P., “Emotion Detection in Hinglish (Hindi+English) Code-Mixed Social Media Text,” Procedia Computer Science, vol. 171, pp. 1346-1352, 2020.
[CrossRef] [Google Scholar] [Publisher Link]
[6] Alexander Ligthart, Cagatay Catal, and Bedir Tekinerdogan, “Systematic Reviews in Sentiment Analysis: A Tertiary Study,” Artificial Intelligence Review, vol. 54, pp. 4997-5053, 2021.
[CrossRef] [Google Scholar] [Publisher Link]
[7] Jamin Rahman Jim et al., “Recent Advancements and Challenges of NLP-Based Sentiment Analysis: A State-of-the-Art Review,” Natural Language Processing Journal, vol. 6, 2024.
[CrossRef] [Google Scholar] [Publisher Link]
[8] Bo Pang, Lillian Lee, and Shivakumar Vaithyanathan, “Thumbs up? Sentiment Classification Using Machine Learning Techniques,” Proceeding of the ACL-02 Conference on Empirical Methods in Natural Language Processing, vol. 10, pp. 79-86. 2002.
[CrossRef] [Google Scholar] [Publisher Link]
[9] Peter D. Turney, “Thumbs up or Thumbs down?: Semantic Orientation Applied to Unsupervised Classification of Reviews,” Proceedings of the 40th Annual Meeting on Association for Computational Linguistics, pp. 417-424, 2002.
[CrossRef] [Google Scholar] [Publisher Link]
[10] Qiang Ye, Ziqiong Zhang, and Rob Law, “Sentiment Classification of Online Reviews to Travel Destinations by Supervised Machine Learning Approaches,” Expert Systems with Applications, vol. 36, no. 3, pp. 6527-6535, 2009.
[CrossRef] [Google Scholar] [Publisher Link]
[11] Lal Khan et al., “Multi-Class Sentiment Analysis of Urdu Text Using Multilingual BERT,” Scientific Reports, vol. 12, 2022.
[CrossRef] [Google Scholar] [Publisher Link]
[12] Hewan Shrestha et al., “Natural Language Processing Based Sentimental Analysis of Hindi (SAH) Script an Optimization Approach,” International Journal of Speech Technology, vol. 23, pp. 757-766, 2020.
[CrossRef] [Google Scholar] [Publisher Link]
[13] Akshi Kumar, and Teeja Mary Sebastian, “Sentiment Analysis: A Perspective on its Past, Present and Future,” International Journal of Intelligent Systems and Applications, vol. 4, no. 10, pp. 1-14, 2012.
[CrossRef] [Google Scholar] [Publisher Link]
[14] Mayur Wankhade, Annavarapu Chandra Sekhara Rao, and Chaitanya Kulkarni, “A Survey on Sentiment Analysis Methods, Applications, and Challenges,” Artificial Intelligence Review, vol. 55, pp. 5731-5780, 2022.
[CrossRef] [Google Scholar] [Publisher Link]
[15] M. Devi Sri Nandhini, and G. Pradeep, “A Hybrid Co-Occurrence and Ranking-Based Approach for Detection of Implicit Aspects in Aspect-Based Sentiment Analysis,” SN Computer Science, vol. 1, no. 3, 2020.
[CrossRef] [Google Scholar] [Publisher Link]
[16] Mauro Dragoni, and Giulio Petrucci, “A Neural Word Embeddings Approach for Multi-Domain Sentiment Analysis,” IEEE Transactions on Affective Computing, vol. 8, no. 4, pp. 457-470, 2017.
[CrossRef] [Google Scholar] [Publisher Link]
[17] Ashima Yadav, and Dinesh Kumar Vishwakarma, “Sentiment Analysis Using Deep Learning Architectures: A Review,” Artificial Intelligence Review, vol. 53, no. 6, pp. 4335-4385, 2020.
[CrossRef] [Google Scholar] [Publisher Link]
[18] Ioannis Korkontzelos et al., “Analysis of the Effect of Sentiment Analysis on Extracting Adverse Drug Reactions from Tweets and Forum Posts,” Journal of Biomedical Informatics, vol. 62, pp. 148-158, 2016.
[CrossRef] [Google Scholar] [Publisher Link]
[19] Andreas Lommatzsch et al., “Towards the Automatic Sentiment Analysis of German News and Forum Documents,” Innovations for Community Services, pp. 18-33, 2017.
[CrossRef] [Google Scholar] [Publisher Link]
[20] Norah Saleh Alghamdi et al., “Predicting Depression Symptoms in an Arabic Psychological Forum,” IEEE Access, vol. 8, pp. 57317-57334, 2020.
[CrossRef] [Google Scholar] [Publisher Link]
[21] Zulfadzli Drus, and Haliyana Khalid, “Sentiment Analysis in Social Media and its Application: Systematic Literature Review,” Procedia Computer Science, vol. 161, pp. 707-714, 2019.
[CrossRef] [Google Scholar] [Publisher Link]
[22] Michelle Annett, and Grzegorz Kondrak, “A Comparison of Sentiment Analysis Techniques: Polarizing Movie Blogs,” Advances in Artificial Intelligence, pp. 25-35, 2008.
[CrossRef] [Google Scholar] [Publisher Link]
[23] Mondher Bouazizi, and Tomoaki Otsuki Ohtsuki, “A Pattern-Based Approach for Sarcasm Detection on Twitter,” IEEE Access, vol. 4, pp. 5477-5488, 2016.
[CrossRef] [Google Scholar] [Publisher Link]
[24] Abdullah Y. Muaad et al., “Arabic Document Classification: Performance Investigation of Preprocessing and Representation Techniques,” Mathematical Problems in Engineering, vol. 2022, no. 1, 2022.
[CrossRef] [Google Scholar] [Publisher Link]
[25] Steven Bird, Ewan Klein, and Edward Loper, Natural Language Processing with Python - Analyzing Text with the Natural Language Toolkit, O'Reilly, 2009.
[Google Scholar] [Publisher Link]
[26] P. Kathiravan, R. Saranya, and Sridurga Sekar, “Sentiment Analysis of COVID-19 Tweets Using TextBlob and Machine Learning Classifiers: An Evaluation to Show How COVID-19 Opinions Is Influencing Psychological Reactions of People’s Behavior in Social Media,” Proceedings of International Conference on Data Science and Applications, pp. 89-106, 2023.
[CrossRef] [Google Scholar] [Publisher Link]
[27] Ted Kwartler, Text Mining in Practice with R, John Wiley & Sons, Ltd, 2017.
[CrossRef] [Google Scholar] [Publisher Link]
[28] Christopher Manning et al., “The Stanford CoreNLP natural Language Processing Toolkit,” Proceedings of 52nd Annual Meeting of the Association for Computational Linguistics: System Demonstrations, pp. 55-60, 2014.
[CrossRef] [Google Scholar] [Publisher Link]
[29] Arfath Pasha et al., “MADAMIRA: A Fast, Comprehensive Tool for Morphological Analysis and Disambiguation of Arabic,” Proceedings of the Ninth International Conference on Language Resources and Evaluation (LREC'14), pp. 1094-1101, 2014.
[Google Scholar] [Publisher Link]
[30] Vaishali Kalra, and Rashmi Aggarwal, “Importance of Text Data Preprocessing & Implementation in RapidMiner,” Proceedings of the First International Conference on Information Technology and Knowledge Management, vol. 14, pp. 71-75, 2017.
[Google Scholar] [Publisher Link]
[31] Abdullah Ikram, Mohit Kumar, and Geetika Munjal, “Twitter Sentiment Analysis Using Machine Learning,” 2022 12th International Conference on Cloud Computing, Data Science & Engineering (Confluence), Noida, India, pp. 629-634, 2022.
[CrossRef] [Google Scholar] [Publisher Link]
[32] Ravinder Ahuja et al., “The Impact of Features Extraction on the Sentiment Analysis,” Procedia Computer Science, vol. 152, pp. 341-348, 2021.
[CrossRef] [Google Scholar] [Publisher Link]
[33] Yousif A. Alhaj et al., “A Study of the Effects of Stemming Strategies on Arabic Document Classification,” IEEE Access, vol. 7, pp. 32664-32671, 2019.
[CrossRef] [Google Scholar] [Publisher Link]
[34] Mohamed Elhag Mohamed Abo et al., “A Multi-Criteria Approach for Arabic Dialect Sentiment Analysis for Online Reviews: Exploiting Optimal Machine Learning Algorithm Selection,” Sustainability, vol. 13, no. 18, 2021.
[CrossRef] [Google Scholar] [Publisher Link]
[35] Despoina Antonakaki, Paraskevi Fragopoulou, and Sotiris Ioannidis, “A Survey of Twitter Research: Data Model, Graph Structure, Sentiment Analysis and Attacks,” Expert Systems with Applications, vol. 164, 2021.
[CrossRef] [Google Scholar] [Publisher Link]
[36] Bhumika Gupta et al., “Study of Twitter Sentiment Analysis Using Machine Learning Algorithms on Python,” International Journal of Computer Applications, vol. 165, no. 9, pp. 9-34, 2017.
[CrossRef] [Google Scholar] [Publisher Link]
[37] Nhan Cach Dang, María N. Moreno-García, and Fernando De la Prieta, “Sentiment Analysis Based on Deep Learning: A Comparative Study,” Electronics, vol. 9, no. 3, 2020.
[CrossRef] [Google Scholar] [Publisher Link]
[38] Htet Htet Htun, Michael Biehl, and Nicolai Petkov, “Survey of Feature Selection and Extraction Techniques for Stock Market Prediction,” Financial Innovation, vol. 9, 2023.
[CrossRef] [Google Scholar] [Publisher Link]
[39] Mohammed Kasri, Marouane Birjali, and Abderrahim Beni-Hssane, “A Comparison of Features Extraction Methods for Arabic Sentiment Analysis,” Proceedings of the 4th International Conference on Big Data and Internet of Things, pp. 1-6, 2019.
[[CrossRef] [Google Scholar] [Publisher Link]
[40] Tomas Mikolov et al., “Efficient Estimation of Word Representations in Vector Space,” arXiv, 2013.
[CrossRef] [Google Scholar] [Publisher Link]
[41] Jeffrey Pennington, Richard Socher, and Christopher Manning, “GloVe: Global Vectors for Word Representation,” Proceedings of the 2014 Conference on Empirical Methods in Natural Language Processing (EMNLP), pp. 1532-1543, 2014.
[CrossRef] [Google Scholar] [Publisher Link]
[42] Abdullah Y. Muaad et al., “ArCAR: A Novel Deep Learning Computer-Aided Recognition for Character-Level Arabic Text Representation and Recognition,” Algorithms, vol. 14, no. 7, 2021.
[CrossRef] [Google Scholar] [Publisher Link]
[43] Siti Rohaidaha Ahmad, Azuraliza Abub Bakar, and Mohd Ridzwanb Yaakub, “A Review of Feature Selection Techniques in Sentiment Analysis,” Intelligent Data Analysis, vol. 23, no. 1, pp. 159-189, 2019.
[CrossRef] [Google Scholar] [Publisher Link]
[44] Basant Agarwal, and Namita Mittal, “Optimal Feature Selection for Sentiment Analysis,” Computational Linguistics and Intelligent Text Processing, pp. 13-24, 2013.
[CrossRef] [Google Scholar] [Publisher Link]
[45] Yousef Seyfari, and Akbar Meimandi, “A New Approach to Android Malware Detection Using Fuzzy Logic-Based Simulated Annealing and Feature Selection,” Multimedia Tools and Applications, vol. 83, no. 4, pp. 10525-10549, 2024.
[CrossRef] [Google Scholar] [Publisher Link]
[46] Abdur Rasool et al., “GAWA-A Feature Selection Method for Hybrid Sentiment Classification,” IEEE Access, vol. 8, pp. 191850-191861, 2020.
[CrossRef] [Google Scholar] [Publisher Link]
[47] Ahmed Abbasi et al., “Selecting Attributes for Sentiment Classification Using Feature Relation Networks,” IEEE Transactions on Knowledge and Data Engineering, vol. 23, no. 3, pp. 447-462, 2011.
[CrossRef] [Google Scholar] [Publisher Link]
[48] Hailong Zhang, Wenyan Gan, and Bo Jiang, “Machine Learning and Lexicon Based Methods for Sentiment Classification: A Survey,” 2014 11th Web Information System and Application Conference, Tianjin, China, pp. 262-265, 2014.
[CrossRef] [Google Scholar] [Publisher Link]
[49] Soumya S., and Pramod K.V., “Sentiment Analysis of Malayalam Tweets Using Machine Learning Techniques,” ICT Express, vol. 6, no. 4, pp. 300-305, 2020.
[CrossRef] [Google Scholar] [Publisher Link]
[50] Gediminas Adomavicius, and YoungOk Kwon, “Improving Aggregate Recommendation Diversity Using Ranking Based Techniques,” IEEE Transactions on Knowledge and Data Engineering, vol. 24, no. 5, pp. 896-911, 2012.
[CrossRef] [Google Scholar] [Publisher Link]
[51] Noelia Sánchez-Maroño, Amparo Alonso-Betanzos, and Rosa M. Calvo-Estévez, “A Wrapper Method for Feature Selection in Multiple Classes Datasets,” Bio-Inspired Systems: Computational and Ambient Intelligence, pp. 456-463, 2009.
[CrossRef] [Google Scholar] [Publisher Link]
[52] Kang Leng Chiew et al., “A New Hybrid Ensemble Feature Selection Framework for Machine Learning Based Phishing Detection System,” Information Sciences, vol. 484, pp. 153-166, 2019.
[CrossRef] [Google Scholar] [Publisher Link]
[53] Maryam Bahojb Imani, Mohammad Reza Keyvanpour, and Reza Azmi, “A Novel Embedded Feature Selection Method: A Comparative Study in the Application of Text Categorization,” Applied Artificial Intelligence, vol. 27, no. 5, pp. 408-427, 2013.
[CrossRef] [Google Scholar] [Publisher Link]
[54] Rishabh Misra, and Prahal Arora, “Sarcasm Detection Using News Headlines Dataset,” AI Open, vol. 4, pp. 13-18, 2023.
[CrossRef] [Google Scholar] [Publisher Link]
[55] Erik Cambria et al., “Affective Computing and Sentiment Analysis,” A Practical Guide to Sentiment Analysis, pp 1-10, 2017.
[CrossRef] [Google Scholar] [Publisher Link]
[56] Ye Tian et al., “Facebook Sentiment: Reactions and Emojis,” Proceedings of the Fifth International Workshop on Natural Language Processing for Social Media, pp. 11-16, 2017.
[CrossRef] [Google Scholar] [Publisher Link]
[57] S.Ashika Parvin, M. Sumathi, and C. Mohan, “Challenges of Sentiment Analysis - A Survey,” 2021 5th International Conference on Trends in Electronics and Informatics (ICOEI), Tirunelveli, India, pp. 781-786, 2021.
[CrossRef] [Google Scholar] [Publisher Link]
[58] Arindam Chatterjere et al., “Minority Positive Sampling for Switching Points-An Anecdote for the Code-Mixing Language Modeling,” Proceedings of the 12th Language Resources and Evaluation Conference, pp. 6228-6236, 2020.
[Google Scholar] [Publisher Link]
[59] Anna Jurek, Maurice D. Mulvenna, and Yaxin Bi, “Improved Lexicon-Based Sentiment Analysis for Social Media Analytics,” Security Informatics, vol. 4, no. 1, 2015.
[CrossRef] [Google Scholar] [Publisher Link]
[60] Srishti Vashishtha, and Seba Susan, “Fuzzy Interpretation of Word Polarity Scores for Unsupervised Sentiment Analysis,” 2020 11th International Conference on Computing, Communication and Networking Technologies (ICCCNT), Kharagpur, India, pp. 1-6, 2020.
[CrossRef] [Google Scholar] [Publisher Link]
[61] Preeti Routray, Chinmaya Kumar Swain, and Smita Praya Mishra, “A Survey on Sentiment Analysis,” International Journal of Computer Applications, vol. 76, no. 10, pp. 1-8, 2013.
[CrossRef] [Google Scholar] [Publisher Link]
[62] Vijay Gupta, and Punam Rattan, “Improving Twitter Sentiment Analysis Efficiency with SVM-PSO Classification and EFWS Heuristic,” Procedia Computer Science, vol. 230, pp. 698-715, 2023.
[CrossRef] [Google Scholar] [Publisher Link]
[63] Jaspreet Singh, Gurvinder Singh, and Rajinder Singh, “Optimization of Sentiment Analysis Using Machine Learning Classifiers,” Human-Centric Computing and Information Sciences, vol. 7, no. 1, 2017.
[CrossRef] [Google Scholar] [Publisher Link]
[64] Joseph Max Kaufmann, “JMaxAlign: A Maximum Entropy Parallel Sentence Alignment Tool,” Proceedings of COLING: Demonstration papers, pp. 277-288, 2012.
[Google Scholar] [Publisher Link]
[65] Xin Xie et al., “An Improved Algorithm for Sentiment Analysis Based on Maximum Entropy,” Soft Computing, vol. 23, no. 2, pp. 599-611, 2019.
[CrossRef] [Google Scholar] [Publisher Link]
[66] Delali Kwasi Dake, and Esther Gyimah, “Using Sentiment Analysis to Evaluate Qualitative Students’ Responses,” Education and Information Technologies, vol. 28, no. 4, pp. 4629-4647, 2023.
[CrossRef] [Google Scholar] [Publisher Link]
[67] Miftahul Qorib et al., “COVID-19 Vaccine Hesitancy: Text Mining, Sentiment Analysis and Machine Learning on COVID-19 Vaccination Twitter Dataset,” Expert Systems with Applications, vol. 212, 2023.
[CrossRef] [Google Scholar] [Publisher Link]
[68] Chen Kewen et al., “Turning from TF-IDF to TF-IGM for Term Weighting in Text Classification,” Expert Systems with Applications, vol. 66, pp. 245-260, 2016.
[CrossRef] [Google Scholar] [Publisher Link]
[69] Zhi-Hong Deng, Kun-Hu Luo, and Hong-Liang Yu, “A Study of Supervised Term Weighting Scheme for Sentiment Analysis,” Expert Systems with Applications, vol. 41, no. 7, pp. 3506-3513, 2014.
[CrossRef] [Google Scholar] [Publisher Link]
[70] Alexandra Balahur, Jesús M. Hermida, and Andrés Montoyo, “Detecting Implicit Expressions of Emotion in Text: A Comparative Analysis,” Decision Support Systems, vol. 53, no.4, pp. 742-753, 2012.
[CrossRef] [Google Scholar] [Publisher Link]
[71] Fatemeh Zarisfi Kermani, Faramarz Sadeghi, and Esfandiar Eslami, “Solving the Twitter Sentiment Analysis Problem Based on a Machine Learning-Based Approach,” Evolutionary Intelligence, vol. 13, no. 3, pp. 381-398, 2020.
[CrossRef] [Google Scholar] [Publisher Link]
[72] Maryum Bibi et al., “A Cooperative Binary-Clustering Framework Based on Majority Voting for Twitter Sentiment Analysis,” IEEE Access, vol. 8, pp. 68580-68592, 2020.
[CrossRef] [Google Scholar] [Publisher Link]
[73] Mohammad A. Hassonah et al., “An Efficient Hybrid Filter and Evolutionary Wrapper Approach for Sentiment Analysis of Various Topics on Twitter,” Knowledge-Based Systems, vol. 192, 2020.
[CrossRef] [Google Scholar] [Publisher Link]
[74] Urooba Sehar et al., “Urdu Sentiment Analysis via Multimodal Data Mining Based on Deep Learning Algorithms,” IEEE Access, vol. 9, pp. 153072-153082, 2021.
[CrossRef] [Google Scholar] [Publisher Link]
[75] Carmel Mary Belinda M.J. et al., “Linguistic Analysis of Hindi-English Mixed Tweets for Depression Detection,” Journal of Mathematics, vol. 2022, no. 1, 2022.
[CrossRef] [Google Scholar] [Publisher Link]
[76] Erick Odhiambo Omuya, George Okeyo, and Michael Kimwele, “Sentiment Analysis on Social Media Tweets Using Dimensionality Reduction and Natural Language Processing,” Engineering Reports, vol. 5, no. 3, 2023.
[CrossRef] [Google Scholar] [Publisher Link]
[77] Ammar Mohammed, and Rania Kora, “An Effective Ensemble Deep Learning Framework for Text Classification,” Journal of King Saud University-Computer and Information Sciences, vol. 34, no. 10, pp. 8825-8837, 2022.
[CrossRef] [Google Scholar] [Publisher Link]
[78] Lin Xiang, “Application of an Improved TF-IDF Method in Literary Text Classification,” Advances in Multimedia, 2022.
[CrossRef] [Google Scholar] [Publisher Link]
[79] Yassine Al Amrani, Mohamed Lazaar, and Kamal Eddine El Kadiri, “Random Forest and Support Vector Machine Based Hybrid Approach to Sentiment Analysis,” Procedia Computer Science, vol. 127, pp. 511-520, 2018.
[CrossRef] [Google Scholar] [Publisher Link]
[80] Gagandeep Kaur, and Amit Sharma, “A Deep Learning-Based Model Using Hybrid Feature Extraction Approach for Consumer Sentiment Analysis,” Journal of Big Data, vol. 10, no. 1, 2023.
[CrossRef] [Google Scholar] [Publisher Link]
[81] Mrityunjay Singh, Amit Kumar Jakhar, and Shivam Pandey, “Sentiment Analysis on the Impact of Coronavirus in Social Life Using the BERT Model,” Social Network Analysis and Mining, vol. 11, 2021.
[CrossRef] [Google Scholar] [Publisher Link]
[82] Ravindra Kumar, Husanbir Singh Pannu, and Avleen Kaur Malhi, “Aspect-Based Sentiment Analysis Using Deep Networks and Stochastic Optimization,” Neural Computing and Applications, vol. 32, pp. 3221-3235, 2020.
[CrossRef] [Google Scholar] [Publisher Link]
[83] Faliang Huang et al., “Attention-Emotion-Enhanced Convolutional LSTM for Sentiment Analysis,” IEEE Transactions on Neural Networks and Learning Systems, vol. 33, no. 9, pp. 4332-4345, 2022.
[CrossRef] [Google Scholar] [Publisher Link]
[84] İrfan Aygün, Buket Kaya, and Mehmet Kaya, “Aspect Based Twitter Sentiment Analysis on Vaccination and Vaccine Types in COVID-19 Pandemic with Deep Learning,” IEEE Journal of Biomedical and Health Informatics, vol. 26, no. 5, pp. 2360-2369, 2022.
[CrossRef] [Google Scholar] [Publisher Link]
[85] Ahmed Sulaiman M. Alharbi, and Elise de Doncker, “Twitter Sentiment Analysis with a Deep Neural Network: An Enhanced Approach Using User Behavioral Information,” Cognitive Systems Research, vol. 54, pp. 50-61, 2019.
[CrossRef] [Google Scholar] [Publisher Link]
[86] Aarushi Vohra, and Ritu Garg, “Deep Learning Based Sentiment Analysis of Public Perception of Working from Home through Tweets,” Journal of Intelligent Information Systems, vol. 60, no. 1, pp. 255-274, 2023.
[CrossRef] [Google Scholar] [Publisher Link]
[87] K. Paramesha et al., “Sentiment Analysis on Cross-Domain Textual Data Using Classical and Deep Learning Approaches,” Multimedia Tools and Applications, vol. 82, no. 20, pp. 30759-30782, 2023.
[CrossRef] [Google Scholar] [Publisher Link]
[88] Rohitash Chandra, and Venkatesh Kulkarni, “Semantic and Sentiment Analysis of Selected Bhagavad Gita Translations Using BERT-Based Language Framework,” IEEE Access, vol. 10, pp. 21291-21315, 2022.
[CrossRef] [Google Scholar] [Publisher Link]
[89] Anam Yousaf et al., “Emotion Recognition by Textual Tweets Classification Using Voting Classifier (LR-SGD),” IEEE Access, vol. 9, pp. 6286-6295, 2020.
[CrossRef] [Google Scholar] [Publisher Link]
[90] Babacar Gaye, Dezheng Zhang, and Aziguli Wulamu, “A Tweet Sentiment Classification Approach Using a Hybrid Stacked Ensemble Technique,” Information, vol. 12, no. 9, 2021.
[CrossRef] [Google Scholar] [Publisher Link]
[91] Mehreen Naz, Kashif Zafar, and Ayesha Khan, “Ensemble Based Classification of Sentiments Using Forest Optimization Algorithm,” vol. 4, no. 2, 2019.
[CrossRef] [Google Scholar] [Publisher Link]
[92] Raymond Chiong, Gregorious Satia Budhi, and Sandeep Dhakal, “Combining Sentiment Lexicons and Content-Based Features for Depression Detection,” IEEE Intelligent Systems, vol. 36, no. 6, pp. 99-105, 2021.
[CrossRef] [Google Scholar] [Publisher Link]
[93] Naila Aslam et al., “Sentiment Analysis and Emotion Detection on Cryptocurrency Related Tweets Using Ensemble LSTM-GRU Model,” IEEE Access, vol. 10, pp. 39313-39324, 2022.
[CrossRef] [Google Scholar] [Publisher Link]
[94] Khursheed Aurangzeb, Nasir Ayub, and Musaed Alhussein, “Aspect Based Multi-Labeling Using SVM Based Ensembler,” IEEE Access, vol. 9, pp. 26026-26040, 2021.
[CrossRef] [Google Scholar] [Publisher Link]
[95] Ogobuchi Daniel Okey et al., “Investigating ChatGPT and Cybersecurity: A Perspective on Topic Modeling and Sentiment Analysis,” Computers & Security, vol. 135, 2023.
[CrossRef] [Google Scholar] [Publisher Link]
[96] Channabasava Chola et al., “Gender Identification and Classification of Drosophila Melanogaster Flies Using Machine Learning Techniques,” Computational and Mathematical Methods in Medicine, vol. 2022, 2022.
[CrossRef] [Google Scholar] [Publisher Link]
[97] Nivin A. Helal et al., “A Contextual-Based Approach for Sarcasm Detection,” Scientific Reports, vol. 14, 2024.
[CrossRef] [Google Scholar] [Publisher Link]