Integrating Human Pose Regression with Motion Analysis and Lightweight Edge Solutions for Advanced Pedestrian Detection
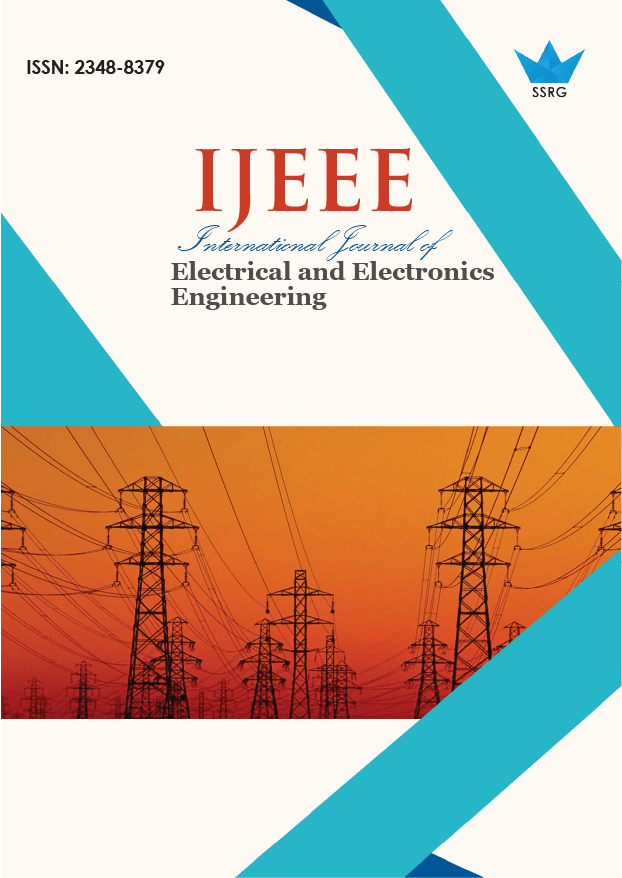
International Journal of Electrical and Electronics Engineering |
© 2024 by SSRG - IJEEE Journal |
Volume 11 Issue 12 |
Year of Publication : 2024 |
Authors : Priyanka Bagul, Shobha Sachin Nikam, Yogita Ajgar, Shilpa Prafull Khedkar |
How to Cite?
Priyanka Bagul, Shobha Sachin Nikam, Yogita Ajgar, Shilpa Prafull Khedkar, "Integrating Human Pose Regression with Motion Analysis and Lightweight Edge Solutions for Advanced Pedestrian Detection," SSRG International Journal of Electrical and Electronics Engineering, vol. 11, no. 12, pp. 94-99, 2024. Crossref, https://doi.org/10.14445/23488379/IJEEE-V11I12P108
Abstract:
Recent work has focused on combining human pose regression with complementary techniques to improve pedestrian detection. This method enhances pedestrian tracking and recognition by combining pose estimation, motion analysis and scene perception. A notable trend is the development of lightweight models suitable for edge devices that enable real-time detection in many real-world situations. Furthermore, methods such as domain adaptation and transfer learning are being investigated to enhance the ability of posture regression models to generalize to different datasets and environments. By combining motion analysis, visual perception and position regression, this work seeks to improve pedestrian detection by developing effective models for edge devices. The aim is to improve accuracy and real-time performance while guaranteeing compatibility with a wide range of practical applications through the use of posture estimation to record detailed body points and the development of models that work well on devices with limited processing resources.
Keywords:
Human pose regression, Pedestrian detection, Motion Analysis, Lightweight Edge Solutions.
References:
[1] Mrinal R. Bachute, and Javed M. Subhedar, “Autonomous Driving Architectures: Insights of Machine Learning and Deep Learning Algorithms,” Machine Learning with Applications, vol. 6, 2021.
[CrossRef] [Google Scholar] [Publisher Link]
[2] Yuxiao Zhang et al., “Perception and Sensing for Autonomous Vehicles Under Adverse Weather Conditions: A Survey,” ISPRS Journal of Photogrammetry and Remote Sensing, vol. 196, pp. 146-177, 2023.
[CrossRef] [Google Scholar] [Publisher Link]
[3] Yash Amol Rathod et al., “Energy Meter Tamper Detection and Alert Messaging System,” International Journal of Technology Engineering Arts Mathematics Science, vol. 1, no. 2, pp. 14-18, 2021.
[Google Scholar] [Publisher Link]
[4] K.U. Kadam et al., “Image Segmentation in Diagnosing the Ground Bud Necrosis Virus in Tomatoes Using K-Means Clustering,” International Journal of Electrical and Electronics Research (IJEER), vol. 11, no. 3, pp. 675-681, 2023.
[CrossRef] [Google Scholar] [Publisher Link]
[5] Shrikhande Rohini, and Vinayak Bairagi, “Lossless Medical Image Security,” International Journal of Applied Engineering Research, vol. 1, no. 3, pp. 536-541, 2010.
[Google Scholar] [Publisher Link]
[6] Mingyang Zhang et al., “Urban Anomaly Analytics: Description, Detection, and Prediction,” IEEE Transactions on Big Data, vol. 8, no. 3, pp. 809-826, 2022.
[CrossRef] [Google Scholar] [Publisher Link]
[7] Luiz G. Galvao et al., “Pedestrian and Vehicle Detection in Autonomous Vehicle Perception Systems-A Review,” Sensors, vol. 21, no. 21, pp. 1-47, 2021.
[CrossRef] [Google Scholar] [Publisher Link]
[8] Bassem Besbes et al., “Pedestrian Detection in Far-Infrared Daytime Images Using a Hierarchical Codebook of SURF,” Sensors, vol. 15, no. 4, pp. 8570-8594, 2015.
[CrossRef] [Google Scholar] [Publisher Link]
[9] Manli Zhu, Aleix M. Martinez, and Hong Z. Tan, “Template-Based Recognition of Static Sitting Postures,” 2003 Conference on Computer Vision and Pattern Recognition Workshop, Madison, WI, USA, vol. 5, 2003.
[CrossRef] [Google Scholar] [Publisher Link]
[10] Loris Nanni et al., “Coupling RetinaFace and Depth Information to Filter False Positives,” Applied Science, vol. 13, no. 5, pp. 1-14, 2023.
[CrossRef] [Google Scholar] [Publisher Link]
[11] Sundas Iftikhar et al., “Deep Learning-Based Pedestrian Detection in Autonomous Vehicles: Substantial Issues and Challenges,” Electronics, vol. 11, no. 21, pp. 1-23, 2022.
[CrossRef] [Google Scholar] [Publisher Link]
[12] Rui Li, and Yaxin Zu, “Research on Pedestrian Detection Based on the Multi-Scale and Feature-Enhancement Model,” Information, vol. 14, no. 2, pp. 1-14, 2023.
[CrossRef] [Google Scholar] [Publisher Link]
[13] Navneet Dalal, and Bill Triggs, “Histograms of Oriented Gradients for Human Detection,” 2005 IEEE Computer Society Conference on Computer Vision and Pattern Recognition (CVPR'05), San Diego, CA, USA, vol. 1, pp. 886-893, 2005.
[CrossRef] [Google Scholar] [Publisher Link]
[14] Bilel Tarchoun et al., “Hand-Crafted Features Vs Deep Learning for Pedestrian Detection in Moving Camera,” Signal Processing, vol. 37, no. 2, pp. 209-216, 2020.
[CrossRef] [Google Scholar] [Publisher Link]
[15] Navneet Dalal, and Bill Triggs, “Histograms of Oriented Gradients for Human Detection,” 2005 IEEE Computer Society Conference on Computer Vision and Pattern Recognition (CVPR'05), San Diego, CA, USA, vol. 1, pp. 886-893, 2005. [CrossRef] [Google Scholar] [Publisher Link]
[16] Huijing Zhao et al., “A Laser-Scanner-Based Approach Toward Driving Safety and Traffic Data Collection,” IEEE Transactions on Intelligent Transportation Systems, vol. 10, no. 3, pp. 534-546, 2009.
[CrossRef] [Google Scholar] [Publisher Link]
[17] Boyi Wang, “Research on Pedestrian Detection Algorithm Based on Image,” Journal of Physics: Conference Series, vol. 1345, no. 6, pp. 1-13, 2019.
[CrossRef] [Google Scholar] [Publisher Link]
[18] Liangchen Song et al., “Human Pose Estimation and Its Application to Action Recognition: A Survey,” Journal of Visual Communication and Image Representation, vol. 76, 2021.
[CrossRef] [Google Scholar] [Publisher Link]
[19] Esraa Samkari et al., “Human Pose Estimation Using Deep Learning: A Systematic Literature Review,” Machine Learning and Knowledge Extraction, vol. 5, no. 4, pp. 1612-1659, 2023.
[CrossRef] [Google Scholar] [Publisher Link]
[20] Ruoxin Xiong, and Pingbo Tang, “Pose Guided Anchoring for Detecting Proper Use of Personal Protective Equipment,” Automation in Construction, vol. 130, 2021.
[CrossRef] [Google Scholar] [Publisher Link]
[21] Xuefei Sun et al., “Pixel-Coordinate-Induced Human Pose High-Precision Estimation Method,” Electronics, vol. 12, no. 7, pp. 1-21, 2023.
[CrossRef] [Google Scholar] [Publisher Link]
[22] Wei Chen et al., “Occlusion and Multi-Scale Pedestrian Detection A Review,” Array, vol. 19, 2023.
[CrossRef] [Google Scholar] [Publisher Link]
[23] DuYeong Heo, Jae Yeal Nam, and Byoung Chul Ko, “Estimation of Pedestrian Pose Orientation Using Soft Target Training Based on Teacher-Student Framework,” Sensors, vol. 19, no. 5, 2019.
[CrossRef] [Google Scholar] [Publisher Link]
[24] Mingjing Li et al., “An Improved Lightweight Dense Pedestrian Detection Algorithm,” Applied Science, vol. 13, no. 15, pp. 1-15, 2023.
[CrossRef] [Google Scholar] [Publisher Link]
[25] Zehui Zhao et al., “A Comparison Review of Transfer Learning and Self-Supervised Learning: Definitions, Applications, Advantages and Limitations,” Expert Systems with Applications, vol. 242, 2024.
[CrossRef] [Google Scholar] [Publisher Link]