Performance Analysis of CBAM-Based Capsule Net for Neural Network for Speech Emotion Recognition
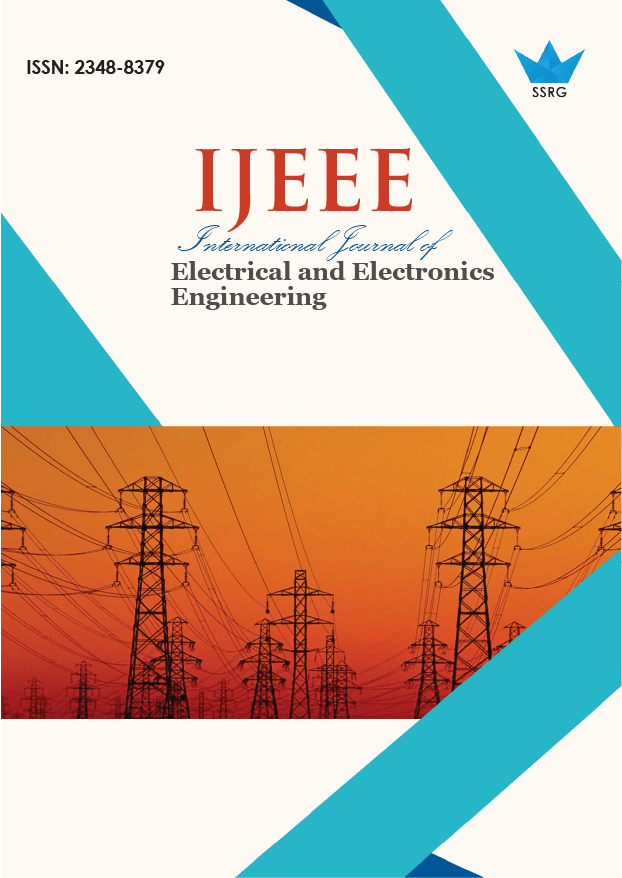
International Journal of Electrical and Electronics Engineering |
© 2024 by SSRG - IJEEE Journal |
Volume 11 Issue 12 |
Year of Publication : 2024 |
Authors : Nishant Barsainyan, Dileep Kumar Singh |
How to Cite?
Nishant Barsainyan, Dileep Kumar Singh, "Performance Analysis of CBAM-Based Capsule Net for Neural Network for Speech Emotion Recognition," SSRG International Journal of Electrical and Electronics Engineering, vol. 11, no. 12, pp. 243-254, 2024. Crossref, https://doi.org/10.14445/23488379/IJEEE-V11I12P122
Abstract:
Emotion recognition from audio signals is a challenging yet crucial task with applications in human-computer interaction, affective computing, and psychological research. This paper presents an innovative approach for audio emotion recognition, starting with a comprehensive pre-processing pipeline that integrates Infinite Impulse Response (IIR) filtering, MFCC, and Modified Emphasized Dynamic Com (MEDC). This combination surpasses conventional methods like MFCC and FFT by better isolating emotion-specific features and reducing noise. The paper introduces a novel architecture combining Capsule Networks (CapsNets) with a Convolutional Block Attention Module (CBAM). The CapsNet architecture, inspired by the human visual system, efficiently captures hierarchical spatial features and contextual dependencies, addressing the limitations of traditional CNN-based models. The integration of CBAM further refines the feature maps by emphasizing salient regions, improving emotion-related information extraction. The proposed system achieves an accuracy of 98.57% in recognizing emotions from audio data. Experimental results demonstrate the effectiveness of this approach on benchmark datasets, showing resilience to variations in voice quality, background noise, and speaker characteristics. A comparative analysis with traditional deep learning architectures and existing emotion recognition methods substantiates the CapsNet-CBAM model’s accuracy and computational efficiency superiority.
Keywords:
Emotion recognition, Convolutional block attention module, CapsNet, Accuracy, Computational time.
References:
[1] Zhiheng Xi et al., “The Rise and Potential of Large Language Model Based Agents: A Survey,” arXiv, 2023.
[CrossRef] [Google Scholar] [Publisher Link]
[2] Kat Roemmich, and Nazanin Andalibi, “Data Subjects' Conceptualizations of and Attitudes toward Automatic Emotion Recognition-Enabled Wellbeing Interventions on Social Media,” Proceedings of the ACM on Human-Computer Interaction, vol. 5, pp. 1-34, 2021.
[CrossRef] [Google Scholar] [Publisher Link]
[3] Fuzhen Zhuang et al., “A Comprehensive Survey on Transfer Learning,” Proceedings of the IEEE, vol. 109, no. 1, pp. 43-76, 2020.
[CrossRef] [Google Scholar] [Publisher Link]
[4] Jingyuan Zhao et al., “Specialized Deep Neural Networks for Battery Health Prognostics: Opportunities and Challenges,” Journal of Energy Chemistry, vol. 87, pp. 416-438, 2023.
[CrossRef] [Google Scholar] [Publisher Link]
[5] Alan Tan Wei Min, Abhishek Gupta, and Yew-Soon Ong, “Generalizing Transfer Bayesian Optimization to Source-Target Heterogeneity,” IEEE Transactions on Automation Science and Engineering, vol. 18, no. 4, pp. 1754-1765, 2021.
[CrossRef] [Google Scholar] [Publisher Link]
[6] Andrzej Janowski, “Natural Language Processing Techniques for Clinical Text Analysis in Healthcare,” Journal of Advanced Analytics in Healthcare Management, vol. 7, no. 1, pp. 51-76, 2023.
[Google Scholar] [Publisher Link]
[7] Fan Zhang et al., “Speech-Driven Personalized Gesture Synthetics: Harnessing Automatic Fuzzy Feature Inference,” IEEE Transactions on Visualization and Computer Graphics, vol. 10, no. 10, pp. 6984-6996, 2024.
[CrossRef] [Google Scholar] [Publisher Link]
[8] Yan Wang et al., “A Systematic Review on Affective Computing: Emotion Models, Databases, and Recent Advances,” Information Fusion, vol. 83-84, pp. 19-52, 2022.
[CrossRef] [Google Scholar] [Publisher Link]
[9] Sandeep Kumar et al., “Multilayer Neural Network Based Speech Emotion Recognition for Smart Assistance,” Computers, Materials & Continua, vol. 74, no. 1, pp. 1523-1540, 2023.
[CrossRef] [Google Scholar] [Publisher Link]
[10] A. Christy et al., “Multimodal Speech Emotion Recognition and Classification Using Convolutional Neural Network Techniques,” International Journal of Speech Technology, vol. 23, pp. 381-388, 2020.
[CrossRef] [Google Scholar] [Publisher Link]
[11] Ala Saleh Alluhaidan et al., “Speech Emotion Recognition through Hybrid Features and Convolutional Neural Network,” Applied Sciences, vol. 13, no. 8, 2023.
[CrossRef] [Google Scholar] [Publisher Link]
[12] Rashid Jahangir et al., “Convolutional Neural Network-based Cross-Corpus Speech Emotion Recognition with Data Augmentation and Features Fusion,” Machine Vision and Applications, vol. 33, no. 3, 2022.
[CrossRef] [Google Scholar] [Publisher Link]
[13] Kudakwashe Zvarevashe, and Oludayo O. Olugbara, “Recognition of Speech Emotion Using Custom 2D-Convolution Neural Network Deep Learning Algorithm,” Intelligent Data Analysis, vol. 24, no. 5, pp. 1065-1086, 2020.
[CrossRef] [Google Scholar] [Publisher Link]
[14] Shibani Hamsa et al., “Emotion Recognition from Speech Using Wavelet Packet Transform Cochlear Filter Bank and Random Forest Classifier,” IEEE Access, vol. 8, pp. 96994-97006, 2020.
[CrossRef] [Google Scholar] [Publisher Link]
[15] Chawki Barhoumi, and Yassine BenAyed, “Real-Time Speech Emotion Recognition Using Deep Learning and Data Augmentation,” vol. 58, no. 2, 2024.
[CrossRef] [Google Scholar] [Publisher Link]
[16] Mustaqeem Khan et al., “MSER: Multimodal Speech Emotion Recognition Using Cross-Attention with Deep Fusion,” Expert Systems with Applications, vol. 245, 2024.
[CrossRef] [Google Scholar] [Publisher Link]
[17] Mengsheng Wang et al., “Design of Smart Home System Speech Emotion Recognition Model based on Ensemble Deep Learning and Feature Fusion,” Applied Acoustics, vol. 218, 2024.
[CrossRef] [Google Scholar] [Publisher Link]
[18] Bachchu Paul et al., “Machine Learning Approach of Speech Emotions Recognition Using Feature Fusion Technique,” Multimedia Tools and Applications, vol. 83, no. 3, pp. 8663-8688, 2023.
[CrossRef] [Google Scholar] [Publisher Link]
[19] Geoffrey E. Hinton, Alex Krizhevsky, and Sida D. Wang, “Transforming Auto-Encoders,” Artificial Neural Networks and Machine Learning-ICANN 2011: 21st International Conference on Artificial Neural Networks, Espoo, Finland, Berlin Heidelberg, pp. 44-51, 2011.
[CrossRef] [Google Scholar] [Publisher Link]
[20] Sara Sabour, Nicholas Frosst, and Geoffrey E. Hinton, “Dynamic Routing between Capsules,” Advances in Neural Information Processing Systems, vol. 30, 2017.
[Google Scholar]
[21] Sanghyun Woo et al., “CBAM: Convolutional Block Attention Module,” Proceedings of the European Conference on Computer Vision (ECCV), pp. 3-19, 2018.
[Google Scholar] [Publisher Link]
[22] Yu Wang, Dejun Ning, and Songlin Feng, “A Novel Capsule Network Based on Wide Convolution and Multi-Scale Convolution for Fault Diagnosis,” Applied Sciences, vol. 10, no. 10, 2020.
[CrossRef] [Google Scholar] [Publisher Link]
[23] Yuni Zeng et al., “Spectrogram Based Multi-Task Audio Classification,” Multimedia Tools and Applications, vol. 78, pp. 3705-3722, 2019.
[CrossRef] [Google Scholar] [Publisher Link]
[24] Kunxia Wang et al., “Speech Emotion Recognition Using Fourier Parameters,” IEEE Transactions on Affective Computing, vol. 6, no. 1, pp. 69-75, 2015.
[CrossRef] [Google Scholar] [Publisher Link]
[25] Anjali Bhavan et al., “Bagged Support Vector Machines for Emotion Recognition from Speech,” Knowledge-Based Systems, vol. 184, 2019.
[CrossRef] [Google Scholar] [Publisher Link]
[26] Hua Zhang et al., “Pre-Trained Deep Convolution Neural Network Model with Attention for Speech Emotion Recognition,” Frontiers in Physiology, vol. 12, 2021.
[CrossRef] [Google Scholar] [Publisher Link]
[27] Samson Akinpelu, Serestina Viriri, and Adekanmi Adegun, “An Enhanced Speech Emotion Recognition Using Vision Transformer,” Scientific Reports, vol. 14, no. 1, 2024.
[CrossRef] [Google Scholar] [Publisher Link]