A Learning on Intensity Prediction of Tropical Cyclone Infrared Images by Gabor Filter on Binary and Multi Class Approach
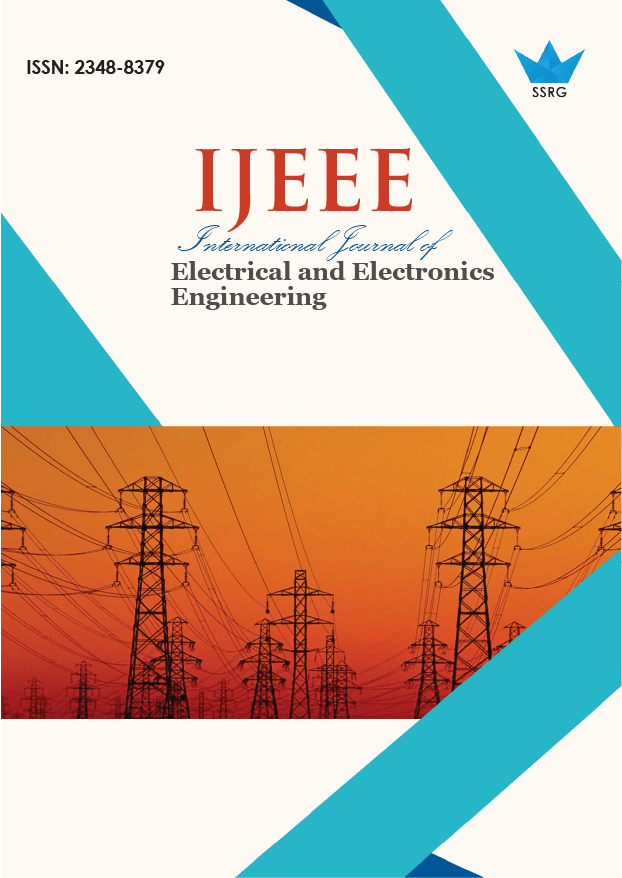
International Journal of Electrical and Electronics Engineering |
© 2024 by SSRG - IJEEE Journal |
Volume 11 Issue 12 |
Year of Publication : 2024 |
Authors : S. Jayasree, K.R. Ananthapadmanaban |
How to Cite?
S. Jayasree, K.R. Ananthapadmanaban, "A Learning on Intensity Prediction of Tropical Cyclone Infrared Images by Gabor Filter on Binary and Multi Class Approach," SSRG International Journal of Electrical and Electronics Engineering, vol. 11, no. 12, pp. 263-275, 2024. Crossref, https://doi.org/10.14445/23488379/IJEEE-V11I12P124
Abstract:
Tropical cyclone intensity classification is critical for disaster preparedness and resource allocation. Existing methods rely heavily on either manual analysis or computationally intensive deep learning models, which, despite their high accuracy, are often impractical for real-time scenarios. A significant gap exists in the literature where lightweight yet accurate models optimized for real-time applications are underexplored. This study addresses this gap by leveraging Gabor filter-based texture feature extraction combined with machine learning models, enabling precise cyclone intensity classification while balancing computational efficiency and prediction accuracy. By evaluating multiple classifiers, including Random Forest, SVM, and KNN, this study offers a comparative perspective to identify the most effective model for cyclone intensity classification in binary and multi-class setups.
Keywords:
Tropical cyclone, Bayes Net, KNN, SVM, Intensity.
References:
[1] Wei Tian et al., “Predicting the Intensity of Tropical Cyclones Over the Western North Pacific Using a Dual-Branch Spatiotemporal Attention Convolutional Network,” Weather and Forecasting, vol. 39, no. 5, pp. 807-819, 2024.
[CrossRef] [Google Scholar] [Publisher Link]
[2] Chang-Hoi Ho et al., “Geostationary Satellite–Derived Positioning of a Tropical Cyclone Center Using Artificial Intelligence Algorithms Over the Western North Pacific,” Bulletin of the American Meteorological Society, vol.105, no. 3, pp. E486-E500, 2024.
[CrossRef] [Google Scholar] [Publisher Link]
[3] M. Thenmozhi et al., “Assessment of Cyclone Risk and Case Study of Gaja Cyclone Using GIS Techniques and Machine Learning Algorithms in Coastal Zone of Tamil Nadu, India,” Environmental Research, vol. 246, 2024.
[CrossRef] [Google Scholar] [Publisher Link]
[4] Minki Choo et al., “Bridging Satellite Missions: Deep Transfer Learning for Enhanced Tropical Cyclone Intensity Estimation,” GIScience & Remote Sensing, vol. 61, no. 1,2024.
[CrossRef] [Google Scholar] [Publisher Link]
[5] M. Shahriar Sonet et al., “Assessing Tropical Cyclone Impacts in Coastal Bangladesh: A Change Detection Analysis on Cyclone Bulbul Using Geospatial Analysis and Remote Sensing Techniques,” International Journal of Disaster Risk Reduction, vol. 112, 2024.
[CrossRef] [Google Scholar] [Publisher Link]
[6] Wennan Wang et al., “A Multimodal Deep Learning Approach for Hurricane Tack Forecast Based on Encoder-Decoder Framework,” Pattern Analysis and Applications, vol. 27, no. 4, pp. 1-6, 2024.
[CrossRef] [Google Scholar] [Publisher Link]
[7] Tongfei Li et al., “Tropical Cyclone Trajectory Based on Satellite Remote Sensing Prediction and Time Attention Mechanism ConvLSTM Model,” Big Data Research, vol. 36, 2024.
[CrossRef] [Google Scholar] [Publisher Link]
[8] Mitra Nasimi, and Richard L. Wood, “Using Deep Learning and Advanced Image Processing for the Automated Estimation of Tornado-Induced Treefall,” Remote Sensing, vol. 16, no. 7, pp. 1-22, 2024.
[CrossRef] [Google Scholar] [Publisher Link]
[9] Jacob Senior-Williams et al., “The Classification of Tropical Storm Systems in Infrared Geostationary Weather Satellite Images Using Transfer Learning,” IEEE Journal of Selected Topics in Applied Earth Observations and Remote Sensing, vol. 17, pp. 5234-5244, 2024.
[CrossRef] [Google Scholar] [Publisher Link]
[10] Prachee Patra, Umakanta Das, and Sonam Agrawal, “Satellite Imagery-Based Tropical Cyclone Impact Assessment on LULC and Vegetation: A Case Study of Cyclone Biparjoy,” Environmental Monitoring and Assessment, vol. 196, 2024.
[CrossRef] [Google Scholar] [Publisher Link]
[11] Chuanhai Qian et al., “Tropical Cyclone Monitoring and Analysis Techniques: A Review,” Journal of Meteorological Research, vol. 8, pp. 351-367, 2024.
[CrossRef] [Google Scholar] [Publisher Link]
[12] Marwa S. Moustafa et al., “Cyclone Detection with End-to-End Super Resolution and Faster R-CNN,” Earth Science Informatics, vol. 17, pp. 1837-1850, 2024.
[CrossRef] [Google Scholar] [Publisher Link]
[13] Qi Yao et al., “Anti-Tropical Cyclone Load Reduction Control of Wind Turbines Based on Deep Neural Network Yaw Algorithm,” Applied Energy, vol. 376, 2024.
[CrossRef] [Google Scholar] [Publisher Link]
[14] Joshua May et al., “Automated Segmentation of Tropical Cyclone Clouds in Geostationary Infrared Images,” IEEE Geoscience and Remote Sensing Letters, vol. 21, 2024.
[CrossRef] [Google Scholar] [Publisher Link]
[15] Guangyi Ma et al., “An Improved Deep-Learning-Based Precipitation Estimation Algorithm Using Multitemporal GOES-16 Images,” IEEE Transactions on Geoscience and Remote Sensing, vol. 62, pp. 1-17, 2024.
[CrossRef] [Google Scholar] [Publisher Link]
[16] Juhyun Lee, Jungho Im, and Yeji Shin, “Enhancing Tropical Cyclone Intensity Forecasting with Explainable Deep Learning Integrating Satellite Observations and Numerical Model Outputs,” 50 iScience, vol. 27, no. 6, 2024.
[CrossRef] [Google Scholar] [Publisher Link]
[17] Han Wang et al., “Determination of Low-Intensity Tropical Cyclone Centers in Geostationary Satellite Images Using a Physics-Enhanced Deep-Learning Model,” IEEE Transactions on Geoscience and Remote Sensing, vol. 62, pp. 1-10, 2024. [CrossRef] [Google Scholar] [Publisher Link]
[18] Shanshan Mu et al., “High-Resolution Tropical Cyclone Rainfall Detection From C-Band SAR Imagery with Deep Learning,” IEEE Transactions on Geoscience and Remote Sensing, vol. 62, pp. 1-15, 2024.
[CrossRef] [Google Scholar] [Publisher Link]
[19] Asanobu Kitamoto et al., “Digital Typhoon: Long-Term Satellite Image Dataset for the Spatio-Temporal Modeling of Tropical Cyclones,” 37th Conference on Neural Information Processing Systems (NeurIPS), vol. 36, pp. 40623-40636, 2024.
[Google Scholar] [Publisher Link]
[20] Sarah M. Griffin, Anthony Wimmers, and Christopher S. Velden, “Predicting Short-Term Intensity Change in Tropical Cyclones Using a Convolutional Neural Network,” Weather and Forecasting, vol. 39, no. 1, pp. 177-202, 2024.
[CrossRef] [Google Scholar] [Publisher Link]
[21] Chang-Jiang Zhang et al., “Tropical Cyclone Tracking from Geostationary Infrared Satellite Images using Deep learning Techniques,” International Journal of Remote Sensing, vol. 45, no. 18, pp. 6324-6341, 2024.
[CrossRef] [Google Scholar] [Publisher Link]
[22] Zhitao Zhao et al., “MT-GN: Multi-Task-Learning-Based Graph Residual Network for Tropical Cyclone Intensity Estimation,” Remote Sensing, vol. 16, no. 2, pp. 1-22, 2024.
[CrossRef] [Google Scholar] [Publisher Link]
[23] L. Raynaud et al., “A Convolutional Neural Network for Tropical Cyclone Wind Structure Identification in Kilometer-Scale Forecasts,” Artificial Intelligence for the Earth Systems, vol. 3, no. 2, pp. 1-15, 2024.
[CrossRef] [Google Scholar] [Publisher Link]
[24] Hongxing Cui et al., “Modeling Ocean Cooling Induced by Tropical Cyclone Wind Pump Using Explainable Machine Learning Framework,” IEEE Transactions on Geoscience and Remote Sensing, vol. 62, pp. 1-17, 2024.
[CrossRef] [Google Scholar] [Publisher Link]
[25] Soumyajit Pal, Uma Das, and Oishila Bandyopadhyay, “SSN: A Novel CNN-Based Architecture for Classification of Tropical Cyclone Images From INSAT-3D,” IEEE Transactions on Geoscience and Remote Sensing, vol. 62, pp. 1-8, 2024.
[CrossRef] [Google Scholar] [Publisher Link]
[26] K. Bharathi, A. Archita, and S. Gandhimathi Alias Usha, Predicting Tropical Cyclones: A Supervised Machine Learning Approach, Predicting Natural Disasters with AI and Machine Learning, IGI Global, pp. 158-172, 2024.
[CrossRef] [Google Scholar] [Publisher Link]
[27] Xiaoxian Tian et al., “EasyRP-R-CNN: a Fast Cyclone Detection Model,” The Visual Computer, vol. 40, pp. 4829-4841, 2024.
[CrossRef] [Google Scholar] [Publisher Link]
[28] Li Liu et al., “Enhanced Rainfall Nowcasting of Tropical Cyclone by an Interpretable Deep Learning Model and Its Application in Real-time Flood Forecasting,” Journal of Hydrology, vol. 644, 2024.
[CrossRef] [Google Scholar] [Publisher Link]
[29] Rui Zhang et al., “Estimating Tropical Cyclone Intensity Using an STIA Model FROM Himawari-8 Satellite Images in the Western North Pacific Basin,” IEEE Transactions on Geoscience and Remote Sensing, vol. 62, pp. 1-13, 2024.
[CrossRef] [Google Scholar] [Publisher Link]
[30] Xiaohui L et al., “Transfer Learning-Based Generative Adversarial Network Model for Tropical Cyclone Wind Speed Reconstruction from SAR Images,” IEEE Transactions on Geoscience and Remote Sensing, vol. 62, pp. 1-16, 2024.
[CrossRef] [Google Scholar] [Publisher Link]
[31] Ryan Eusebi et al., “Realistic Tropical Cyclone Wind and Pressure Fields Can Be Reconstructed from Sparse Data Using Deep Learning,” Communications Earth & Environment, vol. 5, no. 8, pp. 1-10. 2024.
[CrossRef] [Google Scholar] [Publisher Link]
[32] Hirotaka Hachiya et al., “Multi-Class AUC Maximization for Imbalanced Ordinal Multi-Stage Tropical Cyclone Intensity Change Forecast,” Machine Learning with Applications, vol. 17, 2024.
[CrossRef] [Google Scholar] [Publisher Link]
[33] Manish Kumar Mawatwal, and Saurabh Das, “An End-to-End Deep Learning Framework for Cyclone Intensity Estimation in North Indian Ocean Region Using Satellite Imagery,” Journal of the Indian Society of Remote Sensing, vol. 52, pp. 2165-2175, 2024.
[CrossRef] [Google Scholar] [Publisher Link]
[34] Quan Nguye, and Chanh Kieu, “Predicting Tropical Cyclone Formation with Deep Learning,” Weather and. Forecasting, vol. 39, no. 1, pp. 241-258, 2024.
[CrossRef] [Google Scholar] [Publisher Link]
[35] Wei Tian et al., “TCIP-Net: Quantifying Radial Structure Evolution for Tropical Cyclone Intensity Prediction,” IEEE Transactions on Geoscience and Remote Sensing, vol. 62, pp. 1-14, 2024.
[CrossRef] [Google Scholar] [Publisher Link]
[36] Sshubam Verma, INSAT3D Infrared and Raw Cyclone Imagery (2012-2021), Kaggle, 2021. [Online]. Available: https://www.kaggle.com/datasets/sshubam/insat3d-infrared-raw-cyclone-images-20132021