Design and Validation of Mechanomyography and Torque Measurement Acquisition System for Skeletal Muscle Function
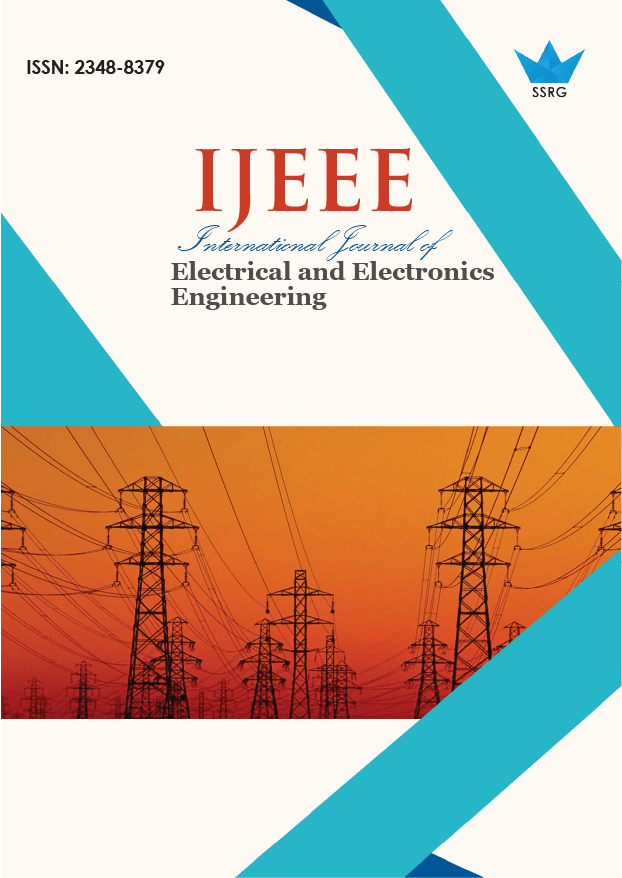
International Journal of Electrical and Electronics Engineering |
© 2024 by SSRG - IJEEE Journal |
Volume 11 Issue 12 |
Year of Publication : 2024 |
Authors : Raphael Uwamahoro, Kenneth Sundaraj, Farah Shahnaz Feroz |
How to Cite?
Raphael Uwamahoro, Kenneth Sundaraj, Farah Shahnaz Feroz, "Design and Validation of Mechanomyography and Torque Measurement Acquisition System for Skeletal Muscle Function," SSRG International Journal of Electrical and Electronics Engineering, vol. 11, no. 12, pp. 276-286, 2024. Crossref, https://doi.org/10.14445/23488379/IJEEE-V11I12P125
Abstract:
Assessment of muscle function is crucial for mitigating the risks of progressive motor weakness, which can ultimately lead to complete muscle impairment. However, existing technologies using commercial dynamometers are expensive, lack open-source availability and are not portable, limiting their accessibility in research settings. This study presents a cost-effective device to record muscle activity and the corresponding elbow joint torque. The device comprises three primary components:1) transducers for Mechanomyography (MMG), 2) torque signals detection, and 3) an application peripheral interface (API) for data acquisition control, visualization, and recording. Both transducers are integrated into an ATMEL ATMEGA 328. The device was validated on 36 able-bodied participants, measuring their MMG and torque across two sessions. Neuromuscular Electrical Stimulation (NMES) was applied to the Biceps Brachii (BB) muscle to induce elbow flexion. Further, submaximal torque and MMG were obtained using a commercial dynamometer and acceleration sensors for comparison. MMG measurements were observed at a maximum mean power frequency beyond 25Hz, while the torque information was found at 10 - 15 % of the Maximum Isometric Contraction (MVIC) induced by NMES. The measurement reliability was assessed using an Interclass Correlation Coefficient (ICC2,1) for elbow joint flexion torque (TQ RMS) and MMG RMS, yielding values between 0.522 and 0. 828. The ICC for the torque measurement device was 0.839, with SEM varying from 3.963 Nm to 11.149 Nm at a CV % of 2.565 to 13.123. These results underscore the potential of the developed device as a reliable, cost-effective alternative, with the added benefit of being replicable using locally available, low-cost electronics.
Keywords:
Mechanomyography, Joint torque, Muscle function, Tri-axis accelerometer.
References:
[1] Yurun Cai et al., “Motor and Physical Function Impairments as Contributors to Slow Gait Speed and Mobility Difficulty in Middle-Aged and Older Adults,” Journals of Gerontology - Series A, vol. 77, no. 8, pp. 1620-1628, 2022.
[CrossRef] [Google Scholar] [Publisher Link]
[2] E.K.J. Chadwick, and A.C. Nicol, “Elbow and Wrist Joint Contact Forces during Occupational Pick and Place Activities,” Journal of Biomechanics, vol. 33, no. 5, pp. 591-600, 2000.
[CrossRef] [Google Scholar] [Publisher Link]
[3] Lindsay Dowhan, “Comparison Between Handgrip Dynamometry and Manual Muscle Testing Performed by Registered Dietitians in Measuring Muscle Strength and Function of Hospitalized Patients,” Journal of Parenteral and Enteral Nutrition, vol. 40, no. 7, pp. 951-958, 2016.
[CrossRef] [Google Scholar] [Publisher Link]
[4] Katherine McNabb et al., “Handheld Dynamometry: Validity and Reliability of Measuring Hip Joint Rate of Torque Development and Peak Torque,” PLoS One, vol. 19, no. 8, 2024.
[CrossRef] [Google Scholar] [Publisher Link]
[5] Danuta Roman-Liu, and Maria Konarska, “Characteristics of Power Spectrum Density Function of EMG during Muscle Contraction Below 30%MVC,” Journal of Electromyography and Kinesiology, vol. 19, no. 5, pp. 864-874, 2009.
[CrossRef] [Google Scholar] [Publisher Link]
[6] Md. Anamul Islam et al., “Mechanomyogram for Muscle Function Assessment: A Review,” PLoS One, vol. 8, no. 3, 2013.
[CrossRef] [Google Scholar] [Publisher Link]
[7] Yichu Jin et al., “Estimation of Joint Torque in Dynamic Activities Using Wearable A-Mode Ultrasound,” Nature Communications, vol. 15, pp. 1-12, 2024.
[CrossRef] [Google Scholar] [Publisher Link]
[8] Jonathan de Oliveira et al., “Multi-Sensing Techniques with Ultrasound for Musculoskeletal Assessment: A Review,” Sensors, vol. 22, no. 23, 2022.
[CrossRef] [Google Scholar] [Publisher Link]
[9] Md. Anamul Islam et al., “Mechanomyography Sensor Development, Related Signal Processing, and Applications: A Systematic Review,” IEEE Sensors Journal, vol. 13, no. 7, pp. 2499-2516, 2013.
[CrossRef] [Google Scholar] [Publisher Link]
[10] T.W. Beck et al., “MMG-EMG Cross Spectrum and Muscle Fiber Type,” International Journal of Sports Medicine, vol. 30, no. 7, pp. 538-544, 2009.
[CrossRef] [Google Scholar] [Publisher Link]
[11] Eric D. Ryan et al., “Mechanomyographic Amplitude and Mean Power Frequency Responses during Isometric Ramp vs. Step Muscle Actions,” Journal of Neuroscience Method, vol. 168, no. 2, pp. 293-305, 2008.
[CrossRef] [Google Scholar] [Publisher Link]
[12] Travis W. Beck et al., “Does the Frequency Content of the Surface Mechanomyographic Signal Reflect Motor Unit Firing Rates? A Brief Review,” Journal of Electromyography and Kinesiology, vol. 17, no. 1, pp. 1-13, 2007.
[CrossRef] [Google Scholar] [Publisher Link]
[13] Travis W. Beck et al., “Comparison of a Piezoelectric Contact Sensor and an Accelerometer for Examining Mechanomyographic Amplitude and Mean Power Frequency Versus Torque Relationships During Isokinetic and Isometric Muscle Actions of the Biceps Brachii,” Journal of Electromyography and Kinesiology, vol. 16, no. 4, pp. 324-335, 2006.
[CrossRef] [Google Scholar] [Publisher Link]
[14] Guillaume Michaud et al., “Monitoring Neuromuscular Blockade at the Vastus Medialis Muscle Using Phonomyography,” Canadian Journal of Anesthesia, vol. 52, no. 8, pp. 795-800, 2015.
[CrossRef] [Google Scholar] [Publisher Link]
[15] Christopher Latella et al., “Test-Retest Reliability of Elbow Flexor Contraction Characteristics with Tensiomyography for Different Elbow Joint Angles,” Journal of Electromyography and Kinesiology, vol. 45, pp. 26-32, 2019.
[CrossRef] [Google Scholar] [Publisher Link]
[16] Syrpailyne Wankhar et al., “A Simple Method for Quantitative Assessment of Elbow Flexion Strength,” Journal of Medical Engineering & Technology, vol. 41, no. 7, pp. 529-533, 2017.
[CrossRef] [Google Scholar] [Publisher Link]
[17] Emiliano Cè et al., “Electromechanical Delay Components during Relaxation after Voluntary Contraction: Reliability and Effects of Fatigue,” Muscle & Nerve, vol. 51, no. 6, pp. 907-915, 2015.
[CrossRef] [Google Scholar] [Publisher Link]
[18] Corrado Cescon et al., “Two-Dimensional Spatial Distribution of Surface Mechanomyographical Response to Single Motor Unit Activity,” Journal of Neuroscience Methods, vol. 159, no. 1, pp. 19-25, 2007.
[CrossRef] [Google Scholar] [Publisher Link]
[19] Eddy Krueger et al., “Advances and Perspectives of Mechanomyography,” Brazilian Journal of Biomedical Engineering, vol. 30, no. 4, pp. 384-401, 2014.
[CrossRef] [Google Scholar] [Publisher Link]
[20] Niall Campbell, Thomas Egan, and Catherine Deegan, “The Application of Digital Accelerometers for Wired and Non-Wired Mechanomyography,” 2017 28th Irish Signals and Systems Conference (ISSC), Killarney, Ireland, pp. 1-6. 2017.
[CrossRef] [Google Scholar] [Publisher Link]
[21] ADXL313, Analog Devices. [Online]. Available: https://www.analog.com/en/products/adxl313.html
[22] Morufu Olusola Ibitoye et al., “Estimation of Electrically-Evoked Knee Torque from Mechanomyography Using Support Vector Regression,” Sensors, vol. 16, no. 7, pp. 1-16, 2016.
[CrossRef] [Google Scholar] [Publisher Link]
[23] MEAS FS20, Compression Load Cell-0.5-4.5V, TE Connectivity. [Online]. Available: https://www.te.com/en/product-CAT-FSE0004.html
[24] Hemmerling, Thomas M et al., “Phonomyography and Mechanomyography can be Used Interchangeably to Measure Neuromuscular Block at the Adductor Pollicis Muscle,” Anesthesia & Analgesia, vol. 98, no. 2, pp. 377-381, 2004.
[CrossRef] [Google Scholar] [Publisher Link]
[25] Brent M. Kelln et al., “Hand-Held Dynamometry: Reliability of Lower Extremity Muscle Testing in Healthy, Physically Active, Young Adults,” Journal of Sport Rehabilitation, vol. 17, no. 2, pp. 160-170, 2008.
[CrossRef] [Google Scholar] [Publisher Link]
[26] Claire Meagher et al., “New Advances in Mechanomyography Sensor Technology and Signal Processing: Validity and Interrater Reliability of Recordings from Muscle,” Journal of Rehabilitation and Assistive Technologies Engineering, vol. 7, 2020.
[CrossRef] [Google Scholar] [Publisher Link]
[27] FS20 Low Force Compression Load Cell, TE Connectivity, 2020. [Online]. Available: https://www.te.com/commerce/DocumentDelivery/DDEController?Action=showdoc&DocId=Data+Sheet%7FFS20%7FA8%7Fpdf%7FEnglish%7FENG_DS_FS20_A8.pdf%7FCAT-FSE0004
[28] Irsa Talib, Kenneth Sundaraj, and Chee Kiang Lam, “Analysis of the Crosstalk in Mechanomyographic Signals Along the Longitudinal, Lateral and Transverse Axes of Elbow Flexor Muscles During Sustained Isometric Forearm Flexion, Supination and Pronation Exercises,” Journal of Musculoskelet Neuronal Interact, vol. 20, no. 2, pp. 194-205, 2020.
[Google Scholar] [Publisher Link]
[29] Vincent Caputo BS et al., “The Utility of the Biceps Palpation-Rotation Test in Diagnosing Partial Distal Biceps Tendon Tears,” Journal of Shoulder and Elbow Surgery, vol. 31, no. 8, pp. 1603-1609 ,2022.
[CrossRef] [Google Scholar] [Publisher Link]
[30] Christian Klauer et al., “Feedback Control of Arm Movements Using Neuro-Muscular Electrical Stimulation (Nmes) Combined with a Lockable, Passive Exoskeleton for Gravity Compensation,” Frontiers in Neuroscience, vol. 8, pp. 1-16, 2014.
[CrossRef] [Google Scholar] [Publisher Link]
[31] Sharon R Perry et al., “Mean Power Frequency and Amplitude of the Mechanomyographic and Electromyographic Signals during Incremental Cycle Ergometry,” Journal of Electromyography and Kinesiology, vol. 11, no. 4, pp. 299-305, 2001.
[CrossRef] [Google Scholar] [Publisher Link]
[32] Tiantong Wang, Yunbiao Zhao, and Qining Wang, “A Wearable Co-Located Neural-Mechanical Signal Sensing Device for Simultaneous Bimodal Muscular Activity Detection,” IEEE Transactions on Biomedical Engineering, vol. 70, no. 12, pp. 3401 - 3412, 2023.
[CrossRef] [Google Scholar] [Publisher Link]
[33] Bertrand Diemont et al., “Spectral Analysis of Muscular Sound at Low and High Contraction Level,” International Journal of Bio-Medical Computing, vol. 23, no. 3-4, pp. 161-175, 1988.
[CrossRef] [Google Scholar] [Publisher Link]
[34] Sungwoo Park et al., “Design and Validation of a Wearable Dynamometry System for Knee Extension-Flexion Torque Measurement,” Scientific Reports, vol. 14, no. 1, 2024.
[CrossRef] [Google Scholar] [Publisher Link]
[35] David Fernández Hernando, Mark Elkins, and Ana Paula Coelho Figueira Freire, “Reporting of Confidence Intervals, Achievement of Intended Sample Size, and Adjustment for Multiple Primary Outcomes in Randomised Trials of Physical Therapy Interventions: an Analysis of 100 Representatively Sampled Trials, Brazilian Journal of Physical Therapy, vol. 28, no. 3, 2024.
[CrossRef] [Google Scholar] [Publisher Link]
[36] Norihiro Shima, Chris J. McNeil, and Charles L. Rice, “Mechanomyographic and electromyographic Responses to Stimulated and Voluntary Contractions in the Dorsiflexors of Young and Old Men,” Muscle & Nerve: Official Journal of the American Association of Electrodiagnostic Medicine, vol. 35, no. 3, pp. 371-378, 2007.
[CrossRef] [Google Scholar] [Publisher Link]
[37] Elgison Santos et al., “Influence of Sensor Mass and Adipose Tissue on the Mechanomyography Signal of Elbow Flexor Muscles,” Journal of Biomechanics, vol. 122, 2021.
[CrossRef] [Google Scholar] [Publisher Link]
[38] Muhammad Imran Ramli et al., “Accessory Respiratory Muscles Performance Among People with Spinal Cord Injury While Singing Songs with Different Musical Parameters,” PLoS One, vol. 19, no. 7, 2024.
[CrossRef] [Google Scholar] [Publisher Link]
[39] Samuel Wilson, and Ravi Vaidyanathan, “Upper-Limb Prosthetic Control Using Wearable Multichannel Mechanomyography,” 2017 International Conference on Rehabilitation Robotics (ICORR), London, UK, pp. 1293-1298, 2017.
[CrossRef] [Google Scholar] [Publisher Link]
[40] Yue Zhang, and Chunming Xia, “A Preliminary Study of Classification of Upper Limb Motions and Forces Based on Mechanomyography,” Medical Engineering & Physics, vol. 81, pp. 97-104, 2020.
[CrossRef] [Google Scholar] [Publisher Link]
[41] Hannah Christine Hund, Mark John Rice and Jesse Ehrenfeld, “An Evaluation of the State of Neuromuscular Blockade Monitoring Devices,” Journal of Medical Systems, vol. 40, no. 12, 2016.
[CrossRef] [Google Scholar] [Publisher Link]
[42] S Shogo Watanabe et al., “Electrically Induced Mechanomyograms Reflect Inspiratory Muscle Strength in Young or Elderly Subjects,” Respiratory Investigation, vol. 54, no. 6, pp. 436-444, 2016.
[CrossRef] [Google Scholar] [Publisher Link]
[43] Fabian Guenzkofer, F. Bubb Engstler, and Klaus Bengler, “Isometric Elbow Flexion and Extension Joint Torque Measurements Considering Biomechanical Aspects,” 1st International Symposium on Digital Human Modeling, pp. 14-15, 2011.
[Google Scholar] [Publisher Link]
[44] T.W. Beck et al., “Comparison of Fourier and Wavelet transform Procedures for examining the Mechanomyographic and Electromyographic Frequency Domain Responses during Fatiguing Isokinetic Muscle Actions of the Biceps Brachii,” Journal of Electromyography and Kinesiology, vol. 15, no. 2, pp. 190-199, 2005.
[CrossRef] [Google Scholar] [Publisher Link]
[45] Natasha Alves, and Tom Chau, “Automatic Detection of Muscle Activity from Mechanomyogram Signals: A Comparison of Amplitude and Wavelet-Based Methods,” Physiological Measurement, vol. 31, no. 4, p. 461, 2010.
[CrossRef] [Google Scholar] [Publisher Link]
[46] Siming Zuo et al., “Integrated Pico-Tesla Resolution Magnetoresistive Sensors for Miniaturised Magnetomyography,” In 42nd Annual International Conference of the IEEE Engineering in Medicine & Biology Society (EMBC), Montreal, QC, Canada, pp. 3415-3419, 2020.
[CrossRef] [Google Scholar] [Publisher Link]
[47] Yurong Li et al., “Neural Network-Based Modeling and Control of Elbow Joint Motion Under Functional Electrical Stimulation,” Neurocomputing, vol. 340, pp. 171-179, 2019.
[CrossRef] [Google Scholar] [Publisher Link]