Hybrid Wavelet-LSTM-Transformer Model for Fault Forecasting in Power Grids
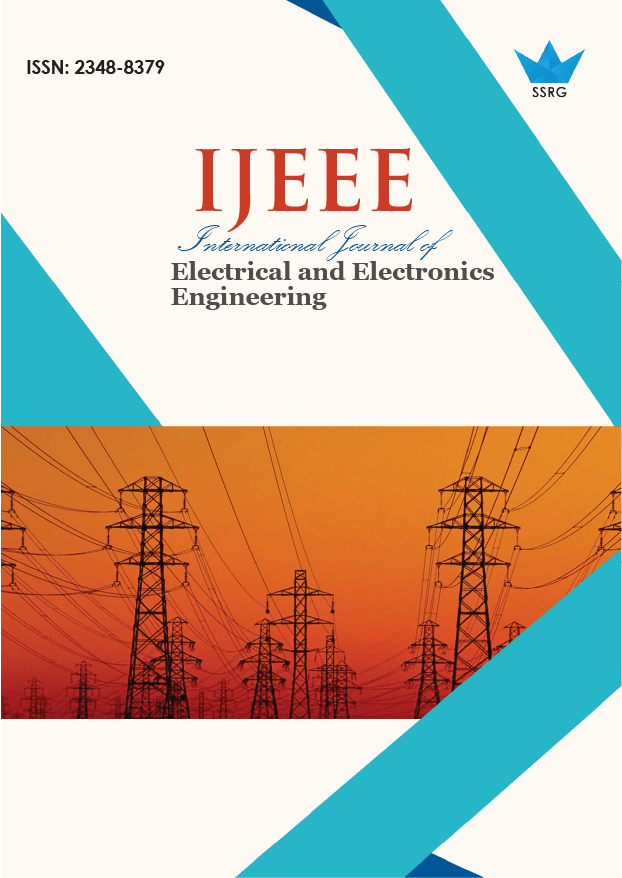
International Journal of Electrical and Electronics Engineering |
© 2024 by SSRG - IJEEE Journal |
Volume 11 Issue 12 |
Year of Publication : 2024 |
Authors : Diaa Salman, Cem Direkoglu, Nabeel Altanneh, Abdulaziz Ahmed |
How to Cite?
Diaa Salman, Cem Direkoglu, Nabeel Altanneh, Abdulaziz Ahmed, "Hybrid Wavelet-LSTM-Transformer Model for Fault Forecasting in Power Grids," SSRG International Journal of Electrical and Electronics Engineering, vol. 11, no. 12, pp. 314-326, 2024. Crossref, https://doi.org/10.14445/23488379/IJEEE-V11I12P130
Abstract:
In power grid management, accurate forecasting of faults is the key to providing uninterrupted electricity supply. Errors can result in significant losses of property as well as severe harm. Better alternatives adhere to the complex power grid data that frequently confuse conventional forecasting techniques and produce less-than-ideal forecasts. This research proposes a novel hybrid Wavelet-LSTM-Transformer model for power grid fault prediction. Long Short-Term Memory (LSTM) network sequential learning capabilities, attention processes of the Transformer model, and time-frequency analysis capabilities of wavelet transform are combined in the suggested model. In order to forecast trends, our approach maintains the notion of long-term memory while capturing short-term variations. This paper demonstrates that the developed model outperforms the most comparable work using numerous experimental trials and comparisons. It offers a workable method to boost the worldwide resilience and dependability of power grid systems. The results stress combining several modeling techniques to tackle difficult forecasting problems in important infrastructure sectors.
Keywords:
Fault forecasting, Deep Learning, Hybrid model, Power grid.
References:
[1] Hamid Reza Baghaee et al., “Support Vector Machine-Based Islanding and Grid Fault Detection in Active Distribution Networks,” IEEE Journal of Emerging and Selected Topics in Power Electronics, vol. 8, no. 3, pp. 2385-2403, 2020.
[CrossRef] [Google Scholar] [Publisher Link]
[2] Muhammad Sarwar et al., “High Impedance Fault Detection and Isolation in Power Distribution Networks Using Support Vector Machines,” Journal of King Saud University - Engineering Sciences, vol. 32, no. 8, pp. 524-535, 2020.
[CrossRef] [Google Scholar] [Publisher Link]
[3] Oktoviano Gandhi et al., “Review of Power System Impacts at High PV Penetration Part I: Factors Limiting PV Penetration,” Solar Energy, vol. 210, pp. 181-201, 2020.
[CrossRef] [Google Scholar] [Publisher Link]
[4] Ali Raza et al., “A Review of Fault Diagnosing Methods in Power Transmission Systems,” Applied Sciences, vol. 10, no. 4, 2020.
[CrossRef] [Google Scholar] [Publisher Link]
[5] Yanlin Shi, Sixian Tang, and Jackie Li, “A Two-Population Extension of the Exponential Smoothing State Space Model with a Smoothing Penalisation Scheme,” Risks, vol. 8, no. 3, 2020.
[CrossRef] [Google Scholar] [Publisher Link]
[6] Senlin Zhang et al., “Data-Based Line Trip Fault Prediction in Power Systems Using LSTM Networks and SVM,” IEEE Access, vol. 6, pp. 7675–7686, 2017.
[CrossRef] [Google Scholar] [Publisher Link]
[7] Ling Zheng et al., “A Fault Prediction of Equipment based on CNN-LSTM Network,” 2019 IEEE International Conference on Energy Internet (ICEI), Nanjing, China, pp. 537-541, 2019.
[CrossRef] [Google Scholar] [Publisher Link]
[8] Stéfano Frizzo Stefenon et al., “Wavelet Group Method of Data Handling for Fault Prediction in Electrical Power Insulators,” International Journal of Electrical Power & Energy Systems, vol. 123, 2020.
[CrossRef] [Google Scholar] [Publisher Link]
[9] Asiye K. Ozcanli, Fatma Yaprakdal, and Mustafa Baysal, “Deep Learning Methods and Applications for Electrical Power Systems: A Comprehensive Review,” International Journal of Energy Research, vol. 44, no. 9, pp. 7136-7157, 2020.
[CrossRef] [Google Scholar] [Publisher Link]
[10] A.A.P. Bíscaro et al., “Integrated Fault Location and Power-Quality Analysis in Electric Power Distribution Systems,” IEEE Transactions on Power Delivery, vol. 31, no. 2, pp. 428–436, 2016.
[CrossRef] [Google Scholar] [Publisher Link]
[11] Cynthia M. Furse et al., “Fault Diagnosis for Electrical Systems and Power Networks: A Review,” IEEE Sensors Journal, vol. 21, no. 2, pp. 888-906, 2021.
[CrossRef] [Google Scholar] [Publisher Link]
[12] Chen Haomin et al., “Fault Prediction for Power System based on Multidimensional Time Series Correlation Analysis,” 2014 China International Conference on Electricity Distribution (CICED), Shenzhen, pp. 1294-1299, 2014.
[CrossRef] [Google Scholar] [Publisher Link]
[13] Charith Silva, and Mohamad Saraee, “Electricity Distribution Network: Seasonality and the Dynamics of Equipment Failures Related Network Faults,” 2020 Advances in Science and Engineering Technology International Conferences (ASET), Dubai, United Arab Emirates, 2020.
[CrossRef] [Google Scholar] [Publisher Link]
[14] Hendrik J. Groenewald, Marius Kleingeld, and Gerrit J. Cloete, “An Autoregressive Fault Model for Condition Monitoring of Electrical Machines in Deep-Level Mines,” 2018 International Conference on the Industrial and Commercial Use of Energy (ICUE), Cape Town, South Africa, 2018.
[Google Scholar] [Publisher Link]
[15] A.V. Koteswara Rao et al., “An Exponential Smoothing based Power Swing Detection Technique for Distance Protection,” 2018 2nd IEEE International Conference on Power Electronics, Intelligent Control and Energy Systems (ICPEICES), Delhi, India, pp. 182-187, 2018.
[CrossRef] [Google Scholar] [Publisher Link]
[16] Danilo Pinto Moreira de Souza, Eliane Da Silva Christo, and Aryfrance Rocha Almeida, “Location of Faults in Power Transmission Lines Using the ARIMA Method,” Energies, vol. 10, no. 10, 2017.
[CrossRef] [Google Scholar] [Publisher Link]
[17] Moath Alrifaey, Wei Hong Lim, and Chun Kit Ang, “A Novel Deep Learning Framework Based RNN-SAE for Fault Detection of Electrical Gas Generator,” IEEE Access, vol. 9, pp. 21433-21442, 2021.
[CrossRef] [Google Scholar] [Publisher Link]
[18] Veerapandiyan Veerasamy et al., “LSTM Recurrent Neural Network Classifier for High Impedance Fault Detection in Solar PV Integrated Power System,” IEEE Access, vol. 9, pp. 32672-32687, 2021.
[CrossRef] [Google Scholar] [Publisher Link]
[19] Ailing Zeng et al., “Are Transformers Effective for Time Series Forecasting?,” Proceedings of the AAAI Conference on Artificial Intelligence, vol. 37, no. 9, pp. 11121-11128, 2023.
[CrossRef] [Google Scholar] [Publisher Link]
[20] Jibin B. Thomas et al., “CNN-Based Transformer Model for Fault Detection in Power System Networks,” IEEE Transactions on Instrumentation and Measurement, vol. 72, pp. 1-10, 2023.
[CrossRef] [Google Scholar] [Publisher Link]
[21] Bin Liao, You Du, and Xiangyun Yin, “Fusion of Infrared-Visible Images in UE-IoT for Fault Point Detection Based on GAN,” IEEE Access, vol. 8, pp. 79754-79763, 2020.
[CrossRef] [Google Scholar] [Publisher Link]
[22] Vikram Bali, Ajay Kumar, and Satyam Gangwar, “A Novel Approach for Wind Speed Forecasting Using LSTM-ARIMA Deep Learning Models,” International Journal of Agricultural and Environmental Information Systems (IJAEIS), vol. 11, no. 3, pp. 13-30, 2020.
[CrossRef] [Google Scholar] [Publisher Link]
[23] Arash Moradzadeh et al., “Hybrid CNN-LSTM Approaches for Identification of Type and Locations of Transmission Line Faults,” International Journal of Electrical Power & Energy Systems, vol. 135, 2022.
[CrossRef] [Google Scholar] [Publisher Link]
[24] Nathielle Waldrigues Branco et al., “Wavelet LSTM for Fault Forecasting in Electrical Power Grids,” Sensors, vol. 22, no. 21, 2022.
[CrossRef] [Google Scholar] [Publisher Link]
[25] M. Sifuzzaman, M.R. Islam, and M.Z. Ali, “Application of Wavelet Transform and its Advantages Compared to Fourier Transform,” Journal of Physical Sciences, vol. 13, pp. 121-134, 2009.
[Google Scholar] [Publisher Link]
[26] Xuan-Hien Le et al., “Application of Long Short-Term Memory (LSTM) Neural Network for Flood Forecasting,” Water, vol. 11, no. 7, 2019.
[CrossRef] [Google Scholar] [Publisher Link]
[27] André Veltman, Duco W.J. Pulle, and R.W. De Doncker, Fundamentalsof Electrical Drives, 2nd ed., Power Systems, Springer, pp. 47-82, 2016.
[Google Scholar] [Publisher Link]