Improved Stochastic Gradient Descent-Decision Tree (ISGD-DT) Framework for Intelligent Heart Disease Prediction
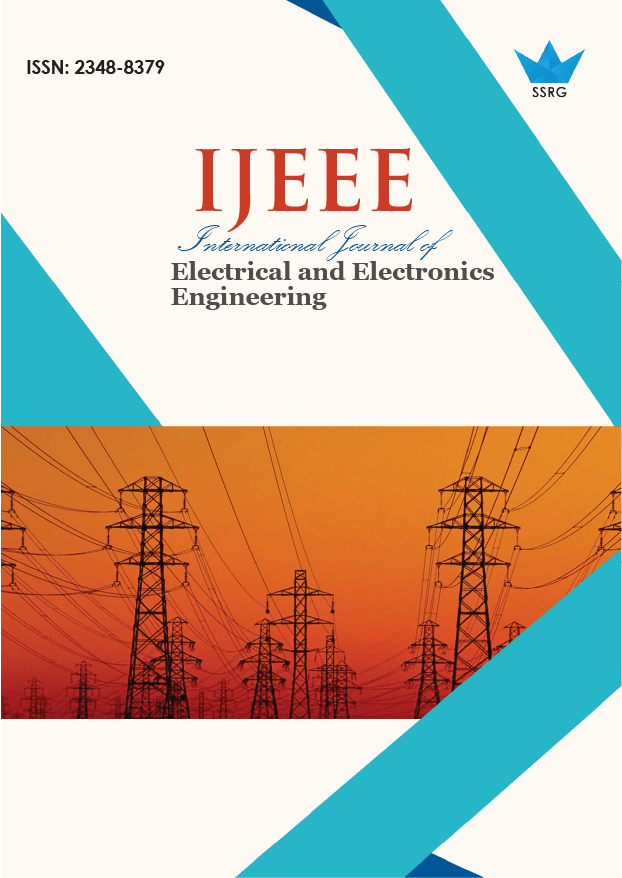
International Journal of Electrical and Electronics Engineering |
© 2024 by SSRG - IJEEE Journal |
Volume 11 Issue 12 |
Year of Publication : 2024 |
Authors : Bollapalli Althaph, Nagendra Panini Challa |
How to Cite?
Bollapalli Althaph, Nagendra Panini Challa, "Improved Stochastic Gradient Descent-Decision Tree (ISGD-DT) Framework for Intelligent Heart Disease Prediction," SSRG International Journal of Electrical and Electronics Engineering, vol. 11, no. 12, pp. 386-398, 2024. Crossref, https://doi.org/10.14445/23488379/IJEEE-V11I12P135
Abstract:
This research presents an innovative system architecture for heart disease prediction that integrates Improved Stochastic Gradient Descent (ISGD) with a Decision Tree (DT) classifier. The ISGD-DT model addresses challenges in existing predictive models, such as imbalanced datasets, limited generalizability, and suboptimal accuracy, by leveraging hierarchical layers, graph databases, and decision trees for robust classification outcomes. Validated using benchmark datasets from the UCI Machine Learning Repository, including the Cleveland and Hungarian heart disease datasets, the model demonstrates superior performance with accuracy rates of 93.17%, 88.39%, and 96.29% across different datasets. These results highlight the model's reliability and robustness, making it a valuable tool for improving predictive modeling in healthcare. This research underscores the potential of combining advanced optimization techniques and classification algorithms to enhance the accuracy and applicability of medical prognostics.
Keywords:
Heart illness prediction, Decision Tree, Improved Stochastic Gradient Descent, Deep Learning.
References:
[1] Cardiovascular Diseases, Health Topics, World Health Organization, 2019. [Online]. Available: https://www.who.int/health-topics/cardiovascular-diseases#tab=tab_1
[2] María Teresa García-Ordás et al., “Heart Disease Risk Prediction Using Deep Learning Techniques with Feature Augmentation,” Multimedia Tools and Applications, vol. 82, no. 20, pp. 31759-31773, 2023.
[CrossRef] [Google Scholar] [Publisher Link]
[3] P. Ramprakash et al., “Heart Disease Prediction Using Deep Neural Network,” International Conference on Inventive Computation Technologies (ICICT), Coimbatore, India, pp. 666-670, 2020.
[CrossRef] [Google Scholar] [Publisher Link]
[4] S. Sambath Kumar, and M. Nandhini, “Entropy Slicing Extraction and Transfer Learning Classification for Early Diagnosis of Alzheimer Diseases with sMRI,” ACM Transactions on Multimedia Computing, Communications, and Applications (TOMM), vol. 17, no. 2, pp. 1-22, 2021.
[CrossRef] [Google Scholar] [Publisher Link]
[5] Ivo Sérgio Guimarães Brites et al., “Machine Learning and Iot Applied to Cardiovascular Diseases Identification Through Heart Sounds: A Literature Review,” Informatics, vol. 8, no. 4, pp. 1-24, 2021.
[CrossRef] [Google Scholar] [Publisher Link]
[6] Adedayo Ogunpola et al., “Machine Learning-Based Predictive Models for Detection of Cardiovascular Diseases,” Diagnostics, vol. 14, no. 2, pp. 1-19, 2024.
[CrossRef] [Google Scholar] [Publisher Link]
[7] Pooja Rani et al., “An Extensive Review of Machine Learning and Deep Learning Techniques on Heart Disease Classification and Prediction,” Archives of Computational Methods in Engineering, vol. 31, no. 6, pp. 3331-3349, 2024.
[CrossRef] [Google Scholar] [Publisher Link]
[8] Xiaofeng Yuan, Lin Li, and Yalin Wang, “Nonlinear Dynamic Soft Sensor Modeling with Supervised Long Short-Term Memory Network,” IEEE Transactions on Industrial Informatics, vol. 16, no. 5, pp. 3168-3176, 2020.
[CrossRef] [Google Scholar] [Publisher Link]
[9] Surenthiran Krishnan, Pritheega Magalingam, and Roslina Ibrahim, “Hybrid Deep Learning Model Using Recurrent Neural Network and Gated Recurrent Unit for Heart Disease Prediction,” International Journal of Electrical and Computer Engineering (IJECE), vol. 11, no. 6, pp. 5467-5476, 2021.
[CrossRef] [Google Scholar] [Publisher Link]
[10] Rohit Bharti et al., “Prediction of Heart Disease Using a Combination of Machine Learning and Deep Learning,” Computational Intelligence and Neuroscience, vol. 2021, no. 1, 2021.
[CrossRef] [Google Scholar] [Publisher Link]
[11] Li Yang et al., “Study of Cardiovascular Disease Prediction Model Based on Random Forest in Eastern China,” Scientific Reports, vol. 10, no. 1, pp. 1-8, 2020.
[CrossRef] [Google Scholar] [Publisher Link]
[12] Khaled Mohamad Almustafa, “Prediction of Heart Disease and Classifiers’ Sensitivity Analysis,” BMC Bioinformatics, vol. 21, no. 1, pp. 1-18, 2020.
[CrossRef] [Google Scholar] [Publisher Link]
[13] Shahan Yamin Siddiqui et al., “Modelling, Simulation and Optimization of Diagnosis Cardiovascular Disease Using Computational Intelligence Approaches,” Journal of Medical Imaging and Health Informatics, vol. 10, no. 5, pp. 1005-1022, 2020.
[CrossRef] [Google Scholar] [Publisher Link]
[14] Mert Ozcan, and Serhat Peker, “A Classification and Regression Tree Algorithm for Heart Disease Modeling and Prediction,” Healthcare Analytics, vol. 3, 2023.
[CrossRef] [Google Scholar] [Publisher Link]
[15] Tanmay Kasbe, and Ravi Singh Pippal, “Enhancement in Diagnosis of Coronary Artery Disease Using Fuzzy Expert System,” International Journal of Scientific Research in Computer Science, Engineering and Information Technology (IJSRCSEIT), vol. 3, no. 3, pp. 1324-1331, 2018.
[Google Scholar] [Publisher Link]
[16] Yan Li et al., “Consistency of Variety of Machine Learning and Statistical Models in Predicting Clinical Risks of Individual Patients: Longitudinal Cohort Study Using Cardiovascular Disease as Exemplar,” BMJ, vol. 371, pp. 1-9, 2020.
[CrossRef] [Google Scholar] [Publisher Link]
[17] Emrana Kabir Hashi, and Shahid Uz Zaman, “Developing A Hyperparameter Tuning Based Machine Learning Approach of Heart Disease Prediction,” Journal of Applied Science and Process Engineering, vol. 7, no. 2, pp. 631-647, 2020.
[CrossRef] [Google Scholar] [Publisher Link]
[18] Sibo Prasad Patro, Gouri Sankar Nayak, and Neelamadhab Padhy, “Heart Disease Prediction by Using Novel Optimization Algorithm: A Supervised Learning Prospective,” Informatics in Medicine Unlocked, vol. 26, 2021.
[CrossRef] [Google Scholar] [Publisher Link]
[19] V. Poornima, and D. Gladis, “A Novel Approach for Diagnosing Heart Disease with Hybrid Classifier,” Biomedical Research, vol. 29, no. 11, pp. 2274-2280, 2018.
[CrossRef] [Google Scholar] [Publisher Link]
[20] Kevin Buchan, Michele Filannino, and Özlem Uzuner, “Automatic Prediction of Coronary Artery Disease from Clinical Narratives,” Journal of Biomedical Informatics, vol. 72, pp. 23-32, 2017.
[CrossRef] [Google Scholar] [Publisher Link]
[21] Kartik Budholiya, Shailendra Kumar Shrivastava, and Vivek Sharma, “An Optimized Xgboost Based Diagnostic System for Effective Prediction of Heart Disease,” Journal of King Saud University-Computer and Information Sciences, vol. 34, no. 7, pp. 4514-4523, 2022.
[CrossRef] [Google Scholar] [Publisher Link]
[22] Afef Mdhaffar et al., “CEP4HFP: Complex Event Processing for Heart Failure Prediction,” IEEE transactions on Nanobioscience, vol. 16, no. 8, pp. 708-717, 2017.
[CrossRef] [Google Scholar] [Publisher Link]
[23] Nabaouia Louridi, Samira Douzi, and Bouabid El Ouahidi, “Machine Learning-Based Identification of Patients with A Cardiovascular Defect,” Journal of Big Data, vol. 8, no. 1, pp. 1-15, 2021.
[CrossRef] [Google Scholar] [Publisher Link]
[24] Saba Bashir et al., “MV5: A Clinical Decision Support Framework for Heart Disease Prediction Using Majority Vote Based Classifier Ensemble,” Arabian Journal for Science and Engineering, vol. 39, no. 11, pp. 7771-7783, 2014.
[CrossRef] [Google Scholar] [Publisher Link]
[25] Divya Tomar, and Sonali Agarwal, “Feature Selection Based Least Square Twin Support Vector Machine for Diagnosis of Heart Disease,” International Journal of Bio-Science and Bio-Technology, vol. 6, no. 2, pp. 69-82, 2014.
[CrossRef] [Google Scholar] [Publisher Link]
[26] Ebenezer Obaloluwa Olaniyi, Oyebade Kayode Oyedotun, and Khashman Adnan, “Heart Diseases Diagnosis Using Neural Networks Arbitration,” International Journal of Intelligent Systems and Applications, vol. 7, no. 12, pp. 75-82, 2015. [CrossRef] [Google Scholar] [Publisher Link]
[27] K. Arul Jothi et al., “WITHDRAWN: Heart Disease Prediction System Using Machine Learning,” Materialstoday: Proceedings, 2021.
[CrossRef] [Google Scholar] [Publisher Link]
[28] Gunasekaran Manogaran, and Daphne Lopez, “Health Data Analytics Using Scalable Logistic Regression with Stochastic Gradient Descent,” International Journal of Advanced Intelligence Paradigms (IJAIP), vol. 10, no. (1-2), pp. 118-132, 2018.
[CrossRef] [Google Scholar] [Publisher Link]
[29] N. Deepa et al., “An AI-Based Intelligent System for Healthcare Analysis Using Ridge-Adaline Stochastic Gradient Descent Classifier” The Journal of Supercomputing, vol. 77, no. 2, pp. 1998-2017, 2020.
[CrossRef] [Google Scholar] [Publisher Link]
[30] Muhammad Saqib Nawaz, Bilal Shoaib, and Muhammad Adeel Ashraf, “Intelligent Cardiovascular Disease Prediction Empowered with Gradient Descent Optimization,” Heliyon, vol. 7, no. 5, 2021.
[CrossRef] [Google Scholar] [Publisher Link]
[31] Purushottam, Kanak Saxena, and Richa Sharma, “Efficient Heart Disease Prediction System,” Procedia Computer Science, vol. 85, pp. 962-969, 2016.
[CrossRef] [Google Scholar] [Publisher Link]
[32] Anil Pandurang Jawalkar et al., “Early Prediction of Heart Disease with Data Analysis Using Supervised Learning with Stochastic Gradient Boosting,” Journal of Engineering and Applied Science, vol. 70, no. 1, pp. 1-18, 2023.
[CrossRef] [Google Scholar] [Publisher Link]
[33] Konstantina-Vasiliki Tompra, George Papageorgiou, and Christos Tjortjis, “Strategic Machine Learning Optimization for Cardiovascular Disease Prediction and High-Risk Patient Identification,” Algorithms, vol. 17, no. 5, pp. 1-23, 2024.
[CrossRef] [Google Scholar] [Publisher Link]