An Integrated Variational Decomposition Models for Overlapped Nonstationary Multicomponent Signal Isolation with Incomplete Data
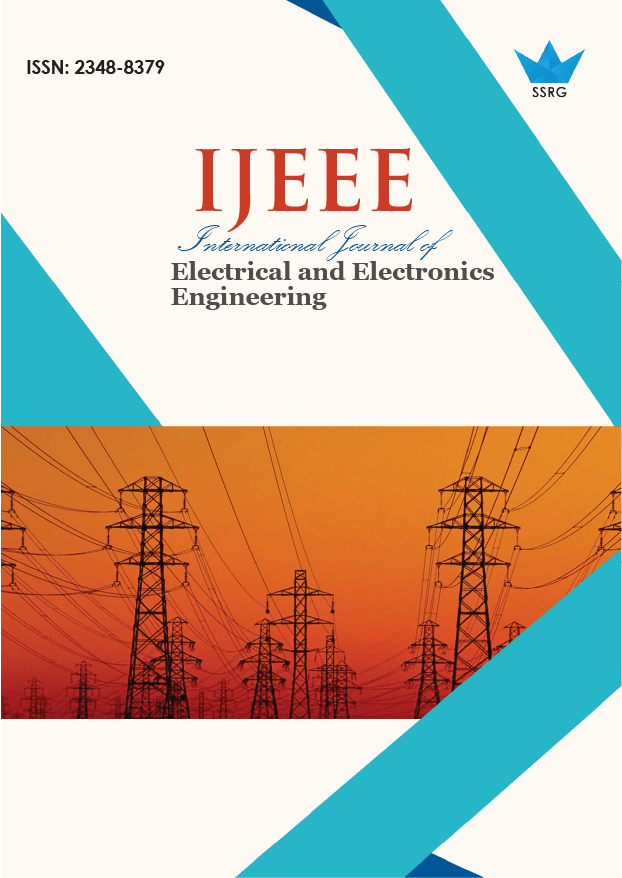
International Journal of Electrical and Electronics Engineering |
© 2024 by SSRG - IJEEE Journal |
Volume 11 Issue 12 |
Year of Publication : 2024 |
Authors : Shaik Mohammed Shareef, M. Venu Gopala Rao |
How to Cite?
Shaik Mohammed Shareef, M. Venu Gopala Rao, "An Integrated Variational Decomposition Models for Overlapped Nonstationary Multicomponent Signal Isolation with Incomplete Data," SSRG International Journal of Electrical and Electronics Engineering, vol. 11, no. 12, pp. 414-426, 2024. Crossref, https://doi.org/10.14445/23488379/IJEEE-V11I12P138
Abstract:
Signal analysis is often challenging due to the complexity of signals, which comprise multiple components that can overlap in frequency and be affected by noise and interference. In many cases, only partial information about these components is available, making signal analysis even more difficult. This paper presents a method to decompose such complex signals using Integrated Variational Decomposition Models. The procedure begins with generating a partially completed multicomponent signal based on radar equations. Then, the signal is transformed into a time-frequency representation using the Short-time Fourier Transform (STFT). Then, a technique called compressive sensing, specifically Rapid Iterative Shrinkage Thresholding Network (RISTN), is utilized to restructure the time-frequency representation by solving a sparse regularization problem. The Instantaneous Frequencies (IF) are subsequently estimated using the Simplified Variational Mode Decomposition (SVMD) algorithm. Finally, the individual components of the overlapped nonstationary multicomponent signal are isolated using an algorithm called Extended Variational Chirp Component Decomposition (EVCCD). The proposed method outperforms existing methods in terms of Root Mean Square Error (RMsE), Mean Squared Error (MSE), Root Mean Absolute Error (RMAE), and computational time, achieving better values such as -15.829 dB, -31.062 dB, 0.034 dB, and 0.90282 respectively.
Keywords:
Compressive sensing, EVCCD, Integrated variational decomposition models, Multicomponent signal, Non-stationary signal, Radar equations, RISTN, STFT, Signal analysis, SVMD, Time-frequency representation, Variational decomposition.
References:
[1] Jing Wu et al., “Micro-Doppler Curve Extraction Based on Reassociation Viterbi Algorithm,” IEEE Transactions on Aerospace and Electronic Systems, vol. 60, no. 4, pp. 4295-4309, 2024.
[CrossRef] [Google Scholar] [Publisher Link]
[2] Huan Wang et al., “TFR Reconstruction from Incomplete m-D Signal via Adaptive Hadamard Product Parametrization,” IEEE Geoscience and Remote Sensing Letters, vol. 20, 2023.
[CrossRef] [Google Scholar] [Publisher Link]
[3] Quentin Legros et al., “Instantaneous Frequency and Amplitude Estimation in Multi-Component Signals Using an EM-based Algorithm,” IEEE Transactions on Signal Processing, vol. 72, pp. 1130-1140, 2024.
[CrossRef] [Google Scholar] [Publisher Link]
[4] Yanbo Mai et al., “ISAR Imaging of Target Exhibiting Micro-Motion Components Based on Joint Constraints of Low Rank and Structured Sparsity,” IEEE Transactions on Antennas and Propagation, vol. 72, no. 5, 2024.
[CrossRef] [Google Scholar] [Publisher Link]
[5] Yipeng Ding et al., “Improved Linear Chirplet Transform and Singular Value Decomposition Joint Algorithm for Motion Target Tracking,” IEEE Internet of Things Journal, vol. 11, no. 8, pp. 13383-13392, 2024.
[CrossRef] [Google Scholar] [Publisher Link]
[6] Yuebin Wang et al., “An RFS Time-Frequency Model for Decomposing Chirp Modes with Dynamic Cross, Appearance and Disappearance,” IEEE Transactions on Aerospace and Electronic Systems, vol. 59, no. 4, pp. 4525-4539, 2023.
[CrossRef] [Google Scholar] [Publisher Link]
[7] Vaishali S. Amin, Yimin D. Zhang, and Braham Himed, “Improved Time-Frequency Representation of Multi-Component FM Signals with Compressed Observations,” 2020 54th Asilomar Conference on Signals, Systems, and Computers, Pacific Grove, CA, USA, pp. 1370-1374, 2020.
[CrossRef] [Google Scholar] [Publisher Link]
[8] Qiyu Tu et al., “Local Maximum Multisynchrosqueezing Transform and its Application,” Digital Signal Processing, vol. 140, 2023.
[CrossRef] [Google Scholar] [Publisher Link]
[9] Xingxing Jiang et al., “Synchronous Chirp Mode Extraction: A Promising Tool for Fault Diagnosis of Rolling Element Bearings under Varying Speed Conditions,” Chinese Journal of Aeronautics, vol. 35, no. 1, pp. 348-364, 2022.
[CrossRef] [Google Scholar] [Publisher Link]
[10] Jie Huang et al., “Adaptive Multivariate Chirp Mode Decomposition,” Mechanical Systems and Signal Processing, vol. 186, 2023.
[CrossRef] [Google Scholar] [Publisher Link]
[11] Qing Li, “Spatio-Temporal Nonconvex Penalty Adaptive Chirp Mode Decomposition for Signal Decomposition of Cross-Frequency Coupled Sources in Seafloor Dynamic Engineering,” Frontiers in Marine Science, vol. 9, 2022.
[CrossRef] [Google Scholar] [Publisher Link]
[12] Jiakai Ding et al., “Slope Synchronous Chirplet Transform and its Application to Tacho-less Order Tracking of Rotating Machineries,” Mechanical Systems and Signal Processing, vol. 196, 2023.
[CrossRef] [Google Scholar] [Publisher Link]
[13] Hongkang Wu et al., “Multivariate Complex Modulation Model Decomposition and its Application to Gear Fault Diagnosis,” Digital Signal Processing, vol. 135, 2023.
[CrossRef] [Google Scholar] [Publisher Link]
[14] Yun Tao Xu, and Zhi Yong Song, “Multi-Component Signal Separation Using CFAR and DBSCAN Based on Ridge Path Regrouping,” Proceedings of the 2023 International Conference on Frontiers of Artificial Intelligence and Machine Learning, pp. 14-18, 2023.
[CrossRef] [Google Scholar] [Publisher Link]
[15] Yi Li, Weijie Xia, and Shiqi Dong, “Time‐Based Multi‐Component Irregular FM Micro‐Doppler Signals Decomposition via STVMD,” IET Radar, Sonar & Navigation, vol. 14, no. 10, pp. 1502-1511, 2020.
[CrossRef] [Google Scholar] [Publisher Link]
[16] Huan Wang et al., “Decomposition for Multi-Component Micro-Doppler Signal with Incomplete Data,” IEEE Geoscience and Remote Sensing Letters, vol. 19, 2022.
[CrossRef] [Google Scholar] [Publisher Link]
[17] Jie Lu et al., “Non-Sinusoidal Micro-Doppler Estimation Based on Dual-Branch Network,” Remote Sensing, vol. 14, no. 19, 2022.
[CrossRef] [Google Scholar] [Publisher Link]
[18] Xin Huang et al., “Multicomponent Collaborative Time-Frequency State-Space Model for Vibration Signal Decomposition under Nonstationary Conditions,” Measurement Science and Technology, vol. 35, no. 6, 2024.
[CrossRef] [Google Scholar] [Publisher Link]
[19] Jamal Akram et al., “Multi-Component Instantaneous Frequency Estimation Using Signal Decomposition and Time-Frequency Filtering,” Signal, Image and Video Processing, vol. 14, no. 8, pp. 1663-1670, 2020.
[CrossRef] [Google Scholar] [Publisher Link]
[20] Lu Yan et al., “Mechanical Varying Non-Stationary Signal Separation Method Based on Instantaneous Frequency Estimation,” Advances in Mechanical Engineering, vol. 15, no. 5, 2023.
[CrossRef] [Google Scholar] [Publisher Link]
[21] Lei Tang et al., “An Improved Local Maximum Synchrosqueezing Transform with Adaptive Window Width for Instantaneous Frequency Identification of Time-Varying Structures,” Engineering Structures, vol. 292, 2023.
[CrossRef] [Google Scholar] [Publisher Link]