Voltage Over Scaling Based GDI Approximate Adder
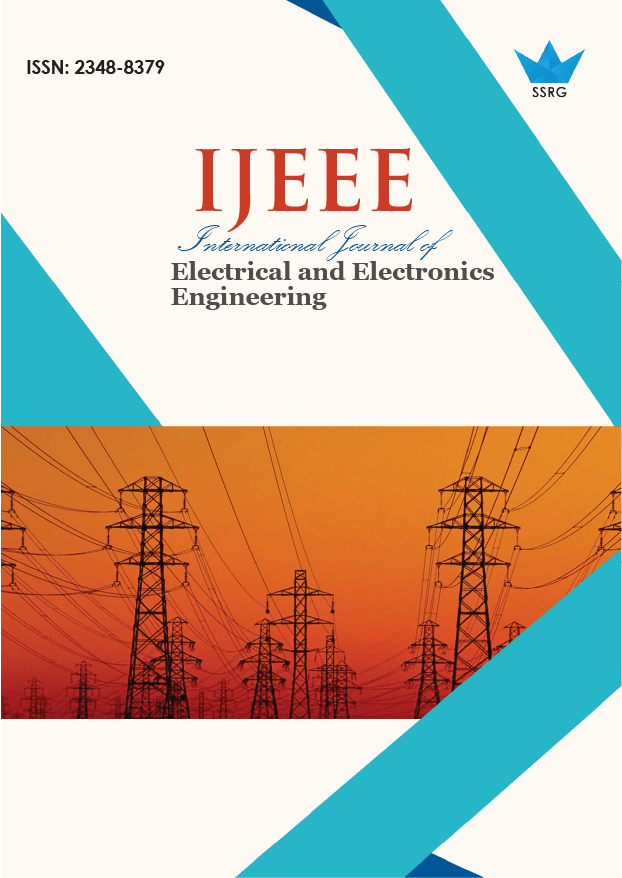
International Journal of Electrical and Electronics Engineering |
© 2025 by SSRG - IJEEE Journal |
Volume 12 Issue 1 |
Year of Publication : 2025 |
Authors : G.R. Mahendra Babu, K.P. Sridhar |
How to Cite?
G.R. Mahendra Babu, K.P. Sridhar, "Voltage Over Scaling Based GDI Approximate Adder," SSRG International Journal of Electrical and Electronics Engineering, vol. 12, no. 1, pp. 47-62, 2025. Crossref, https://doi.org/10.14445/23488379/IJEEE-V12I1P106
Abstract:
Machine Learning (ML) models are deployed in resource-constrained edge and fog devices in the Internet of Things (IoT) paradigm. The ML models are trained with Convolutional Neural Networks (CNN) which directly influence the device performances like power, speed, and network bandwidth. In such cases, efficient hardware devices are needed to implement the ML models. This work aims to identify performance-efficient techniques and adapt them to the proposed design. This paper proposes Voltage Over Scaling (VOS) based Gate Diffusion Input (GDI) Approximate Full Adder (AFA) identified as VOS-GAFA at the transistor level. The proposed VOS-GAFA is used to develop the 8-bit VOS-based Ripple Carry Adder (RCA) identified as VOS-RCA. The experimental results of the proposed VOS-GAFA have shown 56.11 % and 44 % reduction of power consumption and transistor count, respectively, when compared to the best existing approximate full adder. Moreover, VOS-GAFA has a high Noise Margin (NM) value that provides proper circuit operation and robustness against noise sources. VOS-RCA showed improved power, PDP, and delay values when compared to existing 8-bit RCA, and hence, it is appropriate for resource-constrained edge and fog IoT devices. This research work is carried out using the cadence virtuoso tool at gpdk45nm technology.
Keywords:
Approximate computing, Gate diffusion input, Low power full adder, Machine Learning, Voltage over scaling.
References:
[1] Ericsson, Ericsson Mobility Reports, 2024. [Online]. Available: https://www.ericsson.com/en/reports-and-papers/mobility-report
[2] Hao Feng et al., “Collaborative Data Caching and Computation Offloading for Multi-Service Mobile Edge Computing,” IEEE Transaction on Vehiculars Technology, vol. 70, no. 9, pp. 9408-9422, 2021.
[CrossRef] [Google Scholar] [Publisher Link]
[3] Georgios Karakonstantis, and Charles J. Gillan, Computing at the EDGE: New Challenges for Service Provision, 1st ed., Springer Nature, 2022.
[CrossRef] [Google Scholar] [Publisher Link]
[4] Niko Mäkitalo et al., “Action-Oriented Programming Model: Collective Executions and Interactions in the Fog,” Journal of Systems and Software, vol. 157, 2019.
[CrossRef] [Google Scholar] [Publisher Link]
[5] Hans Jakob Damsgaard et al., “Adaptive Approximate Computing in Edge AI and IoT Applications: A Review,” Journal of Systems Architecture, vol. 150, 2024.
[CrossRef] [Google Scholar] [Publisher Link]
[6] Lucas Martin Wisniewski et al., “Hardware Solutions for Low-Power Smart Edge Computing,” Journal of Low Power Electronics and Applications, vol. 12, no. 4, 2022.
[CrossRef] [Google Scholar] [Publisher Link]
[7] Jorge Castro-Godínez et al., “Approximate Acceleration for CNN-Based Applications on IoT Edge Devices,” 2020 IEEE 11th Latin American Symposiums on Circuit & System (LASCAS), San Jose, Costa Rica, pp. 1-4, 2020.
[CrossRef] [Google Scholar] [Publisher Link]
[8] Jie Tang et al., “LoPECS: A Low-Power Edge Computing System for Real-Time Autonomous Driving Services,” IEEE Access, vol. 8, pp. 30467-30479, 2020.
[CrossRef] [Google Scholar] [Publisher Link]
[9] Nabiollah Shiri, Ayoub Sadeghi, and Mahmood Rafiee, “High-Efficient and Error-Resilient Gate Diffusion Input-Based Approximate Full Adders for Complex Multistage Rapid Structures,” Computers and Electrical Engineering, vol. 109, 2023.
[CrossRef] [Google Scholar] [Publisher Link]
[10] Venu Birudu et al., “Computing In-Memory Reconfigurable (Accurate/Approximate) Adder Design with Negative Capacitance FET 6T-SRAM for Energy Efficient AI Edge Devices,” Semiconductor Science and Technology, vol. 39, no. 5, 2024.
[CrossRef] [Google Scholar] [Publisher Link]
[11] Forouzan Bahrami, Nabiollah Shiri, and Farshad Pesaran, “Imprecise Subtractor Using a New Efficient Approximate-Based Gate Diffusion Input Full Adder for Bioimages Processing,” Computers and Electrical Engineering, vol. 108, 2023.
[CrossRef] [Google Scholar] [Publisher Link]
[12] Ayoub Sadeghi et al., “High Efficient GDI-CNTFET-Based Approximate Full Adder for Next Generation of Computer Architectures,” IEEE Embedded Systems Letters, vol. 15, no. 1, pp. 33-36, 2023.
[CrossRef] [Google Scholar] [Publisher Link]
[13] Xuemei Fan et al., “A Timing-Aware Configurable Adder Based on Timing Detection for Low-Voltage Computing,” IEEE Journal on Emerging and Selected Topics in Circuits and Systems, vol. 13, no. 1, pp. 237-248, 2023.
[CrossRef] [Google Scholar] [Publisher Link]
[14] Manikandan Nagarajan et al., “Power and Area Efficient Cascaded Effectless GDI Approximate Adder for Accelerating Multimedia Applications Using Deep Learning Model,” Computational Intelligence and Neuroscience, vol. 2022, no. 1, 2022.
[CrossRef] [Google Scholar] [Publisher Link]
[15] Elahe Baratalipour, and Arezoo Kamran, “SAMA: Self-Adjusting Multi-Cycle Approximate Adder,” Microelectronics Journal, vol. 134, 2023.
[CrossRef] [Google Scholar] [Publisher Link]
[16] Anubothula Tirupathireddy, Musala Sarada, and Avireni Srinivasulu, “Energy-Efficient Approximate Adders for DSP Applications,” Analog Integrated Circuits and Signal Processing, vol. 107, pp. 649-657, 2021.
[CrossRef] [Google Scholar] [Publisher Link]
[17] Padmanabhan Balasubramanian, Raunaq Nayar, and Douglas L. Maskell, “Gate-Level Static Approximate Adders: A Comparative Analysis,” Electronics, vol. 10, no. 23, 2021.
[CrossRef] [Google Scholar] [Publisher Link]
[18] A. Morgenstein, A. Fish, and I.A. Waggner, “Gate-Diffusion Input (GDI): A Power-Efficient Method for Digital Combinatorial Circuits,” IEEE Transactions on Very Large Scale Integration (VLSI) Systems, vol. 10, no. 5, pp. 566-581, 2002.
[CrossRef] [Google Scholar] [Publisher Link]
[19] Arkadiy Morgenshtein, Idan Shwartz, and Alexander Fish, “Gate Diffusion Input (GDI) Logic in Standard CMOS Nanoscale Process,” 26th Convention of Electrical and Electronics Engineers in Israel, Eilat, Israel, pp. 776-780, 2010.
[CrossRef] [Google Scholar] [Publisher Link]
[20] Mohan Shoba, and Rangaswamy Nakkeeran, “GDI Based Full Adders for Energy Efficient Arithmetic Applications,” Engineering Science and Technology, an International Journal, vol. 19, no. 1, pp. 485-496, 2016.
[CrossRef] [Google Scholar] [Publisher Link]
[21] S. Radhakrishnan, T. Nirmalraj, and Rakesh Kumar Karn, “An Enhanced Gate Diffusion Input Technique for Low Power Applications,” Microelectronics Journal, vol. 93, 2019.
[CrossRef] [Google Scholar] [Publisher Link]
[22] Ebrahim Abiri, Abdolreza Darabi, and Ayoub Sadeghi, “Gate-Diffusion Input (GDI) Method for Designing Energy-Efficient Circuits in Analogue Voltage-Mode Fuzzy and QCA Systems,” Microelectronics Journal, vol. 87, pp. 81-100, 2019.
[CrossRef] [Google Scholar] [Publisher Link]
[23] Jebashini Ponnian et al., “A New Systematic GDI Circuit Synthesis Using MUX Based Decomposition Algorithm and Binary Decision Diagram for Low Power ASIC Circuit Design,” Microelectronics Journal, vol. 108, 2021.
[CrossRef] [Google Scholar] [Publisher Link]
[24] Seyedeh Fatemeh Deymad, Nabiollah Shiri, and Farshad Pesaran, “High-Efficient Reversible Full Adder Realized by Dynamic Threshold-Based Gate Diffusion Input Logics,” Microelectronics Journal, vol. 142, 2023.
[CrossRef] [Google Scholar] [Publisher Link]
[25] B. Ravi Chandra et al., “Implementations of Ripples Carry Adders Using Full Swing Gates Diffusion Inputs,” 7th International Conference on Trends in Electronics and Informatics (ICOEI), Tirunelveli, India, pp. 44-50, 2023.
[CrossRef] [Google Scholar] [Publisher Link]
[26] Debabrata Mohapatra, Georgios Karakonstantis, and Kaushik Roy, “Significance Driven Computation: A Voltage-Scalable, Variation-Aware, Quality-Tuning Motion Estimator,” Proceeding of the 2009 ACM/IEEE International Symposium on Low Powers Electronics and Design, pp. 195-200, 2009.
[CrossRef] [Google Scholar] [Publisher Link]
[27] Rengarajan Ragavan et al., “Pushing the Limits of Voltage Over-Scaling for Error-Resilient Applications,” Design, Automation & Test in Europe Conference & Exhibitions (DATE), Lausanne, Switzerland, pp. 476-481, 2017.
[CrossRef] [Google Scholar] [Publisher Link]
[28] Junqi Huang, T. Nandha Kumar, and Haider Abbas, “A Promising Power-Saving Technique: Approximate Computing,” 2018 IEEE Symposium on Computer Applications & Industrial Electronics (ISCAIE), Penang, Malaysia, pp. 285-290, 2018.
[CrossRef] [Google Scholar] [Publisher Link]
[29] Junqi Huang, T. Nandha Kumar, and Haider Abbas, “Simulation-Based Evaluation of Approximate Adders for Image Processing Using Voltage Overscaling Method,” 5th International Conference on Signal and Image Processing (ICSIP), Nanjing, China, pp. 499-505, 2020.
[CrossRef] [Google Scholar] [Publisher Link]
[30] Chandra Shaker Pittala, Vallabhuni Vijay, and B. Naresh Kumar Reddy, “1-Bit FinFET Carry Cells for Low Voltage High-Speed Digital Signal Processing Applications,” Silicon, vol. 15, no. 2, pp. 713-724, 2023.
[CrossRef] [Google Scholar] [Publisher Link]
[31] Vinay K. Chippa et al., “Analysis and Characterization of Inherent Application Resilience for Approximate Computing,” 50th ACM/EDAC/IEEE Design Automation Conference (DAC), Austin, TX, USA, pp. 1-9, 2013.
[CrossRef] [Google Scholar] [Publisher Link]
[32] Ronald G. Dreslinski et al., “Reconfigurable Energy Efficient Near Threshold Cache Architectures,” 41st IEEE/ACM International Symposium on Microarchitectures, Como, Italy, pp. 459-470, 2008.
[CrossRef] [Google Scholar] [Publisher Link]