Sensor and Computer Vision Based Cattle Health Monitoring and Management
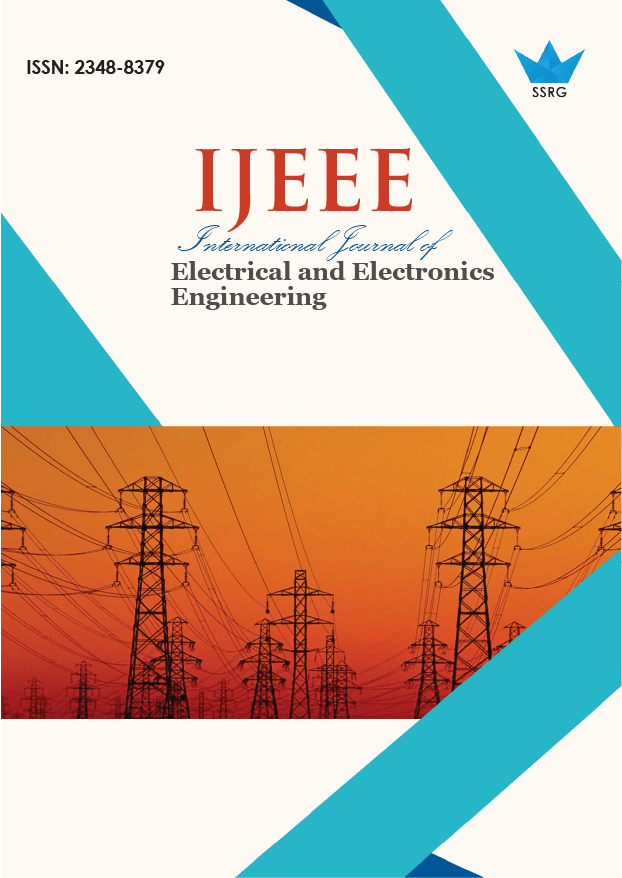
International Journal of Electrical and Electronics Engineering |
© 2025 by SSRG - IJEEE Journal |
Volume 12 Issue 1 |
Year of Publication : 2025 |
Authors : Devendra Singh, Rajesh Singh, Anita Gehlot, Gaurav Bhandari, Atulya Verma, Pavan Gangwar, Purnendu Shekhar Pandey |
How to Cite?
Devendra Singh, Rajesh Singh, Anita Gehlot, Gaurav Bhandari, Atulya Verma, Pavan Gangwar, Purnendu Shekhar Pandey, "Sensor and Computer Vision Based Cattle Health Monitoring and Management," SSRG International Journal of Electrical and Electronics Engineering, vol. 12, no. 1, pp. 94-103, 2025. Crossref, https://doi.org/10.14445/23488379/IJEEE-V12I1P109
Abstract:
As part of its efforts to achieve the Sustainable Development Goals by 2030, the United Nations promotes sustainable farming. Though there are complications with real-world deployment, autonomous farming is being explored within the context of edge computing. Automation and smart farming practices could boost farmer efficiency, sustainability, and the well-being of livestock. These advancements minimize costs, eliminate laborious processes, and elevate product quality. Wearable sensors gauge animal behavior, emphasizing the significance of impeccable remote data sharing in this expanding business. Global population growth is driving an evolution toward intelligent farming to address food security and resource constraints. IoT and data analytics optimize farming productivity by substituting outdated wireless sensor networks. IoT effortlessly incorporates technologies like WSN, RFID, and cloud computing. ZigBee technology finds application in livestock health monitoring systems, where sensors measuring heart rate, temperature, pulse rate, and respiration are included. These sensors have connectivity to a Graphical User Interface (GUI) to improve livestock wellness tracking. The advantages of cloud computing encompass exceptionally low latency, bandwidth optimization, assurance, and real-time analytics. This article examines Computer Vision and sensor-based technologies in intelligent agriculture.
Keywords:
Sustainable development, Intelligent agribusiness, Livestock management, Wearable sensors, Computer Vision.
References:
[1] Mohamed Gameil, and Tarek Gaber, “Wireless Sensor Networks-Based Solutions for Cattle Health Monitoring: A Survey,” Proceedings of the International Conference on Advanced Intelligent Systems and Informatics, pp. 779-788, 2019.
[CrossRef] [Google Scholar] [Publisher Link]
[2] M.J. O'Grady, D. Langton, and G.M.P. O'Hare, “Edge Computing: A Tractable Model for Smart Agriculture?,” Artificial Intelligence in Agriculture, vol. 3, pp. 42-51, 2019.
[CrossRef] [Google Scholar] [Publisher Link]
[3] K.P. Suresh et al., “Livestock Disease Risk Forewarning Methodology-March 2023,” ICAR-NIVEDI, vol. 11, no. 2, pp. 1-51, 2023.
[Google Scholar] [Publisher Link]
[4] Sasmita Padhy et al., “AgriSecure: A Fog Computing-Based Security Framework for Agriculture 4.0 via Blockchain,” Processes, vol. 11, no. 3, 2023.
[CrossRef] [Google Scholar] [Publisher Link]
[5] Wataru Iwasaki, Nobutomo Morita, and Maria Portia Briones Nagata, “IoT Sensors for Smart Livestock Management,” Chemical, Gas, and Biosensors for Internet of Things and Related Applications, pp. 207-221, 2019.
[CrossRef] [Google Scholar] [Publisher Link]
[6] Syed Anas Ansar et al., “A Step towards Smart Farming: Unified Role of AI and IoT,” Computer Vision and Robotics, pp. 557-578, 2023.
[CrossRef] [Google Scholar] [Publisher Link]
[7] Fatih Başçiftçi, and Kamil Aykutalp Gündüz, “Identification of Acidosis Disease in Cattle Using IoT,” 2019 4th International Conference on Computer Science and Engineering (UBMK), Samsun, Turkey, pp. 58-62, 2019.
[CrossRef] [Google Scholar] [Publisher Link]
[8] Navaneethan Renuga Devi et al., “Animal Health Monitoring Using Nanosensor Networks,” Nanosensors for Smart Agriculture, pp. 573-608, 2022.
[CrossRef] [Google Scholar] [Publisher Link]
[9] Hirofumi Nogami et al., “Minimized Bolus-Type Wireless Sensor Node with a Built-in Three-Axis Acceleration Meter for Monitoring a Cow’s Rumen Conditions,” Sensors, vol. 17, no. 4, 2017.
[CrossRef] [Google Scholar] [Publisher Link]
[10] Deepika Sharma, Jayati Mishra, and Nibedita Talukdar, “Application of Precision Livestock Farming for Improved Goat Management and Production,” Revista Electronica de Veterinaria, vol. 24, no. 2, pp. 461-470, 2023.
[Google Scholar] [Publisher Link]
[11] Mohamed Zeineldin et al., “On-Farm Point-of-Care Diagnostic Technologies for Monitoring Health, Welfare, and Performance in Livestock Production Systems,” Sustainable Agriculture Reviews 54, pp. 209-232, 2021.
[CrossRef] [Google Scholar] [Publisher Link]
[12] Kennedy Okokpujie et al., “Development of a Sustainable Internet of Things-Based System for Monitoring Cattle Health and Location with Web and Mobile Application Feedback,” Mathematical Modelling of Engineering Problems, vol. 10, no. 3, pp. 740-748, 2023.
[CrossRef] [Google Scholar] [Publisher Link]
[13] Shyamasree Ghosh, and Rathi Dasgupta, Machine Learning in Biological Sciences: Updates and Future Prospects, 1st ed., Springer Singapore, 2022.
[CrossRef] [Google Scholar] [Publisher Link]
[14] Amsale Zelalem Bayih et al., “Utilization of Internet of Things and Wireless Sensor Networks for Sustainable Smallholder Agriculture,” Sensors, vol. 22, no. 9, 2022.
[CrossRef] [Google Scholar] [Publisher Link]
[15] Anuj Tewari et al., “Biosensors: Modern Tools for Disease Diagnosis and Animal Health Monitoring,” Biosensors in Agriculture: Recent Trends and Future Perspectives, pp. 387-414, 2021.
[CrossRef] [Google Scholar] [Publisher Link]
[16] Brahim Achour et al., “Classification of Dairy Cows’ Behavior by Energy-Efficient Sensor,” Journal of Reliable Intelligent Environments, vol. 8, no. 2, pp. 165-182, 2022.
[CrossRef] [Google Scholar] [Publisher Link]
[17] Mohammad Meraj et al., “A Critical Review of Detection and Prediction of Infectious Disease Using IoT Sensors,” 2021 Second International Conference on Electronics and Sustainable Communication Systems (ICESC), Coimbatore, India, pp. 679-684, 2021.
[CrossRef] [Google Scholar] [Publisher Link]
[18] Mohit Taneja et al., “Connected Cows: Utilizing Fog and Cloud Analytics toward Data-Driven Decisions for Smart Dairy Farming,” IEEE Internet of Things Magazine, vol. 2, no. 4, pp. 32-37, 2019.
[CrossRef] [Google Scholar] [Publisher Link]
[19] Chaowei Jia, and Fei Dong, “Research on Intelligent Collar Animal Husbandry Health Diagnosis Service Platform Based on Cloud Computing,” 2022 World Automation Congress (WAC), San Antonio, TX, USA, pp. 489-493, 2022.
[CrossRef] [Google Scholar] [Publisher Link]
[20] Beibei Xu et al., “Evaluation of Deep Learning for Automatic Multi-View Face Detection in Cattle,” Agriculture, vol. 11, no. 11, 2021.
[CrossRef] [Google Scholar] [Publisher Link]
[21] Sargam Yadav et al., “Disruptive Technologies in Smart Farming: An Expanded View with Sentiment Analysis,” Agri Engineering, vol. 4, no. 2, pp. 424-460, 2022.
[CrossRef] [Google Scholar] [Publisher Link]
[22] Yogeswaranathan Kalyani, and Rem Collier, “A Systematic Survey on the Role of Cloud, Fog, and Edge Computing Combination in Smart Agriculture,” Sensors, vol. 21, no. 17, 2021.
[CrossRef] [Google Scholar] [Publisher Link]
[23] Vu Khanh Quy et al., “IoT-Enabled Smart Agriculture: Architecture, Applications, and Challenges,” Applied Sciences, vol. 12, no. 7, 2022.
[CrossRef] [Google Scholar] [Publisher Link]
[24] Serap Göncü, and Nazan Koluman, “The Sensor Technologies for More Efficient Cow Reproduction Systems,” MOJ Ecology & Environmental Sciences, vol. 4, no. 3, pp. 128-131, 2019.
[CrossRef] [Google Scholar] [Publisher Link]
[25] Shesh Asopa, and Ashish Joshi, “Biosensors: Advanced Tools for Disease Diagnosis and Animal Health Monitoring,” Current Advances in Agriculture, Animal Husbandry and Allied Sciences (CAAAAS), pp. 45-49, 2023.
[Google Scholar]
[26] Karina Džermeikaitė, Dovilė Bačėninaitė, and Ramūnas Antanaitis, “Innovations in Cattle Farming: Application of Innovative Technologies and Sensors in the Diagnosis of Diseases,” Animals, vol. 13, no. 5, 2023.
[CrossRef] [Google Scholar] [Publisher Link]
[27] Sumaira Younis et al., “Nanosensors for Animal Health Monitoring,” Nanosensors for Smart Agriculture, pp. 509-529, 2022.
[CrossRef] [Google Scholar] [Publisher Link]
[28] Ephraim Maltz, “Individual Dairy Cow Management: Achievements, Obstacles and Prospects,” Journal of Dairy Research, vol. 87, no. 2, pp. 145-157, 2020.
[CrossRef] [Google Scholar] [Publisher Link]
[29] P. Keertana, B. Vanathi, and K. Shanmugam, “A Survey on Various Animal Health Monitoring and Tracking Techniques,” International Research Journal of Engineering and Technology, vol. 4, no. 2, pp. 533-536, 2017.
[Google Scholar] [Publisher Link]
[30] AftabAhmed Isak Mulla et al., “Continuous Health Surveillance System for Cattle,” 2017 International Conference on Intelligent Computing and Control Systems (ICICCS), Madurai, India, pp. 1192-1195, 2017.
[CrossRef] [Google Scholar] [Publisher Link]
[31] Olakunle Elijah et al., “An Overview of Internet of Things (IoT) and Data Analytics in Agriculture: Benefits and Challenges,” IEEE Internet of Things Journal, vol. 5, no. 5, pp. 3758-3773, 2018.
[CrossRef] [Google Scholar] [Publisher Link]
[32] Mohd Javaid et al., “Understanding the Potential Applications of Artificial Intelligence in Agriculture Sector,” Advanced Agrochem, vol. 2, no. 1, pp. 15-30, 2023.
[CrossRef] [Google Scholar] [Publisher Link]
[33] Sai Ma et al., “Development of Noncontact Body Temperature Monitoring and Prediction System for Livestock Cattle,” IEEE Sensors Journal, vol. 21, no. 7, pp. 9367-9376, 2021.
[CrossRef] [Google Scholar] [Publisher Link]
[34] Manisha Malhotra et al., “Application of AI/ML Approaches for Livestock Improvement and Management,” Biotechnological Interventions Augmenting Livestock Health and Production, pp. 377-394, 2023.
[CrossRef] [Google Scholar] [Publisher Link]
[35] Andrea Puig et al., “Technological Tools for the Early Detection of Bovine Respiratory Disease in Farms,” Animals, vol. 12, no. 19, 2022.
[CrossRef] [Google Scholar] [Publisher Link]
[36] Inés Sittón-Candanedo et al., “A Review of Edge Computing Reference Architectures and a New Global Edge Proposal,” Future Generation Computer Systems, vol. 99, pp. 278-294, 2019.
[CrossRef] [Google Scholar] [Publisher Link]
[37] Amira Bourechak et al., “At the Confluence of Artificial Intelligence and Edge Computing in IoT-Based Applications: A Review and New Perspectives,” Sensors, vol. 23, no. 3, 2023.
[CrossRef] [Google Scholar] [Publisher Link]
[38] Innocent Kutyauripo, Munyaradzi Rushambwa, and Lyndah Chiwazi, “Artificial Intelligence Applications in the Agrifood Sectors,” Journal of Agriculture and Food Research, vol. 11, 2023.
[CrossRef] [Google Scholar] [Publisher Link]
[39] Mohit Taneja et al., “Machine Learning Based Fog Computing Assisted Data-Driven Approach for Early Lameness Detection in Dairy Cattle,” Computers and Electronics in Agriculture, vol. 171, 2020.
[CrossRef] [Google Scholar] [Publisher Link]
[40] Khouloud Hwerbi et al., “Veterinary Drone: Blockchain-Based System for Cattle Health Monitoring,” 2023 International Wireless Communications and Mobile Computing (IWCMC), Marrakesh, Morocco, pp. 1613-1618, 2023.
[CrossRef] [Google Scholar] [Publisher Link]
[41] Safa Ayadi et al., “Dairy Cow Rumination Detection: A Deep Learning Approach,” Distributed Computing for Emerging Smart Networks, pp. 123-139, 2021.
[CrossRef] [Google Scholar] [Publisher Link]