Data-Driven Approach Using Random Forest Regression for Accurate Electric Vehicle Battery State of Health Estimation
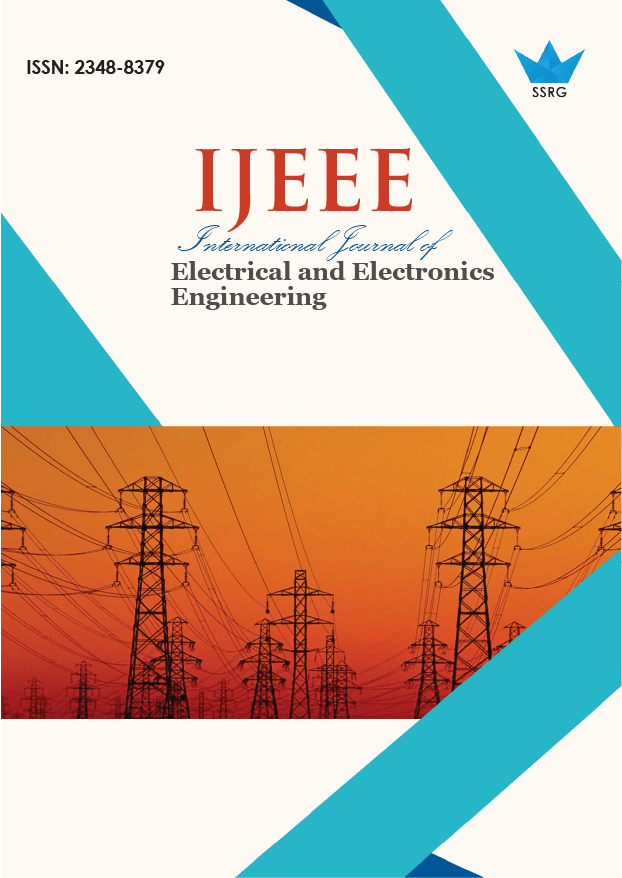
International Journal of Electrical and Electronics Engineering |
© 2025 by SSRG - IJEEE Journal |
Volume 12 Issue 1 |
Year of Publication : 2025 |
Authors : K. Anitha, Jerzy R. Szymański, Marta Zurek-Mortka, Mithileysh Sathiyanarayanan |
How to Cite?
K. Anitha, Jerzy R. Szymański, Marta Zurek-Mortka, Mithileysh Sathiyanarayanan, "Data-Driven Approach Using Random Forest Regression for Accurate Electric Vehicle Battery State of Health Estimation," SSRG International Journal of Electrical and Electronics Engineering, vol. 12, no. 1, pp. 104-113, 2025. Crossref, https://doi.org/10.14445/23488379/IJEEE-V12I1P110
Abstract:
The State of Health (SoH) needs to be accurately estimated for Electric Vehicle (EV) batteries to manage performance, safety and longevity. This study aims to propose a data-driven method with the Random Forest Regression (RFR) model to accurately predict the SoH. This approach builds on historical battery performance data to train the RFR model, which is particularly useful for capturing complex nonlinear relationships between input features and the SoH metric. Whereas model-based methods require electrochemical models, and data-driven methods often rely on extensive laboratory testing, our method demonstrates a pathway to a computationally efficient, flexible, and accurate approach that works across a diversity of battery types and use cases. It used key features like voltage, current, temperature, and charge/discharge rates as predictors, which allows a comprehensive examination of the current and former battery behaviours. This model has been evaluated against various benchmark datasets and has shown a high level of accuracy and robustness.
Keywords:
Battery Management System, Open Circuit Voltage, Random forest regression, Feature selection, Bias-variance.
References:
[1] Abbas Fotouhi et al., “A Review on Electric Vehicle Battery Modelling: From Lithium-ion toward Lithium-Sulphur,” Renewable and Sustainable Energy Reviews, vol. 56, pp. 1008-1021, 2016.
[CrossRef] [Google Scholar] [Publisher Link]
[2] Rui Xiong, Linlin Li, and Jinpeng Tian, “Towards a Smarter Battery Management System: A Critical Review on Battery State of Health Monitoring Methods,” Journal of Power Sources, vol. 405, pp. 18-29, 2018.
[CrossRef] [Google Scholar] [Publisher Link]
[3] Rui Xiong et al., “Critical Review on the Battery State of Charge Estimation Methods for Electric Vehicles,” IEEE Access, vol. 6, pp. 1832-1843, 2017.
[CrossRef] [Google Scholar] [Publisher Link]
[4] Prashant Shrivastava et al., “Review on Technological Advancement of Lithium-Ion Battery States Estimation Methods for Electric Vehicle Applications,” Journal of Energy Storage, vol. 64, 2023.
[CrossRef] [Google Scholar] [Publisher Link]
[5] Nassim Noura, Loïc Boulon, and Samir Jemeï, “A Review of Battery State of Health Estimation Methods: Hybrid Electric Vehicle Challenges,” World Electric Vehicle Journal, vol. 11, no. 4, 2020.
[CrossRef] [Google Scholar] [Publisher Link]
[6] Dae Kyo Seo et al., “Generation of Radiometric, Phenological Normalized Image Based on Random Forest Regression for Change Detection,” Remote Sensing, vol. 9, no. 11, 2017.
[CrossRef] [Google Scholar] [Publisher Link]
[7] Sung-Wook Hwang et al., “Feature Importance Measures from Random Forest Regression Using Near-Infrared Spectra for Predicting Carbonization Characteristics of Kraft Lignin-Derived Hydrochar,” Journal of Wood Science, vol. 69, 2023.
[CrossRef] [Google Scholar] [Publisher Link]
[8] Daniel Doz, Mara Cotič, and Darjo Felda, “Random Forest Regression in Predicting Students’ Achievements and Fuzzy Grades,” Mathematics, vol. 11, no. 19, 2023.
[CrossRef] [Google Scholar] [Publisher Link]
[9] Kei Long Wong et al., “Identifying Degradation Indicators for Electric Vehicle Battery Based on Field Testing Data,” 2022 IEEE Electrical Power and Energy Conference (EPEC), Victoria, BC, Canada, pp. 206-211, 2022.
[CrossRef] [Google Scholar] [Publisher Link]
[10] Murukuri S.V.S.V. Vasanth et al., “DELiB: Deep Extreme Learning-Based Health Estimation for Lithium-ion Battery,” 2023 IEEE International Instrumentation and Measurement Technology Conference (I2MTC), Kuala Lumpur, Malaysia, pp. 1-6, 2023.
[CrossRef] [Google Scholar] [Publisher Link]
[11] Xu Li et al., “State of Health Estimation and Prediction of Electric Vehicle Power Battery Based on Operational Vehicle Data,” Journal of Energy Storage, vol. 72, 2023.
[CrossRef] [Google Scholar] [Publisher Link]
[12] Huzaifa Rauf, Muhammad Khalid, and Naveed Arshad, “Machine Learning in State of Health and Remaining Useful Life Estimation: Theoretical and Technological Development in Battery Degradation Modelling,” Renewable & Sustainable Energy Reviews, vol. 156, 2022.
[CrossRef] [Google Scholar] [Publisher Link]
[13] Zhengyi Bao et al., “Deep-Learning Network-Based Method for SOH Estimation of Lithium-Ion Battery for Electric Vehicles,” The Proceedings of the 5th International Conference on Energy Storage and Intelligent Vehicles (ICEIV 2022), pp. 588-597, 2023.
[CrossRef] [Google Scholar] [Publisher Link]
[14] Edoardo Lelli et al., “On-Road Experimental Campaign for Machine Learning Based State of Health Estimation of High-Voltage Batteries in Electric Vehicles,” Energies, vol. 16, no. 12, 2023.
[CrossRef] [Google Scholar] [Publisher Link]
[15] Taysa Millena Banik Marques et al., “An Overview of Methods and Technologies for Estimating Battery State of Charge in Electric Vehicles,” Energies, vol. 16, no. 13, 2023.
[CrossRef] [Google Scholar] [Publisher Link]