Performance Analysis of Neural Networks for Fault Detection in Induction Motor
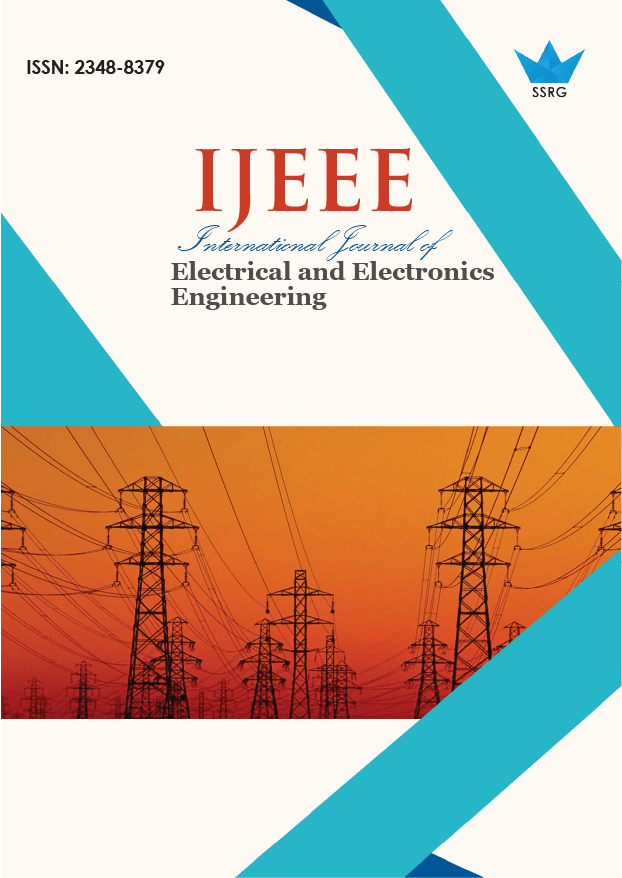
International Journal of Electrical and Electronics Engineering |
© 2025 by SSRG - IJEEE Journal |
Volume 12 Issue 1 |
Year of Publication : 2025 |
Authors : N. Sivaraj, B. Rajagopal |
How to Cite?
N. Sivaraj, B. Rajagopal, "Performance Analysis of Neural Networks for Fault Detection in Induction Motor," SSRG International Journal of Electrical and Electronics Engineering, vol. 12, no. 1, pp. 129-141, 2025. Crossref, https://doi.org/10.14445/23488379/IJEEE-V12I1P112
Abstract:
Condition monitoring and fault identification are essential for preventing damage in industrial machinery, particularly in three-phase squirrel cage Induction Motors (IMs), which are widely used due to their reliability and robust design. This paper compares three advanced techniques for diagnosing Motor faults, including issues like inter-turn faults in the stator, bearing malfunctions and faults from the rotor by analyzing motor current and speed: 1-D Convolutional Neural Networks (1-D CNN), Grey Wolf Optimized Probabilistic Neural Networks (GWOPNN), and Whale Optimized Pattern Recognition Neural Networks (WOAPRNN). The study evaluates each method’s ability to detect and classify faults. Results show that the whale-optimized pattern classification neural network achieves the highest accuracy of 99.15%, making it the most effective method for fault detection. Each technique -offers unique strengths in fault classification and detection, with the goal of enhancing motor reliability and efficiency in industrial environments. By improving fault diagnosis, these methods contribute to reducing downtime, lowering maintenance costs, and increasing the operational lifespan of induction motors.
Keywords:
1-D CNN, Bearing faults, Fault diagnosis, GWOPNN, Induction motors, Machine learning, Rotor faults, Stator Interturn faults, WOAPRNN.
References:
[1] Dhiraj Neupane, and Jongwon Seok, “Bearing Fault Detection and Diagnosis Using Case Western Reserve University Dataset with Deep Learning Approaches: A Review,” IEEE Access, vol. 8, pp. 93155-93178, 2020.
[CrossRef] [Google scholar] [Publisher Link]
[2] Paulo Antonio Delgado-Arredondo et al., “Methodology for Fault Detection in Induction Motors Via Sound and Vibration Signals,” Mechanical Systems and Signal Processing, vol. 83, pp. 568-589, 2017.
[CrossRef] [Google scholar] [Publisher Link]
[3] Ali Namdar et al., “A Robust Stator Inter-Turn Fault Detection in Induction Motor Utilizing Kalman Filter-Based Algorithm,” Measurement, vol. 187, 2022.
[CrossRef] [Google scholar] [Publisher Link]
[4] Zuolu Wang et al., “Fault Identification of Broken Rotor Bars in Induction Motors Using an Improved Cyclic Modulation Spectral Analysis,” Energies, vol. 12, no. 17, 2019.
[CrossRef] [Google scholar] [Publisher Link]
[5] Anurag Choudhary et al., “Condition Monitoring and Fault Diagnosis of Induction Motors: A Review,” Archives of Computational Methods in Engineering, vol. 26, pp. 1221-1238, 2019.
[CrossRef] [Google scholar] [Publisher Link]
[6] Ola E. Hassan et al., “Induction Motor Broken Rotor Bar Fault Detection Techniques Based on Fault Signature Analysis-A Review,” IET Electric Power Applications, vol. 12, no. 7, pp. 895-907, 2018.
[CrossRef] [Google scholar] [Publisher Link]
[7] Majid Hussain et al., “Fault Detection and Identification Using Deep Learning Algorithms in Induction Motors,” Computer Modeling in Engineering and Sciences, vol. 133, no. 2, pp. 435-470, 2022.
[CrossRef] [Google scholar] [Publisher Link]
[8] Rafia Nishat Toma, Alexander E. Prosvirin, and Jong-Myon Kim, “Bearing Fault Diagnosis of Induction Motors Using a Genetic Algorithm and Machine Learning Classifiers,” Sensors, vol. 20, no. 7, 2020.
[CrossRef] [Google scholar] [Publisher Link]
[9] Jiangquan Zhang et al., “A New Bearing Fault Diagnosis Method Based on Modified Convolutional Neural Networks,” Chinese Journal of Aeronautics, vol. 33, no. 2, 2019.
[CrossRef] [Google scholar] [Publisher Link]
[10] Ana L. Martinez-Herrera et al., “Multiple Fault Detection in Induction Motors through Homogeneity and Kurtosis Computation,” Energies, vol. 15, no. 4, 2022.
[CrossRef] [Google scholar] [Publisher Link]
[11] Gholam Reza Agah et al., “Broken Rotor Bar and Rotor Eccentricity Fault Detection in Induction Motors Using a Combination of Discrete Wavelet Transform and Teager-Kaiser Energy Operator,” IEEE Transactions on Energy Conversion, vol. 37, no. 3, pp. 2199-2206, 2022.
[CrossRef] [Google scholar] [Publisher Link]
[12] Purushottam Gangsar, and Rajiv Tiwari, “Comparative Investigation of Vibration and Current Monitoring for Prediction of Mechanical and Electrical Faults in Induction Motor Based on Multiclass-Support Vector Machine Algorithms,” Mechanical Systems and Signal Processing, vol. 94, pp. 464-481, 2017.
[CrossRef] [Google scholar] [Publisher Link]
[13] M. Geethanjali and H. Ramadoss, “Fault Diagnosis of Induction Motors Using Motor Current Signature Analysis: A Review,” Advanced Condition Monitoring and Fault Diagnosis of Electric Machines, pp. 1-37, 2019.
[CrossRef] [Google scholar] [Publisher Link]
[14] Seyedali Mirjalili, Seyed Mohammad Mirjalili, and Andrew Lewis, “Grey Wolf Optimizer,” Advances in Engineering Software, vol. 69, pp. 46-61, 2014.
[CrossRef] [Google scholar] [Publisher Link]
[15] Yuxiang Hou et al., “Improved Grey Wolf Optimization Algorithm and Application,” Sensors, vol. 22, no. 10, 2022.
[CrossRef] [Google scholar] [Publisher Link]
[16] Jianwei Yang et al., “A Modified Gabor Filter Design Method for Fingerprint Image Enhancement,” Pattern Recognition Letters, vol. 24, pp. 1805-1817, 2003.
[CrossRef] [Google scholar] [Publisher Link]
[17] Seyedali Mirjalili, and Andrew Lewis, “The Whale Optimization Algorithm,” Advanced Engineering Software, vol. 95, pp. 51-67, 2016.
[CrossRef] [Google scholar] [Publisher Link]
[18] Zhiguang Wang, Weizhong Yan, and Tim Oates, “Time Series Classification from Scratch with Deep Neural Networks: A Strong Baseline,” International Joint Conference on Neural Networks (IJCNN), Anchorage, AK, USA, pp. 1578-1585, 2017.[CrossRef] [Google scholar] [Publisher Link]