Using Machine-Learning Application to model the Causal Relationship between Electricity Consumption and Economic Growth in Nigeria
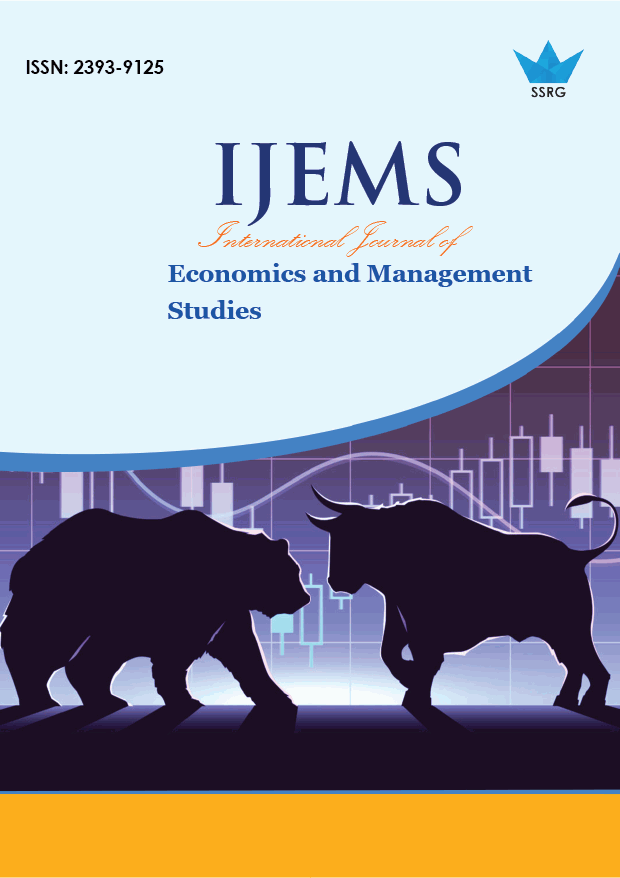
International Journal of Economics and Management Studies |
© 2023 by SSRG - IJEMS Journal |
Volume 10 Issue 12 |
Year of Publication : 2023 |
Authors : Etim Akan, Alwell Nteegah, Barisua Nwinee |
How to Cite?
Etim Akan, Alwell Nteegah, Barisua Nwinee, "Using Machine-Learning Application to model the Causal Relationship between Electricity Consumption and Economic Growth in Nigeria," SSRG International Journal of Economics and Management Studies, vol. 10, no. 12, pp. 1-11, 2023. Crossref, https://doi.org/10.14445/23939125/IJEMS-V10I12P101
Abstract:
This study examines the correlation between electricity consumption and economic growth in Nigeria using timeseries data between 1990 and 2020. The study uses a Recurrent Neural Network (RNN), a machine learning application, to model the relationship between electricity consumption and Gross Domestic Product (GDP), representing the independent and dependent variables, respectively. A 30-year time series data for both variables forms the input data used in training the model and examining the causal relationship between the two variables. The study's outcome compares the actual GDP plot against the predicted values. The predicted GDP values track the actual GDP values with a high degree of accuracy. The RNN establishes a strong correlation between electricity consumption and economic growth in the country and provides an option for making a forecast of future GDP growth trends that are linked to an electricity supply scenario. There is a significant supply-demand gap in the electricity market in Nigeria, which calls for substantial investments to be made to achieve infrastructure upgrades, network expansion and improvement in service quality in the industry.
Keywords:
Correlation, Electricity, GDP, Macroeconomics, RNN.
References:
[1] Samuel Adams, Edem Kwame Mensah Klobodu, and Eric Evans Osei Opoku, “Energy Consumption, Political Regime and Economic Growth in Sub-Saharan Africa,” Energy Policy, vol. 96, pp. 36-44, 2016.
[CrossRef] [Google Scholar] [Publisher Link]
[2] Adegbemi Babatunde Onakoya et al., “Energy Consumption and Nigerian Economic Growth: An Empirical Analysis,” European Scientific Journal, vol.9, no.4, pp. 25-40, 2013.
[Google Scholar] [Publisher Link]
[3] Adeola f. Adenikinju, “Analysis of the Cost of Infrastructure Failures in a Developing Economy: The Case of Electricity Sector in Nigeria,” African Economic Research Consortium AERC Research Paper 148, 2015.
[Google Scholar] [Publisher Link]
[4] Oluseyi O. Ajayi, and Kolawole O. Ajanaku, “Nigeria’s Energy Challenge and Power Development: The Way Forward,” Energy & Environment, vol. 20, no. 3, 2009.
[CrossRef] [Google Scholar] [Publisher Link]
[5] Laurene V. Fausett, Fundamentals of Neural Networks: Architectures, Algorithms and Applications, Prentice-Hall, 1994.
[Google Scholar] [Publisher Link]
[6] Simon S. Haykin, Neural Networks: A Comprehensive Foundation, Prentice Hall, pp. 1-842, 1999.
[Google Scholar] [Publisher Link]
[7] Sepp Hochreiter; and Jürgen Schmidhuber, “Long Short-Term Memory,” Neural computation, vol. 9, no. 8, pp. 1735-1780, 1997.
[CrossRef] [Google Scholar] [Publisher Link]
[8] F.I. Ibitoye, and A. Adenikinju, “Future Demand for Electricity in Nigeria,” Applied Energy, vol. 84, no. 5, pp. 492–504, 2007.
[CrossRef] [Google Scholar] [Publisher Link]
[9] I.D. Ibrahim et al., “A Review on Africa Energy Supply through Renewable Energy Production: Nigeria, Cameroon, Ghana, and South Africa as a Case Study,” Energy Strategy Reviews, vol. 38, 2021.
[CrossRef] [Google Scholar] [Publisher Link]
[10] Akin Iwayemi, “Nigeria’s Dual Energy Problems: Policy Issues and Challenges,” International Association for Energy Economics, 2008.
[Google Scholar] [Publisher Link]
[11] A.K. Jain, Jianchang Mao, and K.M. Mohiuddin, “Artificial Neural Networks: A Tutorial,” IEEE, vol. 29, no. 3, pp 31-44, 1996.
[CrossRef] [Google Scholar] [Publisher Link]
[12] Zhenya Liu, “Global Energy Development: The Reality and Challenges,” Global Energy Interconnection, pp. 1-64, 2015.
[CrossRef] [Google Scholar] [Publisher Link]
[13] Danilo P. Mandic, and Jonathon A. Chambers, Recurrent Neural Networks for Prediction: Learning Algorithms, Architectures, & Stability, John Wiley & Sons, pp. 1-304, 2001.
[Google Scholar] [Publisher Link]
[14] Mesbah Fathy Sharaf, “Energy Consumption and Economic Growth in Egypt: A Disaggregated Causality Analysis with Structural Breaks,” Topics in Middle Eastern and African Economies, vol. 18, no. 2, pp. 61-86, 2016.
[Google Scholar] [Publisher Link]
[15] N. Murata, S. Yoshizawa, and S. Amari, “Network Information Criterion-Determining the Number of Hidden Units for an Artificial Neural Network Model,” IEEE Transaction on Neural Networks, vol. 5, no. 6, pp. 865-872, 1994.
[CrossRef] [Google Scholar] [Publisher Link]
[16] Nnaji C.E., and Uzoma C. C, The CIA world factbook, Central Intelligence Agency, Skyhorse Publishing, 2010.
[Google Scholar] [Publisher Link]
[17] Obas John Ebohon, “Energy, Economic Growth, and Causality in Developing Countries: A Case Study of Tanzania and Nigeria,” Energy Policy, vol. 24, no. 5, pp. 447-453, 1996.
[CrossRef] [Google Scholar] [Publisher Link]
[18] D.M. Rodvold, “Software Development Process Model for Artificial Neural Networks in Critical Applications,” IJCNN’99. International Joint Conference on Neural Networks. Proceedings, IEEE, vol. 5, pp. 3317-3322, 1999.
[CrossRef] [Google Scholar] [Publisher Link]
[19] Sambo A. S. et al., “Nigeria’s Experience on the Application of IAEA’S Energy Models (MAED & WASP) for National Energy Planning,” Faculty of Science & Technology (FST), pp. 24–28.
[Google Scholar] [Publisher Link]