Predicting Neologisms for Marketing:A Text Mining Approach
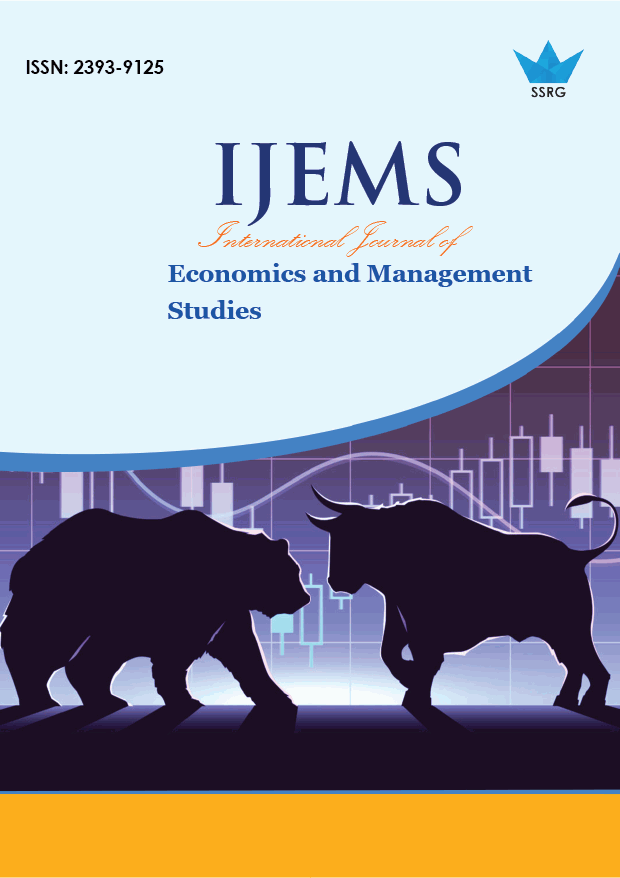
International Journal of Economics and Management Studies |
© 2020 by SSRG - IJEMS Journal |
Volume 7 Issue 7 |
Year of Publication : 2020 |
Authors : Sang-Uk Jung, Jungho Byun, Seongyeol Bae, Donghwi Song |
How to Cite?
Sang-Uk Jung, Jungho Byun, Seongyeol Bae, Donghwi Song, "Predicting Neologisms for Marketing:A Text Mining Approach," SSRG International Journal of Economics and Management Studies, vol. 7, no. 7, pp. 5-9, 2020. Crossref, https://doi.org/10.14445/23939125/IJEMS-V7I7P102
Abstract:
An increasing number of companies rely on neologisms when implementing their marketing strategy. However, companies recognize that indiscriminate use of neologisms can haveabadimpactonmarketing, requiring them to evaluate the pros and cons of the neologism they apply. To help on these issues, this research focuses on creating a model predicting neologisms that are appropriate for marketing use. Using data collected with web-crawling from Korea’s largest community website, 415,000 terms for 6 forums are examined with network analysis, text mining, and logistic regression. We find that ‘Negative’, ‘Summary’, ‘Korean’ are the most meaningful variables when predicting appropriate neologisms for marketing use in Korea. Our model predicts that the ‘Ja-gang-du-chun’ will be a buzzword next year. Up-to-date results will come out with the updated and supplementary data sets. These findings suggest a way for the practitioner to predict a buzzword and how to use it in marketing.
Keywords:
Neologism, Marketing Intelligence, Online Community, Text Mining, Korean.
References:
[1] Y. Ding, The study on the Korean vogue words, thesis, Chonnam National University, Kwangju, Korea,(2008).
[2] A. Kilgarriff, and G. Grefenstette, Introduction to the special issue on the web as corpus, Computational linguistics, 29 (2003) 333–347.
[3] M. E. Newman, Networks,An Introduction, Oxford, England: Oxford University Press, (2010).
[4] L. Romary, S. Salmon-Alt, and G. Francopoulo.Standards are going concrete: from LMF to Morphalou, In WorkshopEnhancing and Using Electronic Dictionaries, Geneva, Switzerland.
[5] S. Ollinger and M. Valette, La créativitélexicale:despratiquessociales aux textes. In Actes del I CongrésInternacional de Neologia de les llengüesromaniques, volume Publicacions de l’InstitutUniversitari de LingüísticaAplicada (IULA) de laUniversitatPompeuFabra (UPF), Barcelona, Spain, (2010) 965–876.
[6] I. Witten and E. Frank, Data Mining: Practical machine learning tools and techniques, 2nd Edition, Morgan Kaufmann, San Francisco, (2005).
[7] K. Wang and H. Wu. Research on neologism detection in entity attribute knowledge acquisition. In 2017 5th International Conference on Machinery, Materials and Computing Technology (ICMMCT 2017). Atlantis Press, (2017).
[8] I. Stewart and J. Eisenstein. Making fetch happen: The influence of social and linguistic context on nonstandard word growth and decline. In Proceedings of the 2018 Conference on Empirical Methods in Natural Language Processing, (2018) 4360–4370.
[9] J. Eisenstein, B. O’Connor, N. A Smith, and E. P. Xing, Diffusion of lexical change in social media,PloS one, vol. 9(11) ( 2014) e113114.
[10] T. J.Liu, S. K.Hsieh, andL. Prevot, Observing features of PTT neologisms: A corpus-driven study with N-gram model. In Proceedings of the 25th Conference on Computational Linguistics and Speech Processing (ROCLING 2013), (2013) 250-259.
[11] M. A. Ahmed, and M. A. Ahmed, Farmers’ Perception and Adaptation to Climate Change: Multinomial Logistic Regression Model Evidence, SSRG International Journal of Economics and Management Studies, 6(10)(2019) 110–119.