Associative Semantic Ranking Models of Satellite Images
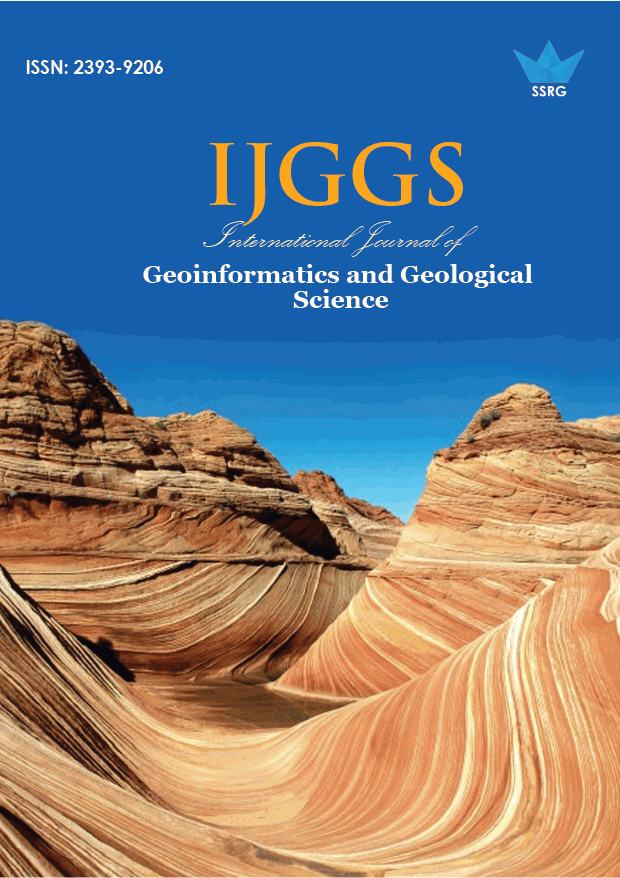
International Journal of Geoinformatics and Geological Science |
© 2015 by SSRG - IJGGS Journal |
Volume 2 Issue 3 |
Year of Publication : 2015 |
Authors : Dr. Kazuhiko I.E |
How to Cite?
Dr. Kazuhiko I.E, "Associative Semantic Ranking Models of Satellite Images," SSRG International Journal of Geoinformatics and Geological Science, vol. 2, no. 3, pp. 1-4, 2015. Crossref, https://doi.org/10.14445/23939206/IJGGS-V2I3P102
Abstract:
Associative procedures for content-based image position by semantics are gorgeous due to the correspondence of produced models to human models of considerate. Though they tend to return results that are better unstated by image analysts, the induction of these models is problematic to build due to factors that affect training density, such as synchronicity of visual patterns in same images, over-fitting or under-fitting and semantic representation differences among image analysts. This article proposes a methodology to reduce the complexity of ranking satellite images for associative methods. Our method employs genetic operations to afford faster and more correct models for ranking by semantic using low level features. The added exactness is provided by a decrease in the likelihood to reach local minima or to over fit. The investigates show that, using genetic optimization, associative methods perform better or at similar levels as state-of-the-art ensemble methods for ranking. The mean average precision (MAP) of ranking by semantic was improved by 14% over similar associative methods that use other optimization procedures while maintaining smaller size for each semantic model.
Keywords:
content-based image ranking, data mining, genetic, satellite images.
References:
[1] Datcu, M.; Seidel, K. Human-centered concepts for exploration and understanding of earth observation images. IEEE Trans. Geosci. Remote Sens. 2005, 43, 601–609.
[2] Tseng, M.-H.; Chen, S.-J.; Hwang, G.-H.; Shen, M.-Y. A genetic algorithm rule-based approach for land-cover classification. ISPRS J. Photogramm. 2008, 63, 202–212.
[3] Mennis, J.; Guo, D. Spatial data mining and geographic knowledge discovery—An introduction. Comput. Environ. Urban Syst. 2009, 33, 403–408.
[4] Datcu, M.; Seidel, K. Image Information Mining: Exploration of Image Content in Large Archives. In Proceedings of 2000 IEEE Aerospace Conference, Big Sky, MT, USA, 18–25 March 2000; Volume 3, pp. 253–264.
[5] Hsu, W.; Lee, M.L.; Zhang, J. Image mining: Trends and developments. J. Intell. Inf. Syst. 2002, 19, 7–23.
[6] Liu, Y.; Zhang, D.; Lu, G.; Ma, W.-Y. A survey of content-based image retrieval with high-level semantics. Pattern Recogn. 2007, 40, 262–282.
[7] Aksoy, S.; Cinbis, R. Image mining using directional spatial constraints. IEEE Geosci. Remote Sens. Lett. 2010, 7, 33–37.
[8] Barb, A.; Shyu, C.-R. Visual-semantic modeling in content-based geospatial information retrieval using associative mining techniques. IEEE Geosci. Remote Sens. Lett. 2010, 7, 38–42.