Lossless Image Solidity Using Neural Network
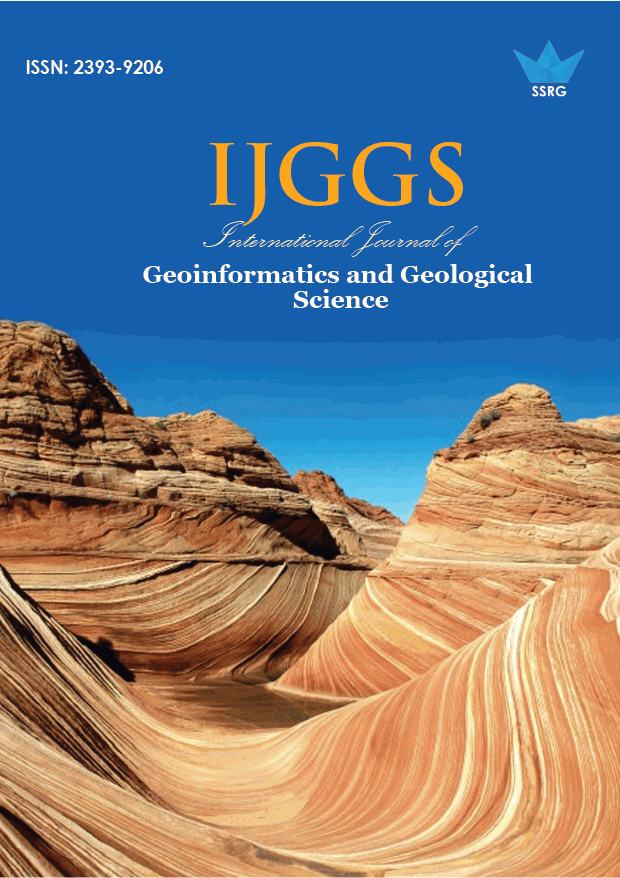
International Journal of Geoinformatics and Geological Science |
© 2014 by SSRG - IJGGS Journal |
Volume 1 Issue 2 |
Year of Publication : 2014 |
Authors : Dr.F.G.Duck-Young, Hyun-Shik |
How to Cite?
Dr.F.G.Duck-Young, Hyun-Shik, "Lossless Image Solidity Using Neural Network," SSRG International Journal of Geoinformatics and Geological Science, vol. 1, no. 2, pp. 1-4, 2014. Crossref, https://doi.org/10.14445/23939206/IJGGS-V1I2P101
Abstract:
In this paper, new multilayer perceptron’s feed forward back propagation Neural Network (NN) performance using BFGS quasi newton , Levenberg -Marquardt (LM), Gradient descent back propagation with adaptive learning rate(GDA) Algorithms are being anticipated with the project detached to develop a lossless image solidity technique using NN and to design and contrivance image compression using Neural network to achieve maximum peak signal to noise ratio (PSNR), and low mean square error (MSE) and compression levels. This paper presents a NN based procedure that may be practical to data compression and breaks down large images into smaller blocks (1x64) and eradicates redundant information. Lastly, this technique uses a NN training functions like and conversion of block codes to vector codes and vice versa. Results obtained with proposed techniques leads to better compression material. Finally, this technique uses a NN training functions like and adaptation of block codes to vector codes and vice versa. Consequencesacquired with proposed techniques leads to better compression ratio at the same time conserving the image quality. The investigational result shows that the BFG quasi newton algorithm is best among the three proposed algorithm which offers better PSNR value and also reduces the MSE value.
Keywords:
Neural network (NN), Multilayer perceptron’s, peak signal to noise ratio (PSNR), mean square error (MSE).
References:
[1] H. Vila Gaidhane and Vijander Singh, “New Approaches for Image Compression Using Neural Network “ Journal of Intelligent Learning Systems and Applications , vol 3, 2011, 220-229.
[2] Samrat Deb Choudhury and Md. Anwar Hussain, “Digital Image Compression using Neural Networks”, 2009 International Conference on Advances in Computing, Control, and Telecommunication Technologies, 2009 IEEE.
[3] LI Huifang and LI Mo, “A New Method Of Image Compression Based On Quantum neural Network “2010 International Conference of Information Science and Management Engineering, 2010 IEEE.
[4] H. Veisi and Jamzad ,"Image Compression Using Neural Networks", Image Processing and Machine Vision Conference (MVIP), 2005 Tehran, Iran .
[5] W. J. Tang, Q. H. Wu, and J. R. Saunders. “Bacterial foreging algorithm for dynamic environments”. 2006 IEEE Conferences on Evolutionary Computation, Vancouver, Canada, , 2006 July 16–21, 4467–4473.
[6] A. Rahman Chowdhury, Mofizur Rahman, “A New Approach for Compressing Color Images using Neural Network”, Proceedings of International Conference on Computational Intelligence for Modeling, Control and Automation – CIMCA 2003 ,Vienna, Austria .
[7] S. F. Wang and G. L. Li. “Application of a BP neural network based on genetic algorithm in image compression. Chinese Journal of Quantum Electronics, 2007. 24 ,4, 425–428 (in Chinese).
[8] Watanabe, Eiji, and Mori, Katsumi, "Lossy image compression using a modular structured neural Network," Proc. of IEEE signal processing society workshop, and 2001 pp.403-412.