Application of Expert Methods for Optimizing and Predicting the Ultimate Tensile Strength of Mild Steel Weldment
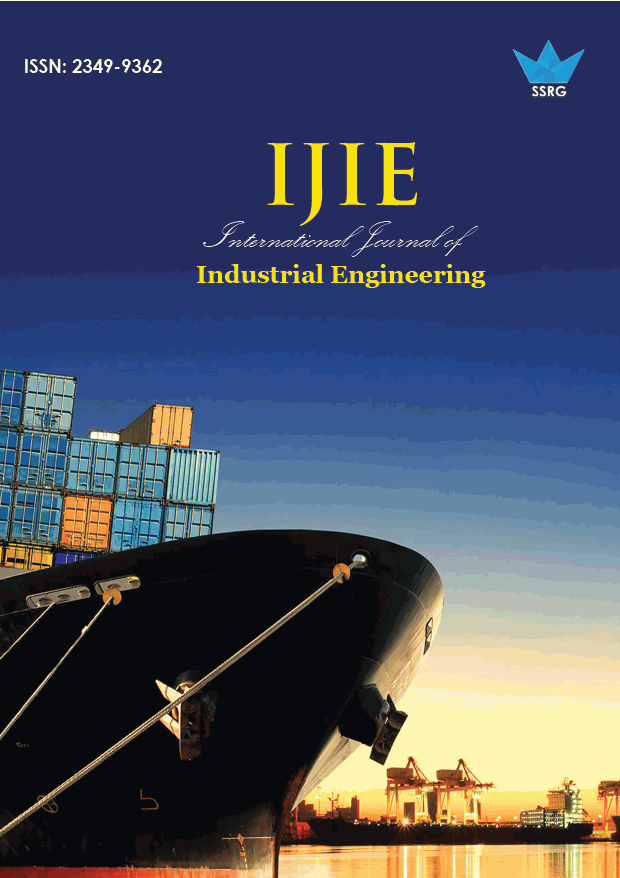
International Journal of Industrial Engineering |
© 2025 by SSRG - IJIE Journal |
Volume 12 Issue 1 |
Year of Publication : 2025 |
Authors : Chukwunedum Ogochukwu Chinedum, Ekwueme Onyekachukwu Godspower, Anizoba, Daniel Chinazom |
How to Cite?
Chukwunedum Ogochukwu Chinedum, Ekwueme Onyekachukwu Godspower, Anizoba, Daniel Chinazom, "Application of Expert Methods for Optimizing and Predicting the Ultimate Tensile Strength of Mild Steel Weldment," SSRG International Journal of Industrial Engineering, vol. 12, no. 1, pp. 32-43, 2025. Crossref, https://doi.org/10.14445/23499362/IJIE-V12I1P104
Abstract:
This research study focuses on designing models to optimize and predict the ultimate tensile strength of mild steel weldment by the use of response surface methodology and artificial neural network analyses. The input variables are current, voltage, and gas flow rate. Ultimate Tensile Strength (UTS) is the response variable. The welding method used is the Tungsten Inert Gas (TIG) welding process. Ultimate Tensile Strength (UTS) was adopted in this research study to measure weld quality, as it is a main mechanical property that can define weld joint efficiency. The adequately optimized response variable certainly will aid in achieving an improved weld with the preferred strength and quality. The response surface methodology analyses yielded the optimal solutions to be: current, 180.00Amps; voltage, 21.672Volts and gas flow rate, 15.504L/min, for the input parameters, and 579.000MPa for the response variable. These optimal solutions, the RSM analyses, gave the Global Desirability (Dg) of achieving to be 83.62%. Weld current has the most significant effect on the response variable, as shown by the variance analysis (ANOVA) result. The predicted optimal solution for the response variable is 530.077MPa by the artificial neural network analyses, with an overall strong correlation (R) between the input parameters and the response variable of 99.893%. Deductively, it is recommended that the optimal solutions be used for modeling and application, whereas the optimal solution of the artificial neural network analyses obtained is better and more robust for practical implementation considering its higher Regression (R) value. Therefore, the results are recommended for more idealistic decision-making.
Keywords:
ANN, Mild Steel, RSM, TIG, Ultimate Tensile Strength.
References:
[1] Haider Jabaur Abid et al., “Experimental Investigation of the Effect of Spot-Welding Process Parameters on the Tensile Strength of Similar Metal Joints,” International Review of Mechanical Engineering, vol. 15, no. 2, pp. 89-95, 2021.
[CrossRef] [Google Scholar] [Publisher Link]
[2] Nehar Ansari, and Lokesh Singh, “Effects on Hardness and Penetration on Changing Process Parameters in TIG Welding Using Optimization Techniques,” International Journal of Innovations in Engineering and Science, vol. 4, no. 12, pp. 13-25, 2019.
[Publisher Link]
[3] Carolina Payares-Asprino, “Prediction of Mechanical Properties as a Function of Welding Variables in Robotic Gas Metal Arc Welding of Duplex Stainless Steels SAF 2205 Welds through Artificial Neural Networks,” Advances in Materials Science, vol. 21, no. 3 (69), pp. 75-90, 2021.
[CrossRef] [Google Scholar] [Publisher Link]
[4] Meiyan Feng et al., “Welding Penetration and Mechanical Properties of Welded Joints of V-Shaped Surface Grooves,” Research Square, 2022.
[CrossRef] [Google Scholar] [Publisher Link]
[5] Raad Jamal Jassim et al., “Influence of Welding Parameters on Optimization of the Tensile Strength and Peak Temperature in AISI 1020 Alloy Joints Welded by SAW,” AIP Conference Proceedings, vol. 2660, 2022.
[CrossRef] [Google Scholar] [Publisher Link]
[6] Jaroslaw Szusta et al., “Effect of Welding Process Parameters on the Strength of Dissimilar Joints of S355 and Strenx 700 Steels Used in the Manufacture of Agricultural Machinery,” Materials, vol. 16, no. 21, pp. 1-30, 2023.
[CrossRef] [Google Scholar] [Publisher Link]
[7] Samir Khrais et al., “Impact of Gas Metal Arc Welding Parameters on Bead Geometry and Material Distortion of AISI 316L,” Journal of Manufacturing and Materials Processing, vol. 7, no. 4, pp. 1-21, 2023.
[CrossRef] [Google Scholar] [Publisher Link]
[8] Noor Ajian Mohd-Lair et al., “SMAW: The Effects of Currents and Welding Rod Diameters on Welded Joint Ultimate Tensile Strength Using the Full Factorial DOE,” Journal of Physics: Conference Series, 1st International Conference on Material Processing and Technology, Perlis, Malaysia, vol. 2129, pp. 1-8, 2021.
[CrossRef] [Google Scholar] [Publisher Link]
[9] Osarobo Osamede Ogbeide, Kolawole Akeredolu, and Samuel Ayodeji Omotehinse, “Optimization of Tensile Strength of Butt Joint Weldment on Mild Steel Plate Using Response Surface Methodology,” Journal of Applied Research on Industrial Engineering, vol. 9, no. 1, pp. 50-58, 2022.
[CrossRef] [Google Scholar] [Publisher Link]
[10] C. Shravan et al., “A Review on Welding Techniques: Properties, Characterisations and Engineering Applications,” Advances in Materials and Processing Technologies, vol. 10, no. 2, pp. 1126-1181, 2023.
[CrossRef] [Google Scholar] [Publisher Link]
[11] Tushar Sonar et al., “A Comprehensive Review on Fusion Welding of High Entropy Alloys – Processing, Microstructural Evolution and Mechanical Properties of Joints,” International Journal of Lightweight Materials and Manufacture, vol. 7, no. 1, pp. 122–183, 2024.
[CrossRef] [Google Scholar] [Publisher Link]
[12] Suprianto, Rian Dede Syahputra, and Farida Ariani, “Study on Microstructure Evolution and Mechanical Properties of Similar Joint of Al-Mg-Si Alloy by Tungsten Inert Gas Welding,” E3S Web of Conferences: 5th Talenta Conference on Engineering, Science and Technology (TALENTA CEST-5 2024), vol. 519, 2024.
[CrossRef] [Google Scholar] [Publisher Link]