A Method for Joint Compressing and Recovering Destructed Signals in Wireless Multimedia Sensor Networks
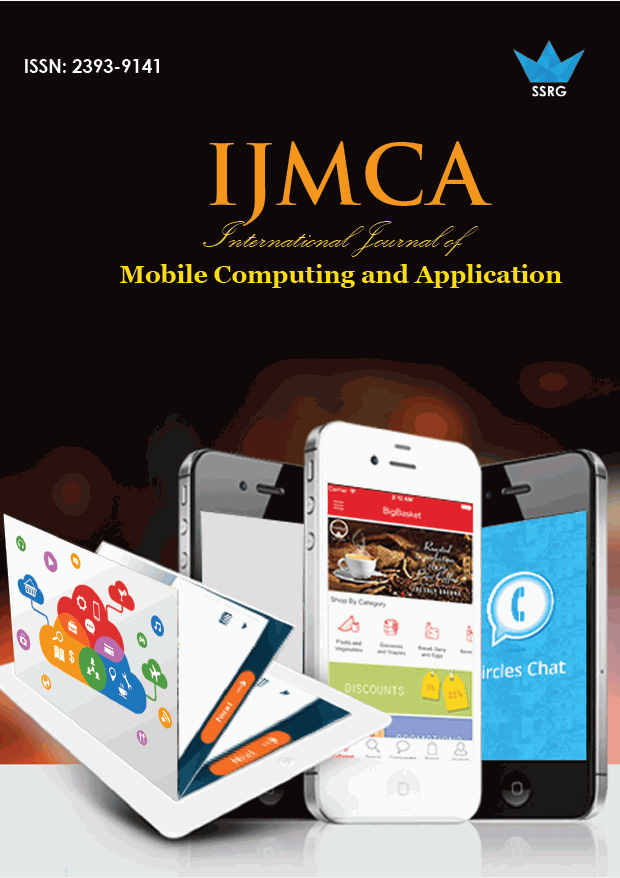
International Journal of Mobile Computing and Application |
© 2014 by SSRG - IJMCA Journal |
Volume 1 Issue 2 |
Year of Publication : 2014 |
Authors : M. Eslami, F. Torkamani-Azar and E. Mehrshahi |
How to Cite?
M. Eslami, F. Torkamani-Azar and E. Mehrshahi, "A Method for Joint Compressing and Recovering Destructed Signals in Wireless Multimedia Sensor Networks," SSRG International Journal of Mobile Computing and Application, vol. 1, no. 2, pp. 16-20, 2014. Crossref, https://doi.org/10.14445/23939141/IJMCA-V1I3P105
Abstract:
The WSNs are developed to sense, gather, process and transmit the real-world information and so in recent years, solving numerous challenges of wireless sensor networking are considered intensely. In addition, in Wireless Multimedia Sensor Networks intra- and intersignal correlations can be exploited in the theory of distributed source coding and similarly in distributed compressive sensing to compress signals as much as possible. These cases may be occurred in applications and services in which work with smart spaces and context aware networks.In this paper based on compressive sensing a framework denoted asECMis proposed to compress and reconstruct the signals of the sensors even for networks which the data transmission is imperfect.ECM uses the concepts of distributed compressive sensing and the shared information between sensor's signals to compress the signals more.
Keywords:
Wireless Sensor Network, Distributed Compressive Sensing, Signal Compression,
References:
[1] I.F. Akyildiz, Wireless multimedia sensor networks: Application and Testbeds, Proceedings of the IEEE, 96(10), 2008, 1588-1605.
[2] W. Wang, D. Peng et al.,Cross-layer Multirate Interaction with Distributed Source Coding in Wireless Sensor Networks,IEEE Transactions on Wireless Communication, 8(2), 2011, 787-795.
[3] D.L. Donoho, Compressed sensing,IEEE Transaction on Information Theory, vol. 52, no. 4, 2006, pp. 1289-1306.
[4] D. Baron, M.B. Wakin et al.,Distributed Compressed Sensing, Preprint, arXiv:0901.3403v1, 2006.
[5] J.Y. Park, M.B Wakin, A geometric approach to multi-view compressive imaging,EURASIP Journal on Advances in Signal Processing, 2012(1),2012.
[6] B. Wilburn, N. Joshi, V, Vaish, E.V. Talvala, E. Antunez, A. Barth, A. Adams, M. Horowitz, M. Levoy, High Performance Imaging Using Large Camera Arrays, ACM Transactions on Graphics, 24(3), 2005, 765-776.
[7] L.W. Kang, C.S. Lu, Distributed compressive video sensing, IEEE International Conference, ICASSP, 2009, 1169 - 1172.
[8] M. Elad, M.A.T. Figueiredo, M. Yi,On the Role of Sparse and Redundant Representations in Image Processing, Proceedings of the IEEE, 98(6), 2010, 972 - 982.
[9] P.R. Gill, A. Wang, A. Molnar,The In-Crowd Algorithm for Fast Basis Pursuit Denoising, IEEE Transactions on Signal Processing, 59(10), 2011, 4595 - 4605.
[10] Z. Wang , X. Yang, Blind channel estimation for ultra wide-band communications employing pulse position modulation, IEEE Signal Processing Letters, 12(7), 2005, 520 - 523.
[11] M. Grant, S. Boyd,CVX: Matlab software for disciplined convex programming, version 2.0 beta. June 2014, http://cvxr.com/cvx, June 2014.
[12] Sparselab, Accessed June 2014, http://sparselab.stanford.edu/.