Comparison Between WEKA and Salford Systemin Data Mining Software
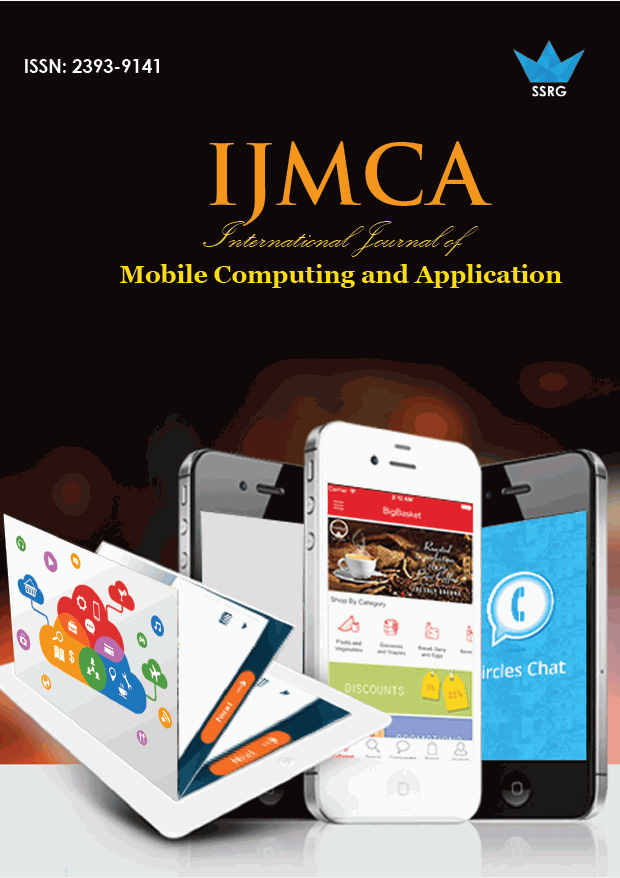
International Journal of Mobile Computing and Application |
© 2016 by SSRG - IJMCA Journal |
Volume 3 Issue 2 |
Year of Publication : 2016 |
Authors : Wirda Fitriani and Andysah Putera Utama Siahaan |
How to Cite?
Wirda Fitriani and Andysah Putera Utama Siahaan, "Comparison Between WEKA and Salford Systemin Data Mining Software," SSRG International Journal of Mobile Computing and Application, vol. 3, no. 2, pp. 18-21, 2016. Crossref, https://doi.org/10.14445/23939141/IJMCA-V3I4P101
Abstract:
Processing of the data generated from transactions that occur every day which resulted in nearly thousands of data per day requires software capable of enabling users to conduct a search of the necessary data. Data mining becomes a solution for the problem. To that end, many large industries began creating software that can perform data processing. Due to the high cost to obtain data mining software that comes from the big industry, then eventually some communities such as universities eventually provide convenience for users who want just to learn or to deepen the data mining to create software based on open source. Meanwhile, many commercial vendors market their products respectively. WEKA and Salford System are both of data mining software. They have the advantages and the disadvantages. This study is to compare them by using several attributes. The users can select which software is more suitable for their daily activities.
Keywords:
Data Mining, Decision Tree, Software
References:
[1] M. J. Berry, G. Linoff, Data Mining Techniques: For Marketing, Sales, and Customer Support, New York: John Wiley & Sons, Inc, 1997.
[2] A. Kumar, O. Singh, V. Rishiwal, R. K. Dwivedi, R. Kumar, “Association Rule Mining On Web Logs For Extracting Interesting Patterns Through Weka Tool,” International Journal of Advanced Technology In Engineering And Science, vol. 3, no. 1, pp. 134-140, 2015.
[3] D. T. Larose, Data Mining Methods and Models, Canada: A John Wiley & Sons, Inc, 2006.
[4] C. D., Discovering Knowledge in Data: An Introduction to Data Mining, Canada: John Wiley & Sons, 2014.
[5] M. Hall, E. Frank, G. Holmes, B. Pfahringer, P. Reutemann, I. H. Witten, “The WEKA Data Mining Software: An Update,” SIGKDD Explorations, vol. 11, no. 1, pp. 10-18, 2015.
[6] T. Krishna, D. Vasumathi, “A Study of Mining Software Engineering Data and Software Testing,” Journal of Emerging Trends in Computing and Information Sciences, vol. 2, no. 11, 2011.
[7] T. Silwattananusarn, A. D. KulthidaTuamsuk, “Data Mining and Its Applications for Knowledge Management : A Literature Review from 2007 to 2012,” International Journal of Data Mining & Knowledge Management Process, vol. 2, no. 5, 2012.
[8] S. Rajagopal, “Customer Data Clustering Using Data Mining Technique,” International Journal of Database Management Systems, vol. 3, no. 4, pp. 1-11, 2011.
[9] D. Tomar, S. Agarwal, “A survey on Data Mining approaches for Healthcare,” International Journal of Bio-Science and Bio-Technology, vol. 5, no. 5, pp. 241-266, 2013.
[10] A. P. U. Siahaan, “Various Patterns of Data Mining Techniques,” 2011. [Online]. Available: http://www.academia.edu/download/46339017/Various_Patterns_of_Data_Mining_Techniques.doc. [Access: 10 7 2016].