Automatic Machine Learning Forgery Detection Based On Svm Classifier
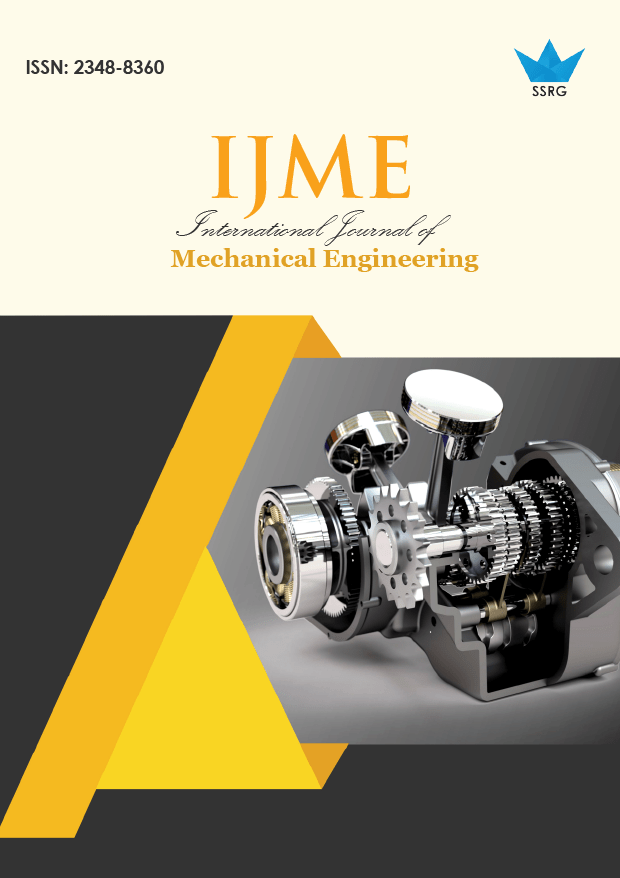
International Journal of Mechanical Engineering |
© 2014 by SSRG - IJME Journal |
Volume 1 Issue 1 |
Year of Publication : 2014 |
Authors : S.L.Jothilakshmi, K Valli, A.Vanitha, A. Selva nathiya, A.Shunmuga priya |
How to Cite?
S.L.Jothilakshmi, K Valli, A.Vanitha, A. Selva nathiya, A.Shunmuga priya, "Automatic Machine Learning Forgery Detection Based On Svm Classifier," SSRG International Journal of Mechanical Engineering, vol. 1, no. 1, pp. 1-5, 2014. Crossref, https://doi.org/10.14445/23488360/IJME-V1I1P101
Abstract:
Photographers are able to create composites of analog pictures, this process is very time consuming and require expert knowledge. In digital image the editing software makes modifications in straight forward. In this project analyze one of the most common form of photographic manipulation known as image composition or splicing.For that propose a forgery detection method is used to exploits subtle inconsistencies in the colour of the illumination of images. The technique ( Machine Learning) is applicable to images containing two or more people. To achieving this concept, the information from physics (chromaticity)- and statistical (texture and edge) - based illuminate estimators on image regions of similar images are taken. Then the extracted texture, skin pigmentation - and edge - based features are provided to a machine - learning approach for automatic decision - making. The classification performance achieved by an SVM (Support Vector Machine) meta - fusion classifier.
Keywords:
Color constancy, illuminant color, image forensics, machine learning, spliced image detection, texture and edge descriptors.
References:
[1]A.Rocha,W.Scheirer,T.E.Boult,andS.Goldenstein,“Vision of the unseen: Current trends and challenges in digital image and video
forensics,” ACM Comput. Surveys, vol. 43, pp. 1–42, 2011.
[2] C. Riess and E. Angelopoulou, “Scene illumination as an indicator of image manipulation,” Inf. Hiding , vol. 6387, pp. 66–80, 2010.
[3] H. Farid and M. J. Bravo, “Image forensic analyses that elude the human visual system,” inProc. Symp. Electron. Imaging (SPIE), 2010, pp. 1–10
[4] Y. Ostrovsky, P. Cavanagh, and P. Sinha, “Perceiving illumination in-consistencies in scenes,”Perception, vol. 34, no. 11, pp. 1301–1314, 2005.
[5] H. Farid, A 3-D lighting and shadow analysis of the JFK Zapruder fi lm (Frame 317), Dartmouth College, Tech. Rep. TR2010–677, 2010.
[6] M. Johnson and H. Farid, “Exposing digital forgeries by detecting in-consistencies in lighting,” in Proc. ACM Workshop on Multimedia and Security, New York, NY, USA, 2005, pp. 1–10.
[7]M.JohnsonandH.Farid,“Exposing digital forgeries in complex
lighting environments,” IEEE Trans. Inf. Forensics Security ,vol.3,
no. 2, pp. 450–461, Jun. 2007.
[8] M. Johnson and H. Farid, “Exposing digital forgeries through specular highlights on the eye,” in Proc. Int. Workshop on Inform. Hiding, 2007,pp. 311–325.
[9] E. Kee and H. Farid, “Exposing digital forgeries from 3-D lighting en-vironments,” in Proc. IEEE Int. Workshop on Inform. Forensics and
Security (WIFS) , Dec. 2010, pp. 1–6.
[10] W. Fan, K. Wang, F. Cayre, and Z. Xiong, “3D lighting-based image forgery detection using shape-from-shading,” in Proc. Eur. Signal Pro-cessing Conf. (EUSIPCO) , Aug. 2012, pp. 1777–1781.
[11] J. F. O’Brien and H. Farid, “Exposing photo manipulation with incon-sistent re fl ections,” ACM Trans. Graphics , vol. 31, no. 1, pp. 1–11, Jan.2012.
[12] S. Gholap and P. K. Bora, “Illuminant colour based image forensics,” in Proc. IEEE Region 10 Conf., 2008, pp. 1–5.
[13] X. Wu and Z. Fang, “Image splicing detection using illuminant color in-consistency,” in Proc. IEEE Int. Conf. Multimedia Inform. Networking and Security, Nov. 2011, pp. 600–603.
[14] P. Saboia, T. Carvalho, and A. Rocha, “Eye specular highlights telltales for digital forensics: A mach ine learning ap proach,” in Proc. IEEE Int. Conf. Image Processing (ICIP), 2011, pp. 1937–1940.
[15] C. Riess and E. Angelopoulou, “Physics-based illuminant color esti-mation as an image semantics clue,” inProc. IEEE Int. Conf. Image Processing, Nov. 2009, pp. 689–692.
[16] K. Barnard, V. Cardei, and B. Funt, “A comparison of computational color constancy algorithms–Part I: Methodology and Experiments With Synthesized Data,”IEEE Trans. Image Process. ,vol.11,no.9, pp. 972–983, Sep. 2002.
[17]K.Barnard,L.Martin,A.Coath,andB.Funt,“Acomparisonof
computational color constancy algorithms – Part II: Experiments With Image Data,” IEEE Trans. Image Process., vol. 11, no. 9, pp. 985–996, Sep. 2002.