A review work on image retrieval of content-based and shape-based method
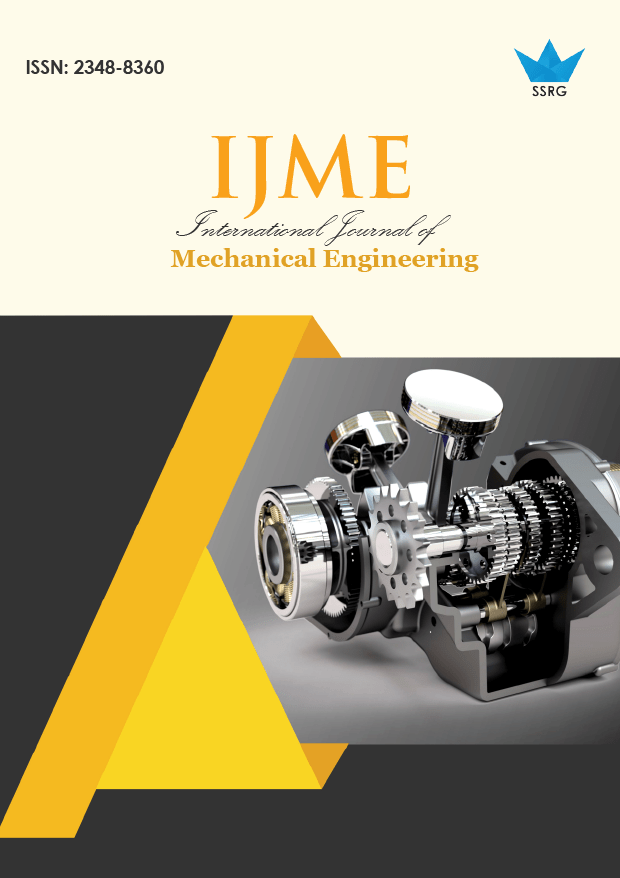
International Journal of Mechanical Engineering |
© 2019 by SSRG - IJME Journal |
Volume 6 Issue 8 |
Year of Publication : 2019 |
Authors : Ahammad Hossain , Md. Sojib Kaisar , Dr. Md. Abdul Hakim Khan |
How to Cite?
Ahammad Hossain , Md. Sojib Kaisar , Dr. Md. Abdul Hakim Khan, "A review work on image retrieval of content-based and shape-based method," SSRG International Journal of Mechanical Engineering, vol. 6, no. 8, pp. 26-32, 2019. Crossref, https://doi.org/10.14445/23488360/IJME-V6I8P104
Abstract:
More and more images have been generated in digital form around the world, due to the decreasing storage and processing costs and the internet. There is a growing interest in finding images in huge collections or from remote databases. In order to find an image, the image has to be narrated or represented by certain features. Shape is a significance visual feature of an image. Looking for images using shape features has attracted much recognition. Shape is one of the primary low-level image features exploited in the newly emerged content-based image retrieval (CBIR). Many shape methods exist. We study many shape representation and description techniques in the literature. However, in this dissertation it has been shown that Fourier descriptor-based methods have better performance for contour-based image search whereas shock graph based skeletal methods shows better accuracy for interior based image search depending on the applications.
Keywords:
retrieval, Texture, Fourier descriptor, shape descriptor
References:
[1] Chaudhuri, D. (2013). Global Contour and Region Based Shape Analysis and Similarity Measures. Defence Science Journal, 74-88.
[2] Chia-Hung W, Y. L.-y.-T. (2009). Trademark Image Retrieval Using Synthetic Feature for Describing Global Shape and Interior Structure. Pattern Recogn., 386-394.
[3] Dengsheng Zhang*, G. L. (2005). Study and evaluation of different Fourier methods for image retrieval. Image and Vision Computing, 33-49.
[4] G.J. Lu, A. S. (1999). Region-baesd shape representation and similarity measure suitable for cbir. Multimedia syst., 165-174.
[5] Goh, W.-B. (2008). Strategies for Shape Matching Using Skeleton. Comput. Vis. Image Underst., 326-345.
[6] Heng Q, K. L. (2010). An Effective Solution for Trademark Image Retrieval by Combining Shape Description and Feature Matching. Pattern Recogn., 2017-2027.
[7] Khanam S, J. S. (2009). An Improved Shock Graph-based Edit Distance Approach Using an Adaptive Weighting Scheme. Proc. Int. Conf. on Future Generation Communication and Networking, Springer-Verlag, Berlin Heidelberg, 501-508.
[8] Khanam S, J. S. (2011). Fast and Simple 2D Shape Retrieval Using Discrete Shock Graph. IEICE Trans. Info. Syst., 2059-2062.
[9] Klein P, T. S. (1998). Indexing Based on Edit Distance Matching of Shape Graphs. Multimedia storage and archiving systems III, 25-36.
[10] Sebastian TB, K. P. (2002). Shock-Based Indexing into Large Shape Databases. Proc. European Conf. (pp. 731-746). Computer Vision.
[11] Sebastian, T. B. (2001). Alignment-Based Recognition of Shape Outlines. Proc. Int’l Workshop Visual Form., 606-618.
[12] Sebastian, T. K. (2004). Recognition of Shapes by Editing Their Shock Graphs. Patt. Anal. Mach. Intel.IEEE Trans., 550-571.
[13] Xidao L.,Yuxiang X,Lili Z., Xin Z ,Chen L, and Jingmeng H. (2018).An Image Similarity Acceleration Detection Algorithm Based on Sparse Coding.Hindawi, Volume 2018, Article Id 1917421.
[14] Li, Y. A. (2012). Scene text detection via stroke width. Pattern Recognition (ICPR), 2012 21st International Conference on. IEEE, 2012. IEEE.