Artificial Neural Network Analysis For Domestic Refrigerator With Hydrocarbon Refrigerant Mixtures
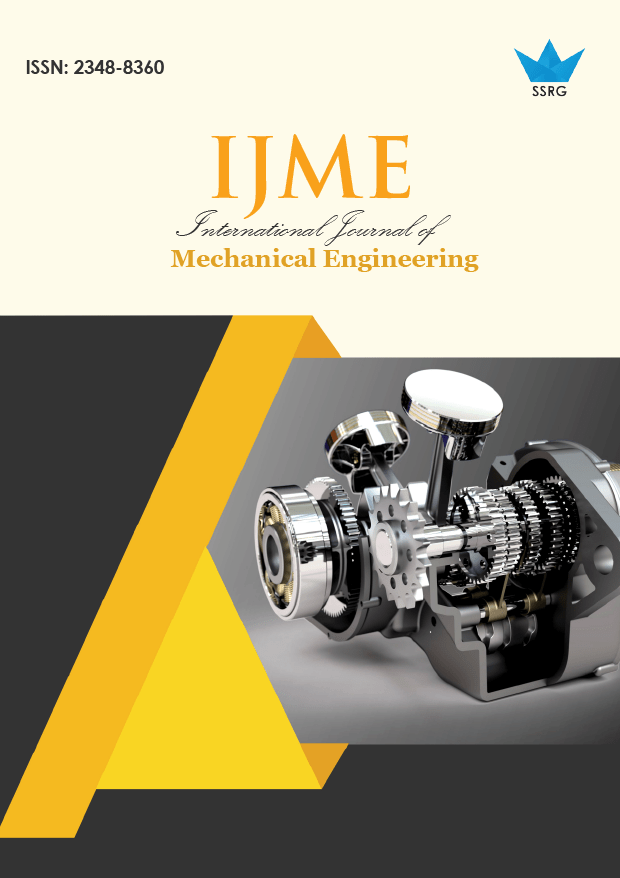
International Journal of Mechanical Engineering |
© 2021 by SSRG - IJME Journal |
Volume 8 Issue 6 |
Year of Publication : 2021 |
Authors : D.V. Raghunatha Reddy, P. Bhramara, K. Govindarajulu |
How to Cite?
D.V. Raghunatha Reddy, P. Bhramara, K. Govindarajulu, "Artificial Neural Network Analysis For Domestic Refrigerator With Hydrocarbon Refrigerant Mixtures," SSRG International Journal of Mechanical Engineering, vol. 8, no. 6, pp. 4-8, 2021. Crossref, https://doi.org/10.14445/23488360/IJME-V8I6P102
Abstract:
This study deals with the usage of Artificial Neural Network (ANN) modeling to predict the domestic refrigerator performances such as refrigeration affects power consumption and the coefficient of performance with hydrocarbon refrigerant mixtures (H.C.M.). Experimental work has conducted to obtain the data to train and test the models. The back-propagation algorithms used as a learning algorithm of ANN in the multilayered feedforward networks. Based on the design of experiments, Taguchi's L25 orthogonal array are used by varying Mass of refrigerant (Mf) length of the capillary tube (Lc) Evaporating temperature(Te) and condenser temperature(Tc) were used as input parameters. To develop ANN model has checked for adequacy and significance to systems performance. Finally, the results of the ANN model were compared and analyzed with experimental values. It is shown that while ANN model was good approaches were deficient in predicting desired output parameters, more accurate results were obtained with experimental results.
Keywords:
H.C.M., Lc, Mf, Artificial Neural Network, Domestic Refrigeration System, Power consumption, and Coefficient of performance.
References:
[1] T. Tasi, 2005 An overview of environmental hazards and exposure and explosive risk of hydrofluorocarbon HFCs, Chemosphere 61, 1539–1547.
[2] E. Johnson, Global warming from HFC, 1998 Environ. Impact Assessment Rev. 18, 485–492.
[3] P. Ashford, D. Clodic, A. McCulloch, L. Kuijpers, 2004 Emission profiles from the foam and refrigeration sectors comparison with atmospheric concentrations, Part 2: Results and discussion, Int. J. of Refrig. 27, 701– 716.
[4] Reddy, DVR., Bhramara, P., &Govindarajulu, K., 2012 Performance, and Optimization of Capillary Tube Length In a Split Type Air Conditioning System, International Journal of Engineering Research &Technology (IJERT), 1 (7), 1-11.
[5] R. Radermacher, K. Kim, 1996 Domestic refrigerators: recent developments, Int. J. Refrig. 19, 61–69.
[6] SomchaiWongwises and Nares Chimres 2005 “Experimental study of hydrocarbon mixtures to replace HFC-134a in a domestic refrigerator”, Energy Conversion and Management, Energy Conversion and Management, Vol.46, pp. 85-100.
[7] Mani K and Selladurai V, 200, Experimental analysis of new refrigerant mixture as a drop-in replacement for CFC12 and HFC134a. International Journal of Thermal Science, Vol.47, pp.1490-500.
[8] M. Mohanraj., S. Jayaraj., C. Muraleedharanand P. Chandrasekar 2009, Experimental investigation of R290/R600a mixture as an alternative to R134a in a domestic refrigerator, International Journal of Thermal Sciences, Vol.48, pp.1036-1042.
[9] M.Y. Lee., D.Y. Lee and Y. Kim, 2008 “Performance characteristics of a small-capacity direct cool refrigerator using R290/R600a (55/45)”, International Journal of Refrigeration, Vol.31, pp.734-741.
[10] Reddy DVR, Bhramara P, and Govindarajulu K. 2018, Experimental evaluation of the effect of refrigerant charge and capillary tube length on the performance of household refrigerator with different configurations of R290 and R600a. Mater Today: Proc.5: 11845–11852.
[11] M. Fatouh and M Kafary 2006 Assessment of propane/commercial butane mixtures as possible alternatives to R134a in domestic refrigerators, Energy Conservation and Management, 47, 2644-2658.
[12] Ching-Song Jwo., Chen-ChingTing, and Wei-Ru Wang, 2009 Efficiency analysis of home refrigerators by replacing hydrocarbon refrigerants," Measurement, 42, 697-70.
[13] Reddy DVR, P. Bhramara, K. Govindarajulu, 2016 , Hydrocarbon refrigerant mixtures as an alternative to R134a in the domestic refrigeration system: the state-of-the-art review, Int. J. Scientific Eng. Res. 7 (6) 87–93 (2016).
[14] Mehdi Rasti, Aghamiriand Mohammad Sadegh, 2013, Energy efficiency enhancement of a Domestic refrigerator using R436A and R600a as alternative refrigerants to R134a, International Journal of Thermal Sciences, 74, 86-94.
[15] Chao-Chieh Yu & Tun-Ping Ting, 2014, Retrofit assessment of refrigerator using hydrocarbon refrigerants, Applied Thermal Engineering, 6, 507-518.
[16] Deepak Paliwal and S.P.S.Rajput 2015, Experimental Analysis of Hydrocarbon Mixtures as the new Refrigerant In Domestic Refrigeration System, International Journal of Scientific & Engineering Research, 6, 623-627.
[17] D. Colbourne, T.J. Ritter, 2000 Compatibility of non-metallic materials with hydrocarbon refrigerants and lubricant mixtures, I.I.F.–I.I.R. Commission B1, B2, E1 and E2, Purdue University, U.S.A.
[18] Kalogirou SA. 200 Application of artificial neural-networks for energy systems. Appl Energy ;67: 17–35.
[19] Pacheco-Vega A, Sen M, Yang KT, McClain RL. 2014 Neural network analysis of fin-tube refrigerating heat exchanger with limited experimental data. Int J Heat Mass Transfer ;44: 763–770.
[20] Bechtler H, Browne MW, Bansal PK, Kecman V. Neural networks a new approach to model vapor-compression heat pumps. Int J Energy Res 2001; 25:591–599.
[21] Arcaklioglu E. 2004 Performance comparison of C.F.C.s with their substitutes using artificial neural networks. Int J Energy Res ;28: 1113–25.
[22] Islamoglu Y. 2005 Performance prediction for non-adiabatic capillary tube suction-line heat exchanger: an artificial neural network approach. Energy Convers Manage ; 46: 223–32.
[23] Ertunc HM, Hosoz M. 2008 Artificial neural network analysis of a refrigeration system with an evaporative condenser. Appl Therm Eng, in press.
[24] Adnan Sozen, ErolArcaklio glu, Ali Erisen, and M. Ali Akcayol, 2003 Performance prediction of a vapor-compression heat-pump, Elsevier,
[25] Reddy DVR, P. Bhramara, K. Govindarajulu, 2020 A Comparative Study of Multiple Regression and Artificial Neural Network models for a domestic refrigeration system with a hydrocarbon refrigerant mixtures, Materials today proceedings No. 22P4, Feb- 2020, 1545-1553
[26] Che Wan Mohd Noor, RizalmanMamat and Ali Najah Ahmed , 2018, Comparative Study Of Artificial Neural Network and Mathematical Model On Marine Diesel Engine Performance Prediction, International Journal of Innovative Computing, Information and Control, 14(3) 959-969
[27] Abhijit Sarkar, Prasenjit Dey, R N Rai And Subhas Chandra Saha, 2016 A comparative study of multiple regression analysis and back propagation neural network approaches on plain carbon steel in submerged-arc welding, Indian Academy of Sciences, 41 (5) 549–559
[28] Erdi Tosun, KadirAydin , Mehmet Bilgili, 2016, Comparison of linear regression and artificial neural network model of a diesel engine fueled with biodiesel-alcohol mixtures, Alexandria Engineering Journal, 55, 3081–3089
[29] Faezehossadat KHADEMI , Mahmoud AKBARI, Sayed Mohammadmehdi JAMAL, Mehdi NIKOO, 2017, Multiple linear regression, artificial neural network, and fuzzy logic prediction of 28 days compressive strength of concrete, Springer Verlag Berlin Heidelberg, 1-10
[30] MuammerNalbant, HasanGokkaya, Thsan Toktas¸ 2007, Comparison of Regression and Artificial Neural Network Models for Surface Roughness Prediction with the Cutting Parameters in CNC Turning, Hindawi Publishing Corporation Modeling and Simulation in Engineering, 1-14